FAIRLABEL: Correcting Bias in Labels
2023 23RD IEEE INTERNATIONAL CONFERENCE ON DATA MINING WORKSHOPS, ICDMW 2023(2023)
摘要
There are several algorithms for measuring fairness of ML models. A fundamental assumption in these approaches is that the ground truth is fair or unbiased. In real-world datasets, however, the ground truth often contains data that is a result of historical and societal biases and discrimination. Models trained on these datasets will inherit and propagate the biases to the model outputs. We propose FAIRLABEL, an algorithm which detects and corrects biases in labels. The goal of FAIRLABELis to reduce the Disparate Impact (DI) across groups while maintaining high accuracy in predictions. We propose metrics to measure the quality of bias correction and validate FAIRLABEL on synthetic datasets and show that the label correction is correct 86.7% of the time vs. 71.9% for a baseline model. We also apply FAIRLABEL on benchmark datasets such as UCI Adult, German Credit Risk, and Compas datasets and show that the Disparate Impact Ratio increases by as much as 54.2%.
更多查看译文
关键词
bias,fairness,fairlabel,synthetic data,bias correction,bias mitigation
AI 理解论文
溯源树
样例
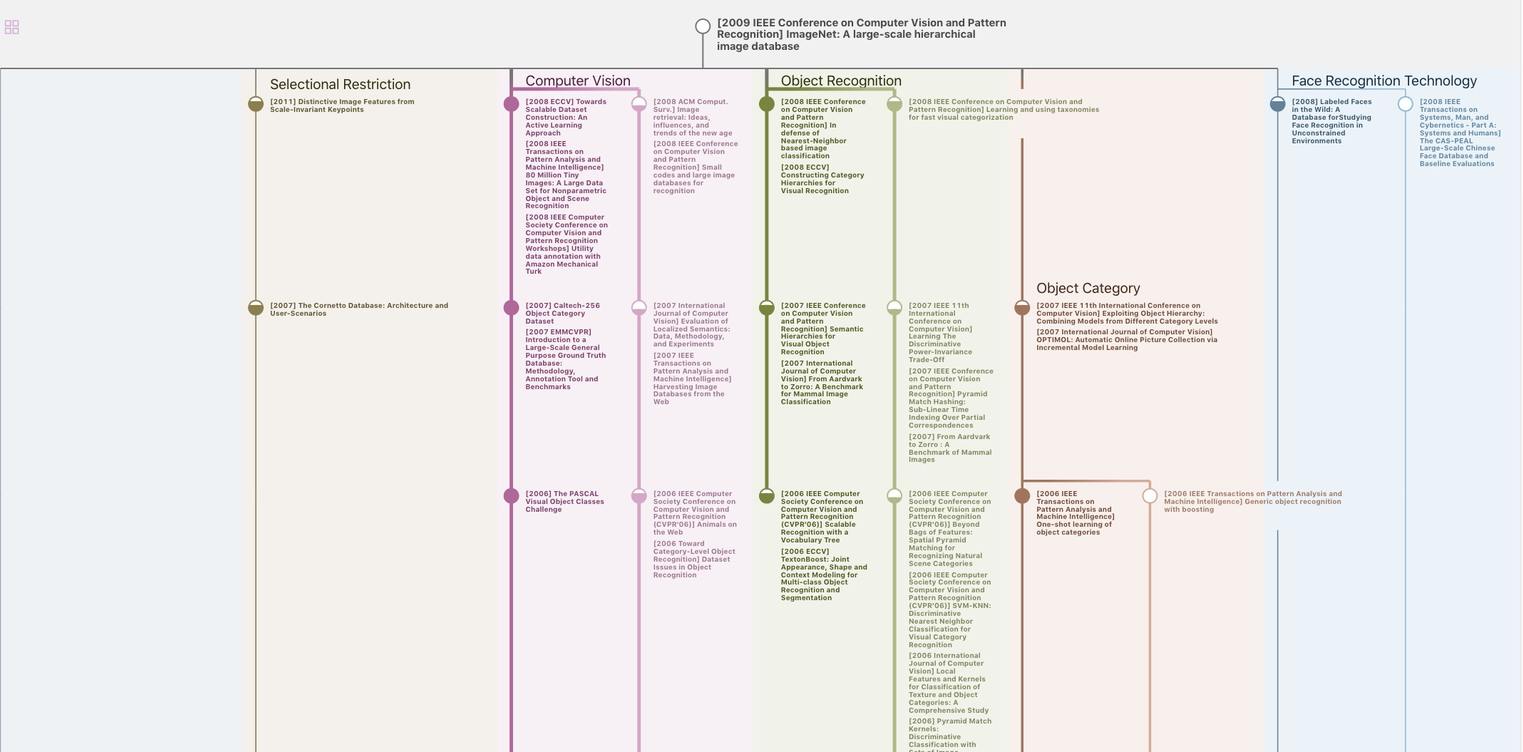
生成溯源树,研究论文发展脉络
Chat Paper
正在生成论文摘要