Data Augmentation for Code Translation with Comparable Corpora and Multiple References.
CoRR(2023)
摘要
One major challenge of translating code between programming languages is that parallel training data is often limited. To overcome this challenge, we present two data augmentation techniques, one that builds comparable corpora (i.e., code pairs with similar functionality), and another that augments existing parallel data with multiple reference translations. Specifically, we build and analyze multiple types of comparable corpora, including programs generated from natural language documentation using a code generation model. Furthermore, to reduce overfitting to a single reference translation, we automatically generate additional translation references for available parallel data and filter the translations by unit tests, which increases variation in target translations. Experiments show that our data augmentation techniques significantly improve CodeT5 for translation between Java, Python, and C++ by an average of 7.5% Computational Accuracy (CA@1), which verifies the correctness of translations by execution. The code is available at https://github.com/Veronicium/CMTrans.
更多查看译文
关键词
code translation,comparable corpora,data augmentation
AI 理解论文
溯源树
样例
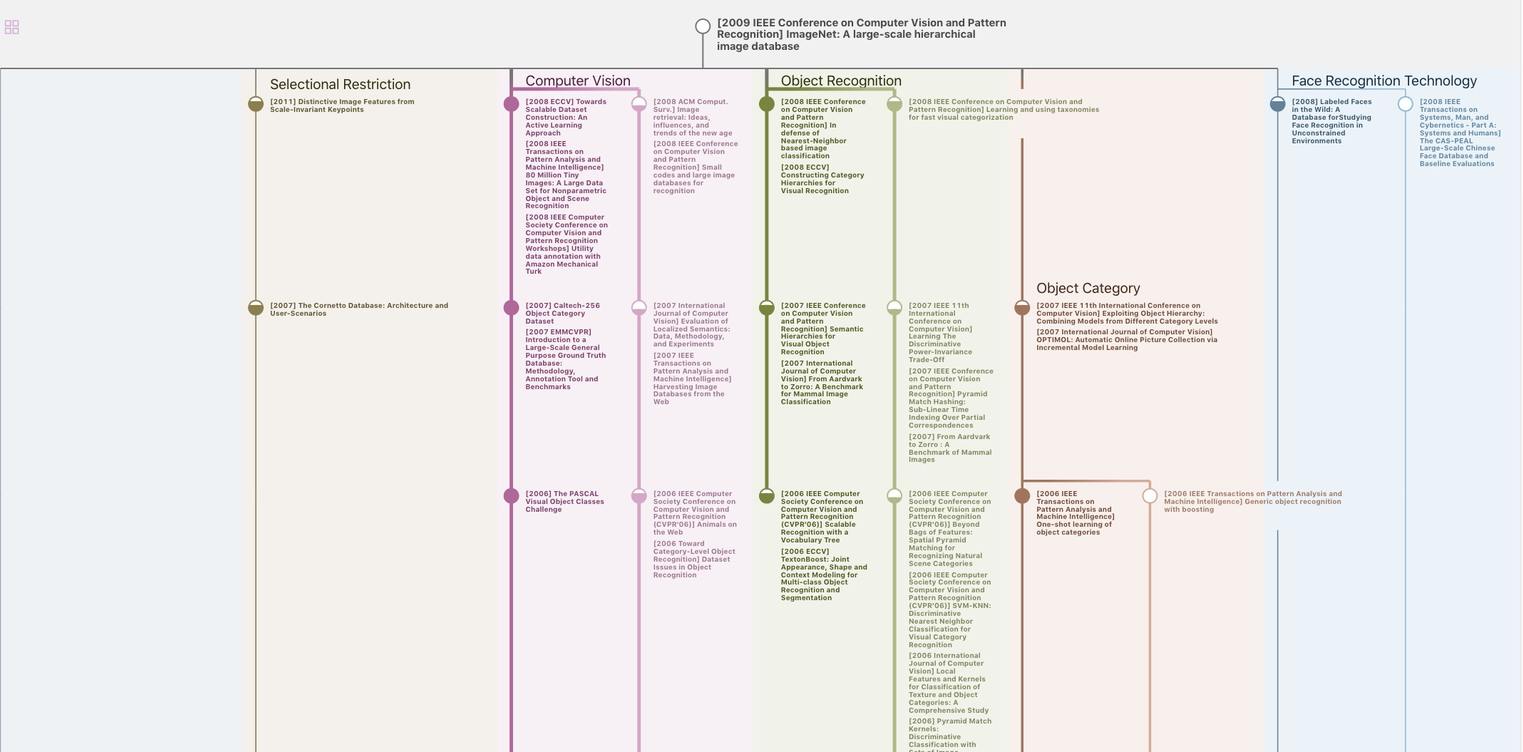
生成溯源树,研究论文发展脉络
Chat Paper
正在生成论文摘要