Random coordinate descent: a simple alternative for optimizing parameterized quantum circuits
arXiv (Cornell University)(2023)
摘要
Variational quantum algorithms rely on the optimization of parameterized quantum circuits in noisy settings. The commonly used back-propagation procedure in classical machine learning is not directly applicable in this setting due to the collapse of quantum states after measurements. Thus, gradient estimations constitute a significant overhead in a gradient-based optimization of such quantum circuits. This paper introduces a random coordinate descent algorithm as a practical and easy-to-implement alternative to the full gradient descent algorithm. This algorithm only requires one partial derivative at each iteration. Motivated by the behavior of measurement noise in the practical optimization of parameterized quantum circuits, this paper presents an optimization problem setting that is amenable to analysis. Under this setting, the random coordinate descent algorithm exhibits the same level of stochastic stability as the full gradient approach, making it as resilient to noise. The complexity of the random coordinate descent method is generally no worse than that of the gradient descent and can be much better for various quantum optimization problems with anisotropic Lipschitz constants. Theoretical analysis and extensive numerical experiments validate our findings.
更多查看译文
关键词
parameterized quantum circuits,random coordinate descent
AI 理解论文
溯源树
样例
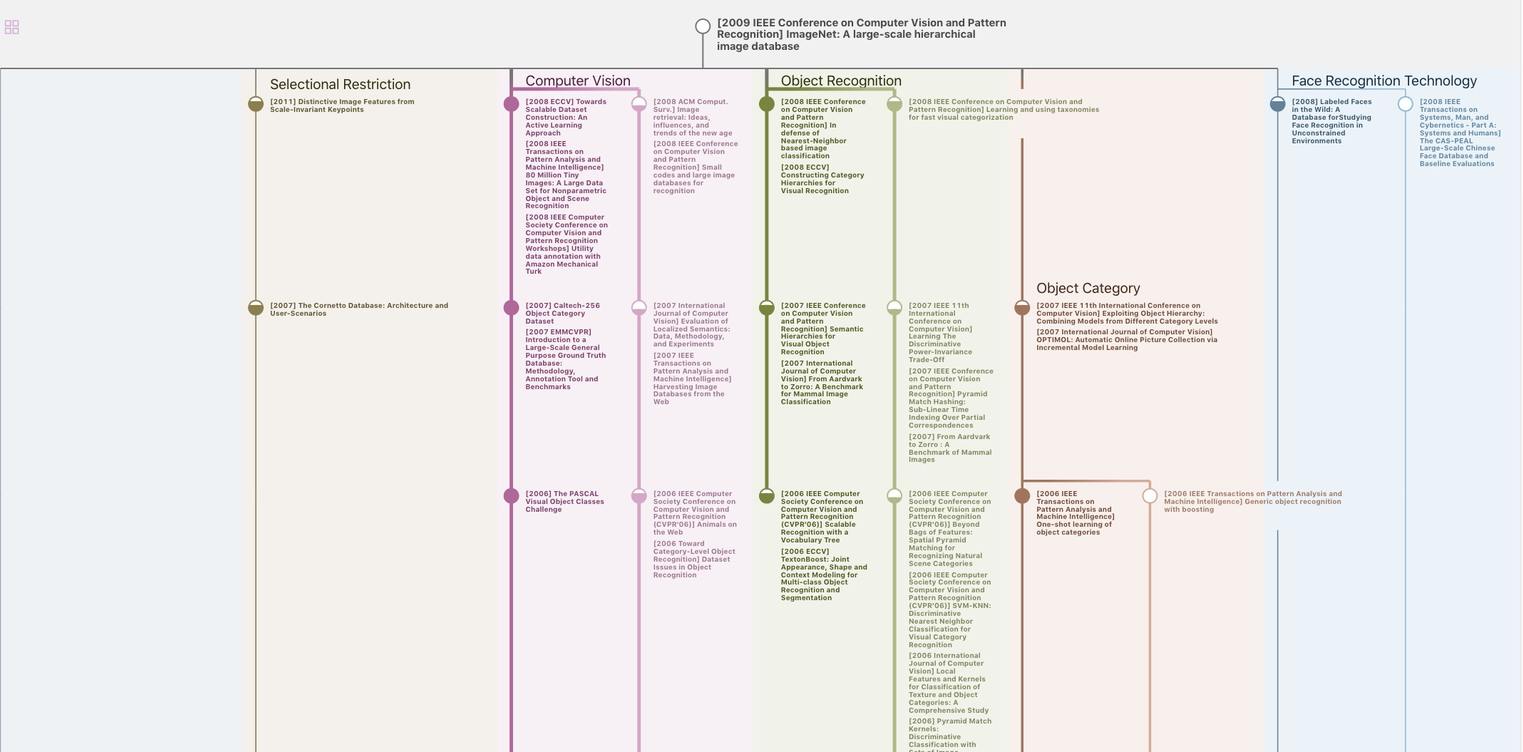
生成溯源树,研究论文发展脉络
Chat Paper
正在生成论文摘要