Esophageal cancer detection framework based on time series information from smear images
EXPERT SYSTEMS WITH APPLICATIONS(2024)
摘要
The gold standard for esophageal cancer diagnosis and treatment is the Thinprep Cytologic Test (TCT) of suspected sections. TCT refers to analyze the features of the lesion areas using tissue regions stained with hematoxylin and eosin. However, pathologists are currently subjected to high workloads and limited experiences. Moreover, automatic esophageal cancer detection methods typically screen the smears by focusing on suspicious lesions identified by cytotechnicians, cell misclassification often results in inaccurate and weak robust diagnoses, smear utilization rate is also reduced. Computer-assisted diagnosis also faces challenges such as information loss, non-quantitative analysis, and nonstandard diagnosing processes. To address these issues, this article proposes a deep learning based detection framework called the Esophageal Time Series Thinprep Cytologic Test (ETS-TCT) to provide a quantitative and robust screening of Whole Slide Imaging (WSI) for esophageal cancer. Our system is divided into three modules: Coarse-fine detection model, quantitative analysis model, and Fusion Long Short Term Memory-Support Vector Machine (FLSTM-SVM) model. These modules are organized to map the pathological information from cell-level to smear-level and offer interpretable predictions to pathologists. For each smear, we design the coarse-fine segmentation model for adaptively locating and classifying target cells, give mathematical descriptions for cell Deoxyribonucleic Acid (DNA) values using the quantitative analysis model, establish an early risk screening model for esophageal cancer using the optimized FLSTM-SVM network. To validate the methodology, we use two clinical datasets containing 580 samples, achieving the accuracy, sensitivity, specificity, and area under ROC (AUC) of 92.0%, 93.4%, 94.4%, and 0.913, respectively. In short, the framework provides robust and accurate diagnosis of esophageal cancer WSIs using the time series features. This screening can assist the pathologists in strengthening the diagnosis by robustly presenting the quantitative intermediate results as well as visual interpretations. Moreover, we clearly present and expatiate the concepts of time series features, highlighting the relationship between these features to physiological states of patients. Our results indicate the possibility of using the framework as a second system to the computer-assisted esophageal cancer screening.
更多查看译文
关键词
Computer-aided screening,Time series classification,Quantitative analysis,Cancer diagnosis,Deep learning
AI 理解论文
溯源树
样例
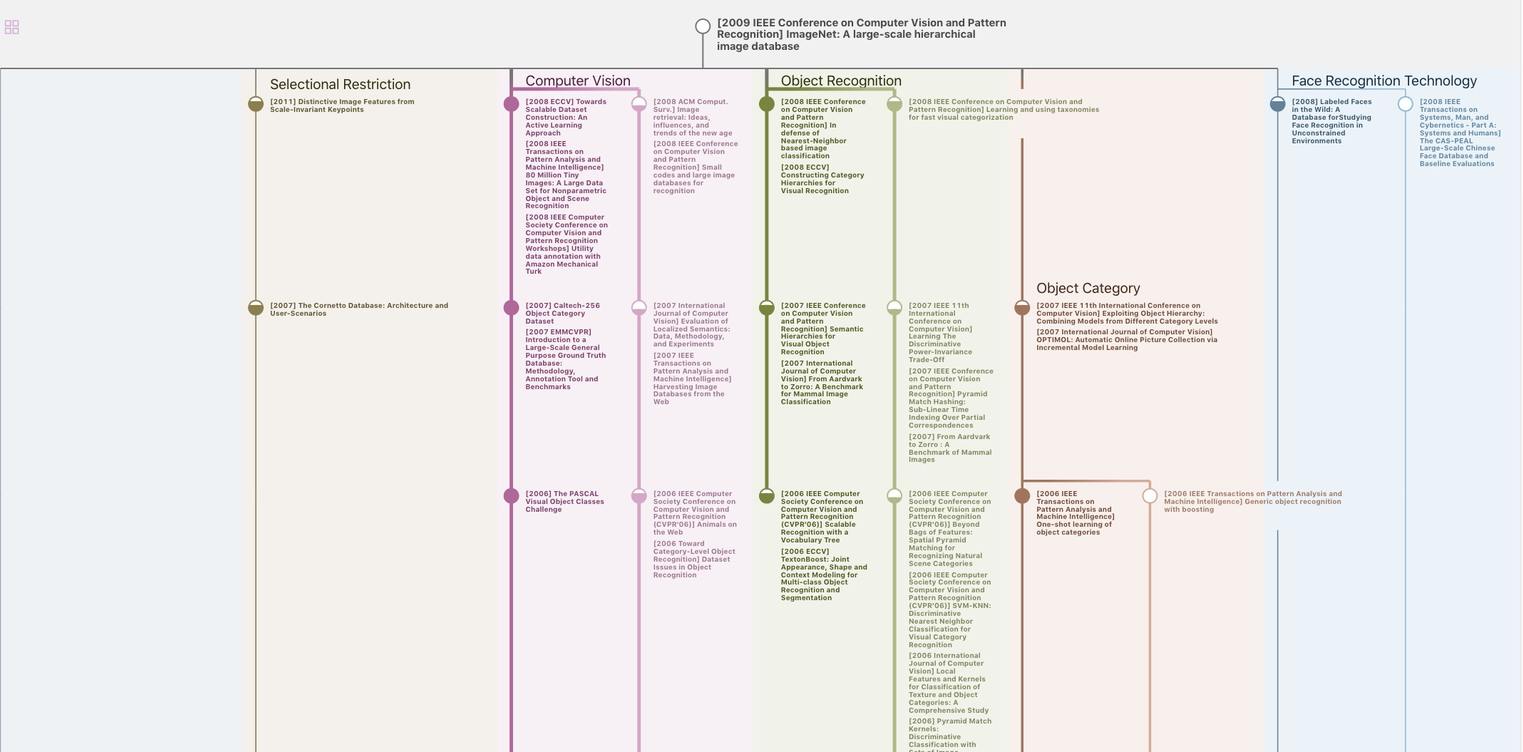
生成溯源树,研究论文发展脉络
Chat Paper
正在生成论文摘要