Admission Control with Resource Efficiency Using Reinforcement Learning in Beyond-5G Networks
2023 IEEE 34TH ANNUAL INTERNATIONAL SYMPOSIUM ON PERSONAL, INDOOR AND MOBILE RADIO COMMUNICATIONS, PIMRC(2023)
摘要
Managing network slices in 5G networks and in communication technologies Beyond-5G (B5G) requires intelligent mechanisms to ensure users' service access and to maximize the utility and efficiency of the network's physical resources. To achieve this, we propose a mechanism based on Reinforcement Learning (RL) for the Admission Control (AC) of User Service Requests (USRs) into network slices through dynamic bandwidth (BW) reallocation. Our approach admits, delays or rejects USRs into service depending on the BW of the slice and the utility this generates for the infrastructure provider (InP). This approach achieves very low USR rejection rates (RRs) with very high resource efficiency, even when peak traffic loads considerably exceed the BW capacity causing resource scarcity scenarios. When compared against a static BW allocation mechanism, our approach achieves RRs that are a fraction (0,33) of those achieved by static (smaller RRs are better), with 33,2x less resource overallocation, significantly achieving a very high resource efficiency.
更多查看译文
关键词
Admission Control,Bandwidth Reallocation,Reinforcement Learning,Network Slicing,B5G
AI 理解论文
溯源树
样例
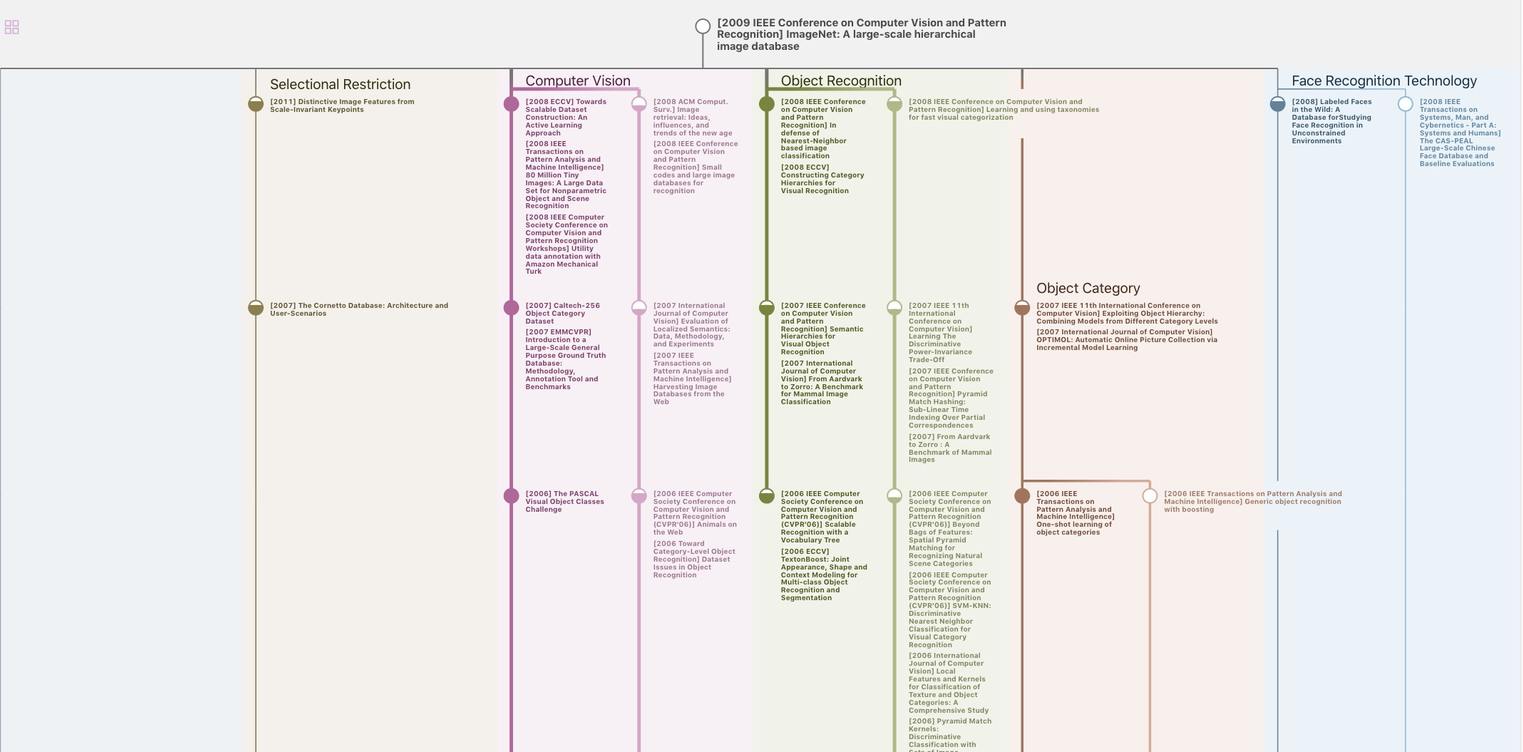
生成溯源树,研究论文发展脉络
Chat Paper
正在生成论文摘要