A Novel Evolutionary Constrained Multi-Objective Optimization Method for Identifying Personalized Drug Targets Combining with Structural Network Control Principles
2023 5th International Conference on Data-driven Optimization of Complex Systems (DOCS)(2023)
摘要
Recently, multi-objective optimization-based structural network control principles (MONCPs) provide a new perspective for identifying personalized drug targets (PDTs), which are envolved with two objectives: minimuming the number of driver nodes (i.e., first objective function) and maximuming the number of prior known drug targets (i.e., second objective function). However, existing methods tend to overlook the knowledge gained from traditional single objective optimization based structural network control principles (SOSNCs, optimizing the first objective function) for solving MONCPs which may affect the accuracy of PDTs idnentification. To improve the performance of MONCPs for identifying PDTs, this paper proposed a novel algorithm called large-scale constrained variables based two stage evolutionary algorithm (LSCV-TSEA) by adapting the prior information of SOSNCs. In the first stage, SOSNCs were utilized in the personalized gene interaction network (PGIN) to explore the information of the first objective function. For the second stage, in the early process the learned information of stage 1 was incorporated into the randomly initialization strategy and during the later evolution process, the search direction of the auxiliary population with optimizing first objective function is modified to the direction of the second objective function, which could help to explore the undeveloped area of PF. By comparing with other algorithms on the three largest cancer omics datasets from The Cancer Genome Atlas database (i.e., breast invasive carcinoma, lung adenocarcinoma and lung squamous cell carcinoma), LSCV-TSEA can more effectively identify PDTs with the higher Area Under the Curve score for predicting clinically annotated combinatorial drugs. Meanwhile, LSCV-TSEA can more efficiently improve the algorithm convergence and diversity compared with other CMOEAs.
更多查看译文
关键词
Structural network control principles,Constraint multi-objective optimization,Personalized drug targets
AI 理解论文
溯源树
样例
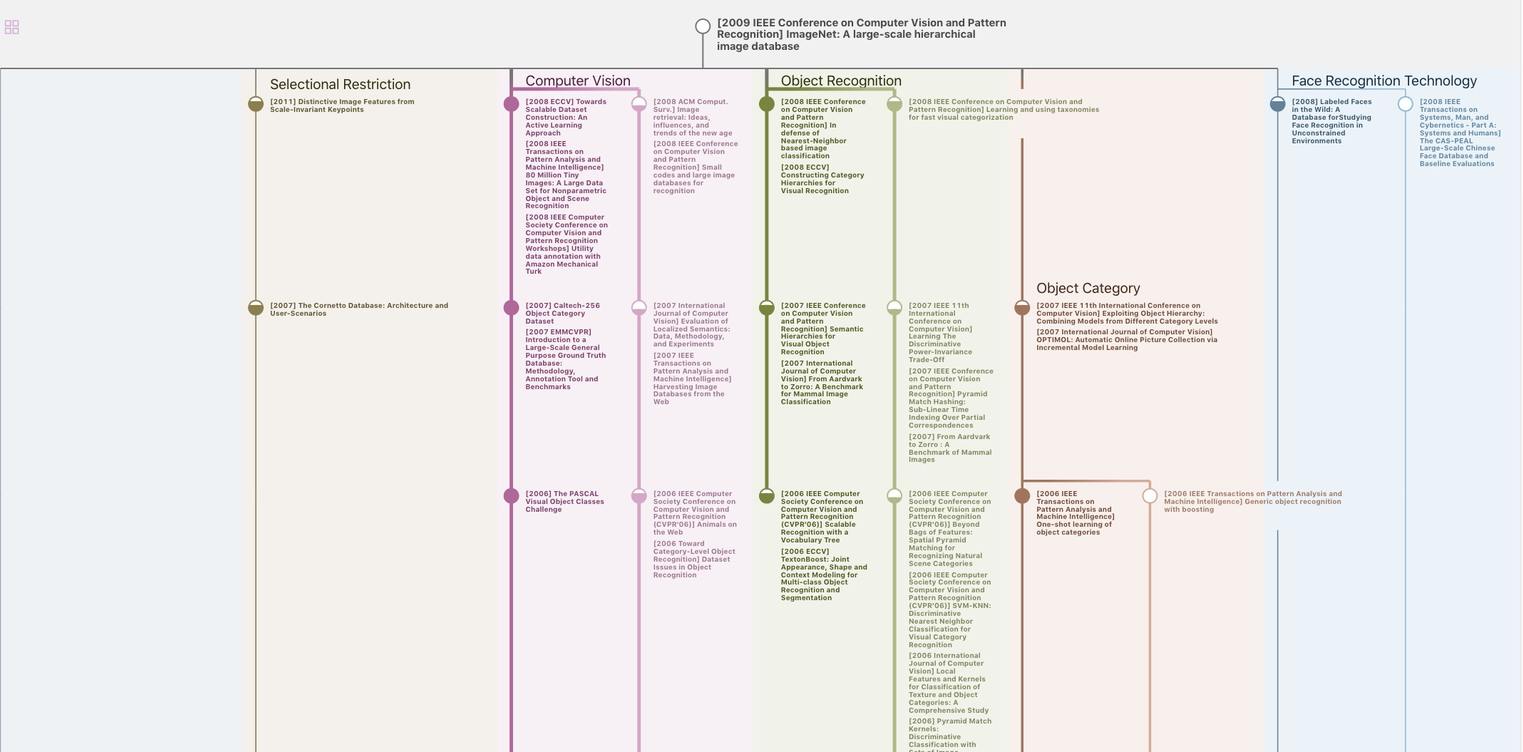
生成溯源树,研究论文发展脉络
Chat Paper
正在生成论文摘要