Convolutional Neural Network Architecture Training Parameters Impact on Multi-Frequency Propagation Channel Model in the VHF and UHF Bands
2023 IEEE AFRICON(2023)
摘要
A path loss estimation model that is both computationally efficient, and precise is required for link budgeting, system performance optimization, base station selection, and coverage analysis. The limitations of empirical and deterministic models, on the other hand, necessitate the incorporation of computational intelligence into the path loss channel models development for multi-frequency band propagation channels. The principle and technique of Deep Neural Network (DNN) were applied in this paper for the modelling and development of a multi-frequency Convolutional Neural Network (CNN)-based path loss estimation model. The CNN architecture employed is a one-dimensional convolution - consisting of a convolution layer, a flattened layer, a dense layer and two fully connected layers. Filter sizes and epochs were varied to examine the effect on accuracy with respect to RMSE, and MSE during training. Results from the CNN model show that there is a significant impact of the training parameters in the development of the CNN model.
更多查看译文
关键词
Multi-frequency,Propagation,CNN model,Deterministic model,Empirical model,Path loss estimation
AI 理解论文
溯源树
样例
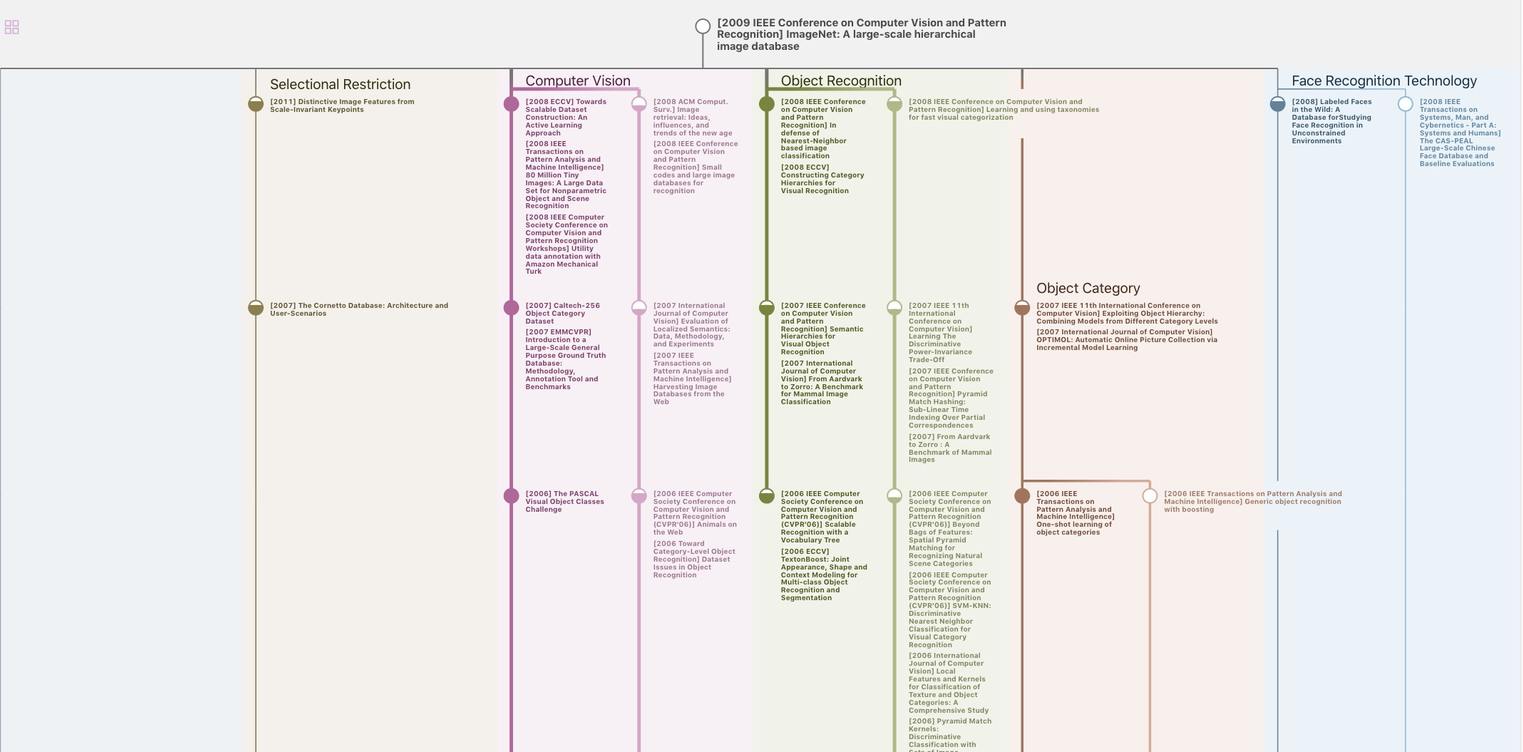
生成溯源树,研究论文发展脉络
Chat Paper
正在生成论文摘要