Temporal enhanced inductive graph knowledge tracing
APPLIED INTELLIGENCE(2023)
摘要
The COVID-19 pandemic has led to a surge in online education, yielding increased attention on knowledge tracing (KT). KT involves predicting a student’s mastery of knowledge based on the student’s learning activity and plays a crucial role for recommending appropriate educational content at the individual level. While several deep learning-based KT models have been proposed, most consider only the interaction sequence of the target student, ignoring the interactions of other students. Furthermore, in practice, all model graphs should be incremented as more student interaction data is obtained, implying that they should be rebuilt to remain up-to-date, which is time-consuming. Moreover, existing graph-based KT models typically rely on predefined knowledge graphs for graph-based learning. Constructing a knowledge graph is labor-intensive, requiring a higher understanding of the domain knowledge. To address these problems, we propose a new inductive graph-based KT (IGKT) framework, which fully utilizes a subgraph extraction approach from a student-exercise bipartite graph, promising scalability and efficiency for practical applicability. The proposed framework consists of three key components: subsequence extraction, subgraph extraction and exericse information addition, and graph-based correctness prediction. For the third component, we test three variants to determine the effectiveness of integrating the timestamp and graph information, thereby creating three models for comparison: IGKT-Basic, IGKT with timestamps (IGKT-TS), and IGKT with graph attention layers that incorporate timestamps as edge features (IGKT-GAT). Extensive experiments conducted on multiple real-world datasets show that the models based on the proposed framework are superior over state-of-the-art models, and IGKT-GAT is the most effective implementation of the proposed framework. We further validate the effectiveness of the IGKT models through an ablation study and multiple in-depth analyses. Graphical abstract
更多查看译文
关键词
Knowledge tracing,Graph-based knowledge tracing,Inductive graph,IGKT
AI 理解论文
溯源树
样例
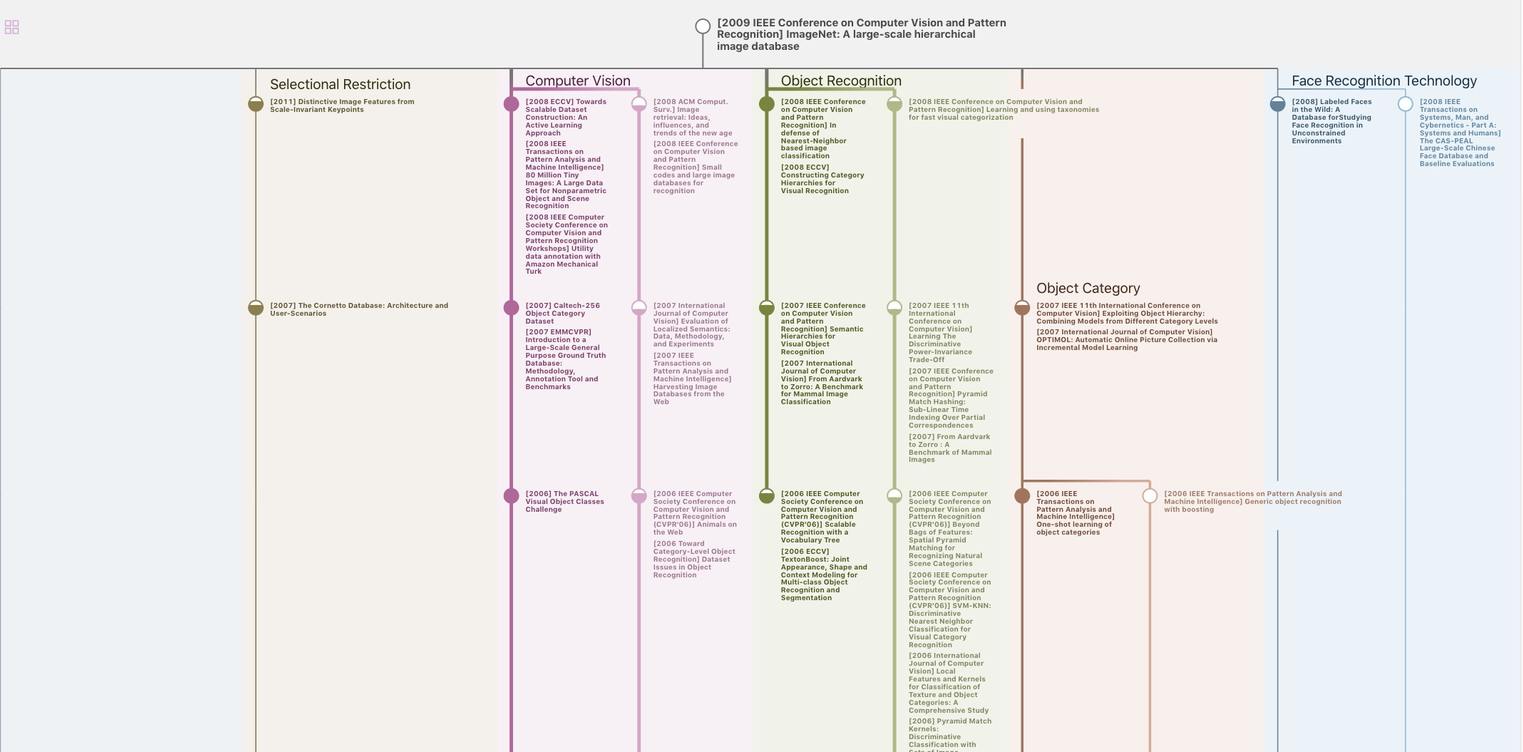
生成溯源树,研究论文发展脉络
Chat Paper
正在生成论文摘要