Refining Diffusion Planner for Reliable Behavior Synthesis by Automatic Detection of Infeasible Plans
NeurIPS(2023)
摘要
Diffusion-based planning has shown promising results in long-horizon,
sparse-reward tasks by training trajectory diffusion models and conditioning
the sampled trajectories using auxiliary guidance functions. However, due to
their nature as generative models, diffusion models are not guaranteed to
generate feasible plans, resulting in failed execution and precluding planners
from being useful in safety-critical applications. In this work, we propose a
novel approach to refine unreliable plans generated by diffusion models by
providing refining guidance to error-prone plans. To this end, we suggest a new
metric named restoration gap for evaluating the quality of individual plans
generated by the diffusion model. A restoration gap is estimated by a gap
predictor which produces restoration gap guidance to refine a diffusion
planner. We additionally present an attribution map regularizer to prevent
adversarial refining guidance that could be generated from the sub-optimal gap
predictor, which enables further refinement of infeasible plans. We demonstrate
the effectiveness of our approach on three different benchmarks in offline
control settings that require long-horizon planning. We also illustrate that
our approach presents explainability by presenting the attribution maps of the
gap predictor and highlighting error-prone transitions, allowing for a deeper
understanding of the generated plans.
更多查看译文
关键词
reliable behavior synthesis
AI 理解论文
溯源树
样例
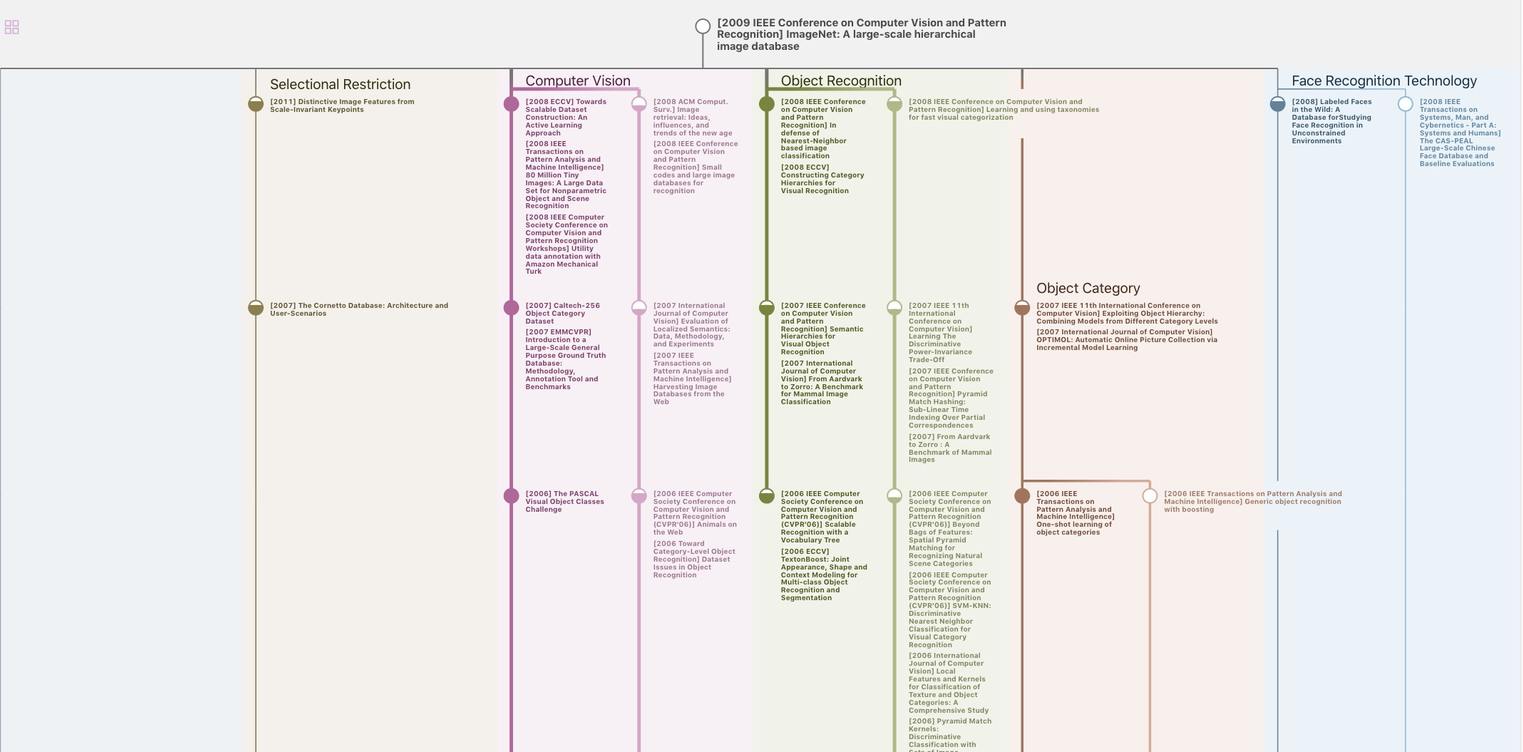
生成溯源树,研究论文发展脉络
Chat Paper
正在生成论文摘要