Efficient Cluster Selection for Personalized Federated Learning: A Multi-Armed Bandit Approach
2023 IEEE Virtual Conference on Communications (VCC)(2023)
摘要
Federated learning (FL) offers a decentralized training approach for machine
learning models, prioritizing data privacy. However, the inherent heterogeneity
in FL networks, arising from variations in data distribution, size, and device
capabilities, poses challenges in user federation. Recognizing this,
Personalized Federated Learning (PFL) emphasizes tailoring learning processes
to individual data profiles. In this paper, we address the complexity of
clustering users in PFL, especially in dynamic networks, by introducing a
dynamic Upper Confidence Bound (dUCB) algorithm inspired by the multi-armed
bandit (MAB) approach. The dUCB algorithm ensures that new users can
effectively find the best cluster for their data distribution by balancing
exploration and exploitation. The performance of our algorithm is evaluated in
various cases, showing its effectiveness in handling dynamic federated learning
scenarios.
更多查看译文
关键词
Federated Learning,Cluster Selection,Dynamic Upper Confidence Bound
AI 理解论文
溯源树
样例
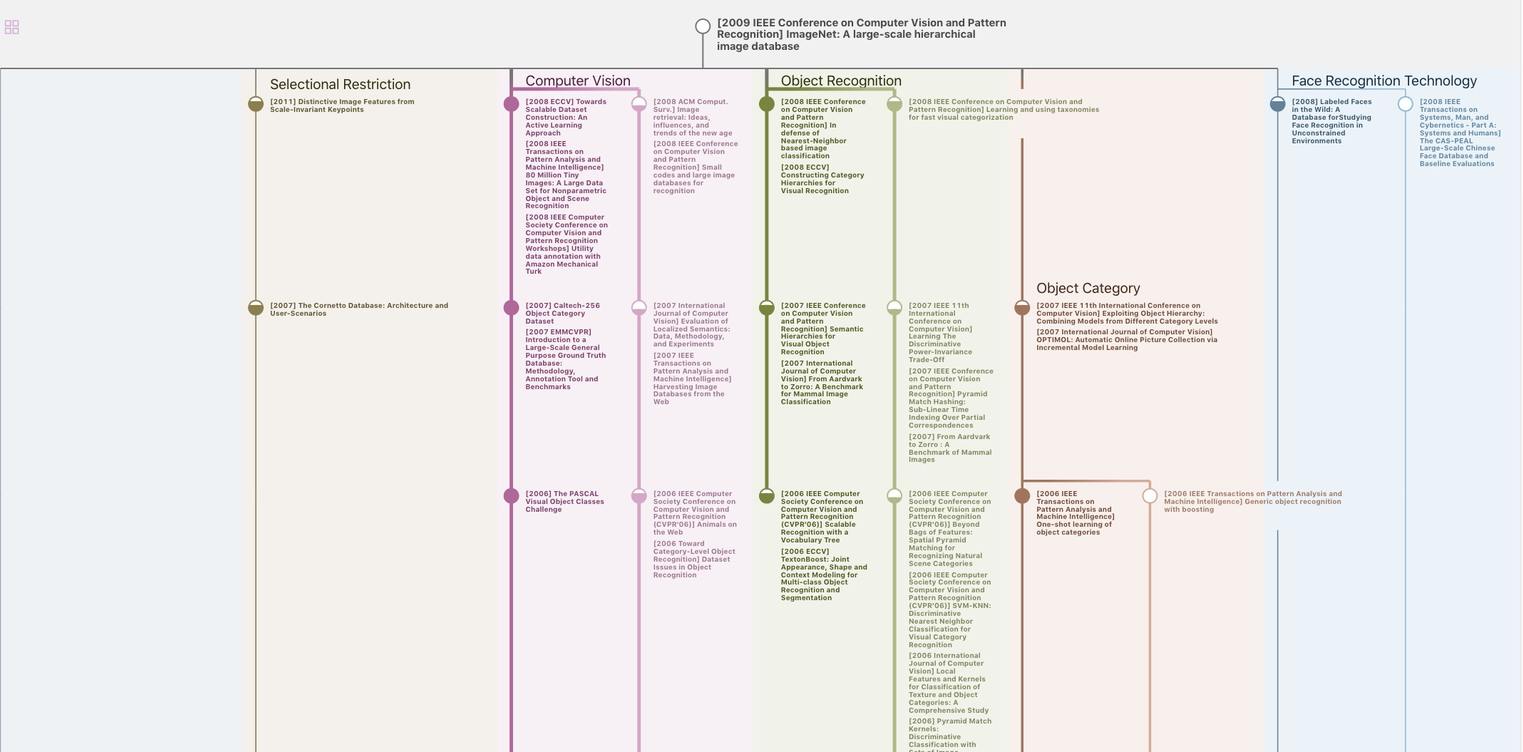
生成溯源树,研究论文发展脉络
Chat Paper
正在生成论文摘要