Explainable few-shot learning for online anomaly detection in ultrasonic metal welding with varying configurations
JOURNAL OF MANUFACTURING PROCESSES(2023)
摘要
Ultrasonic metal welding (UMW) is a solid-state joining technology with widespread industrial applications. While UMW has numerous important advantages compared to traditional fusion-based welding methods, its performance can be substantially influenced by process anomalies such as tool degradation and material surface contamination, which are commonly encountered in industrial-scale productions. Recently, online monitoring has demonstrated excellent anomaly detection capabilities. However, the existing monitoring algorithms require a large amount of labeled data and lack the generalizability or adaptability to new process configurations (i.e., domains). This paper develops a meta-learning-based explainable few-shot learning (XFSL) framework that enables highly data-efficient adaptation of online monitoring algorithms to new process configurations with excellent explainability. We consider two distinct types of problems including tool condition monitoring and workpiece surface condition classification with varying UMW configurations. Using experimental data, we demonstrate that the proposed XFSL method achieves high classification performance in previously unseen target domains and significantly outperforms baseline methods. Furthermore, XFSL is able to evaluate the importance of each feature, thus revealing key features, feature types, and signal frequencies. It is shown that explainability-based feature selection can effectively eliminate unimportant information from monitoring signals while maintaining and even improving prediction performance. The proposed XFSL method is extensible to other manufacturing applications and holds significant potential for advancing the generalizability, adaptability, and agility of decision-making algorithms in modern manufacturing.
更多查看译文
关键词
Few-shot learning,Meta-learning,Domain adaptation,Anomaly detection,Ultrasonic metal welding,Quality control,Explainable machine learning
AI 理解论文
溯源树
样例
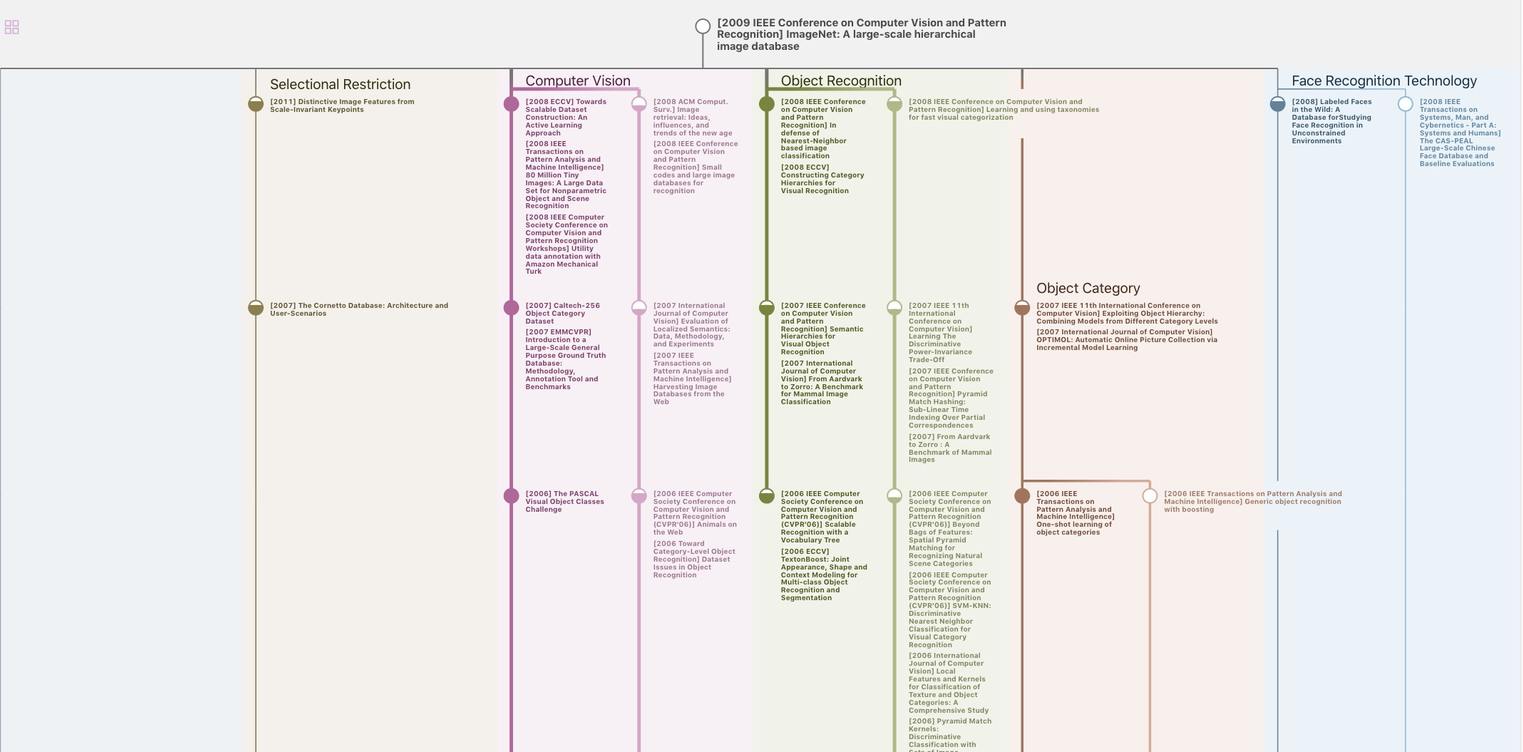
生成溯源树,研究论文发展脉络
Chat Paper
正在生成论文摘要