Leveraging deep learning techniques for ship pipeline valve leak monitoring
OCEAN ENGINEERING(2023)
摘要
The monitoring of ship pipeline valve leaks holds immense significance as it serves to enhance ship safety, mitigate energy and material losses, and protect the health and sustainability of the marine environment. In comparison to traditional expert-based fault diagnosis methods, this study presents a deep learning-based fault feature extraction approach for end-to-end valve leak fault diagnosis. To address the challenge of incomplete leak fault description by a single sensor, a Multi-Channel Multi-Scale Convolutional Neural Network (MCMS-CNN) model is established. Diverging from existing methods, the proposed MCMS-CNN model automatically extracts fault features from grayscale images obtained from two sensors and performs feature-level fusion for fault classification, thereby mitigating the impact of data redundancy and noise and enhancing fault recognition accuracy. The effectiveness of the proposed approach is verified through valve leak experiments, and comparisons are conducted with different models and information fusion methods. The results demonstrate that the proposed MCMS-CNN model exhibits advantages in terms of accuracy and robustness, confirming its practicality in ship pipeline valve leak fault diagnosis.
更多查看译文
关键词
Ship pipeline valve leakage,Fault diagnosis,Signal-to-image mapping,Multi-channel multi-scale convolutional neural,network
AI 理解论文
溯源树
样例
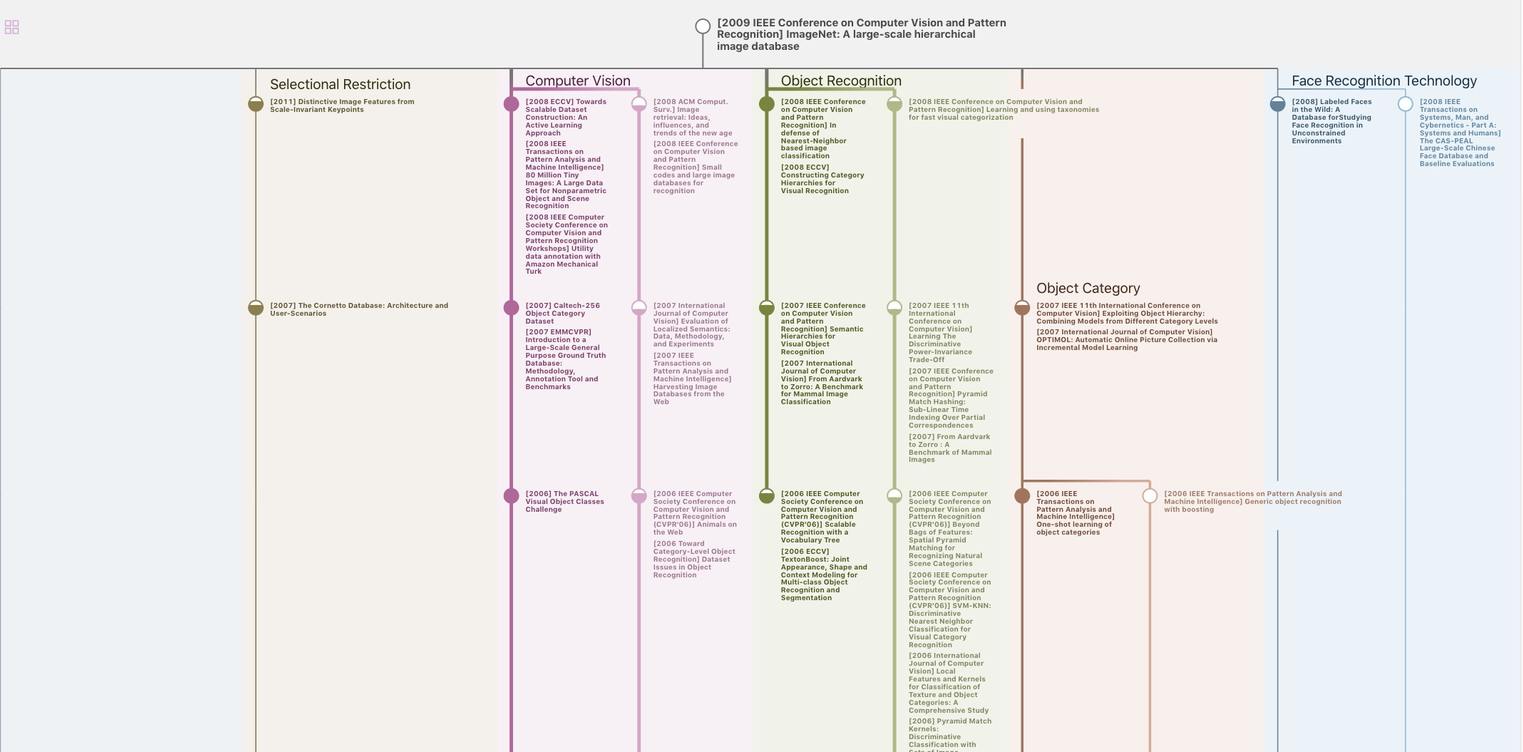
生成溯源树,研究论文发展脉络
Chat Paper
正在生成论文摘要