Machine Learning Refinement of In Situ Images Acquired by Low Electron Dose LC-TEM
MICROSCOPY AND MICROANALYSIS(2024)
摘要
We have studied a machine learning (ML) technique for refining images acquired during in situ observation using liquid-cell transmission electron microscopy. Our model is constructed using a U-Net architecture and a ResNet encoder. For training our ML model, we prepared an original image dataset that contained pairs of images of samples acquired with and without a solution present. The former images were used as noisy images, and the latter images were used as corresponding ground truth images. The number of pairs of image sets was 1,204, and the image sets included images acquired at several different magnifications and electron doses. The trained model converted a noisy image into a clear image. The time necessary for the conversion was on the order of 10 ms, and we applied the model to in situ observations using the software Gatan DigitalMicrograph (DM). Even if a nanoparticle was not visible in a view window in the DM software because of the low electron dose, it was visible in a successive refined image generated by our ML model.
更多查看译文
关键词
in situ observation,liquid cell,machine learning,transmission electron microscopy
AI 理解论文
溯源树
样例
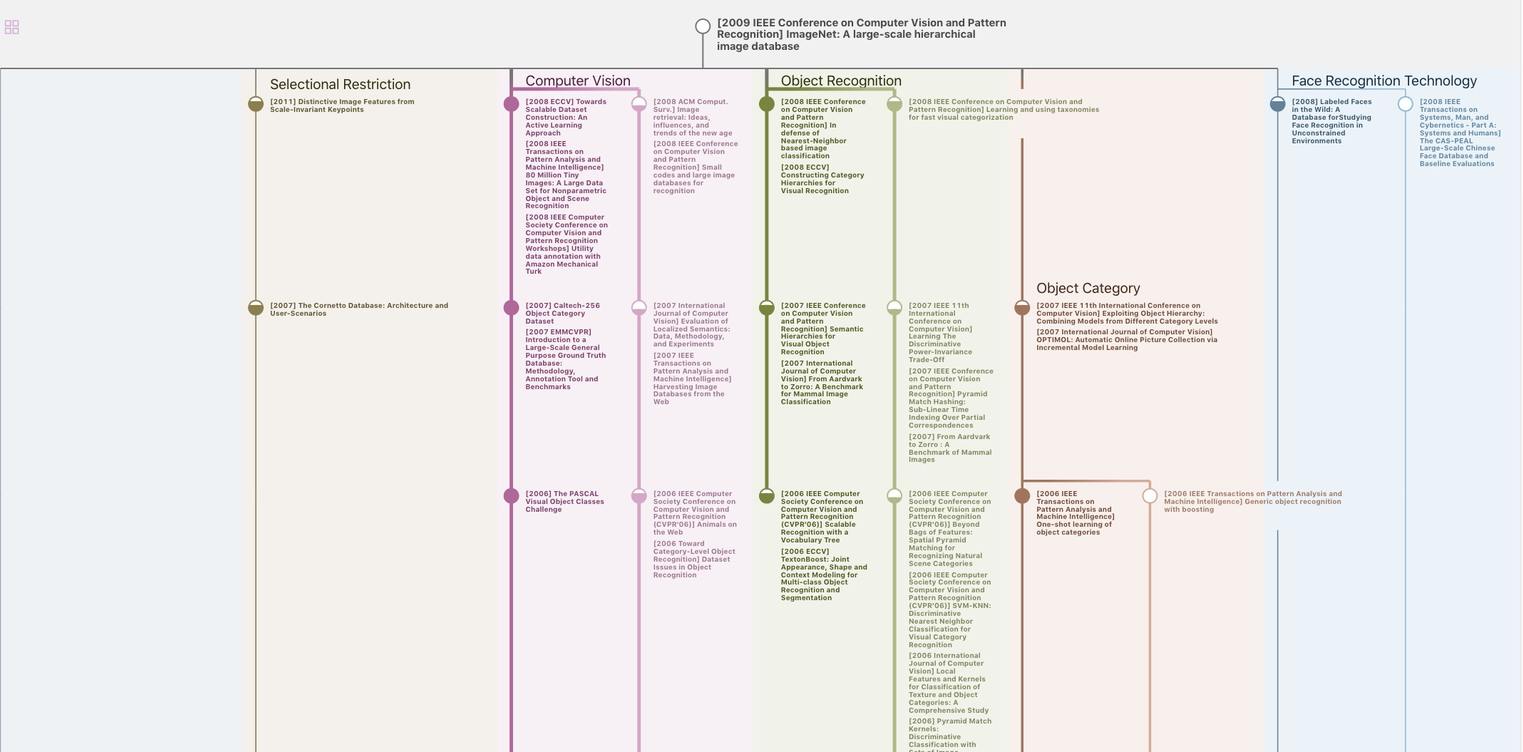
生成溯源树,研究论文发展脉络
Chat Paper
正在生成论文摘要