PolyThrottle: Energy-efficient Neural Network Inference on Edge Devices
CoRR(2023)
摘要
As neural networks (NN) are deployed across diverse sectors, their energy demand correspondingly grows. While several prior works have focused on reducing energy consumption during training, the continuous operation of ML-powered systems leads to significant energy use during inference. This paper investigates how the configuration of on-device hardware-elements such as GPU, memory, and CPU frequency, often neglected in prior studies, affects energy consumption for NN inference with regular fine-tuning. We propose PolyThrottle, a solution that optimizes configurations across individual hardware components using Constrained Bayesian Optimization in an energy-conserving manner. Our empirical evaluation uncovers novel facets of the energy-performance equilibrium showing that we can save up to 36 percent of energy for popular models. We also validate that PolyThrottle can quickly converge towards near-optimal settings while satisfying application constraints.
更多查看译文
关键词
edge devices,neural network,energy-efficient
AI 理解论文
溯源树
样例
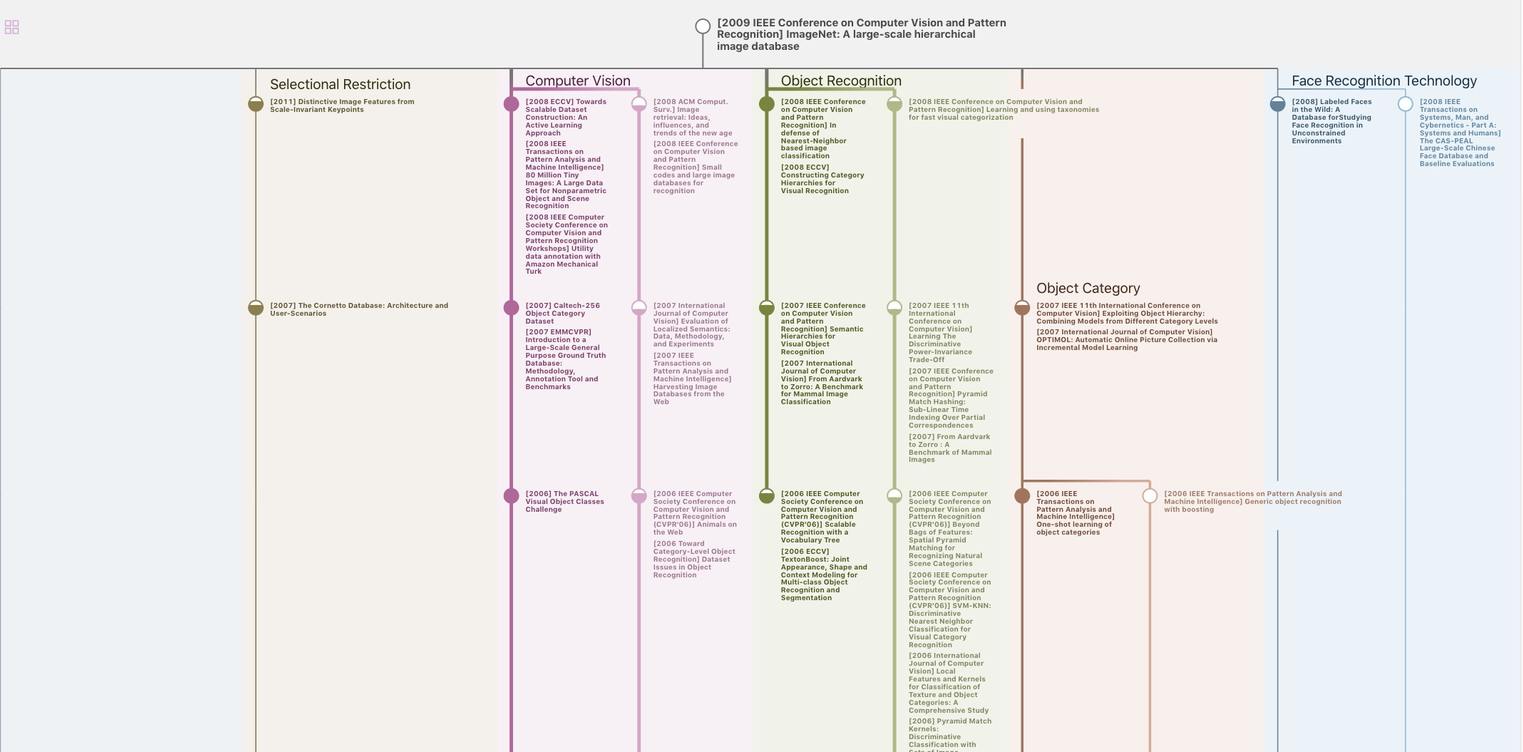
生成溯源树,研究论文发展脉络
Chat Paper
正在生成论文摘要