Meta-Learning Strategies through Value Maximization in Neural Networks
CoRR(2023)
摘要
Biological and artificial learning agents face numerous choices about how to learn, ranging from hyperparameter selection to aspects of task distributions like curricula. Understanding how to make these meta-learning choices could offer normative accounts of cognitive control functions in biological learners and improve engineered systems. Yet optimal strategies remain challenging to compute in modern deep networks due to the complexity of optimizing through the entire learning process. Here we theoretically investigate optimal strategies in a tractable setting. We present a learning effort framework capable of efficiently optimizing control signals on a fully normative objective: discounted cumulative performance throughout learning. We obtain computational tractability by using average dynamical equations for gradient descent, available for simple neural network architectures. Our framework accommodates a range of meta-learning and automatic curriculum learning methods in a unified normative setting. We apply this framework to investigate the effect of approximations in common meta-learning algorithms; infer aspects of optimal curricula; and compute optimal neuronal resource allocation in a continual learning setting. Across settings, we find that control effort is most beneficial when applied to easier aspects of a task early in learning; followed by sustained effort on harder aspects. Overall, the learning effort framework provides a tractable theoretical test bed to study normative benefits of interventions in a variety of learning systems, as well as a formal account of optimal cognitive control strategies over learning trajectories posited by established theories in cognitive neuroscience.
更多查看译文
关键词
value maximization,neural networks,strategies,meta-learning
AI 理解论文
溯源树
样例
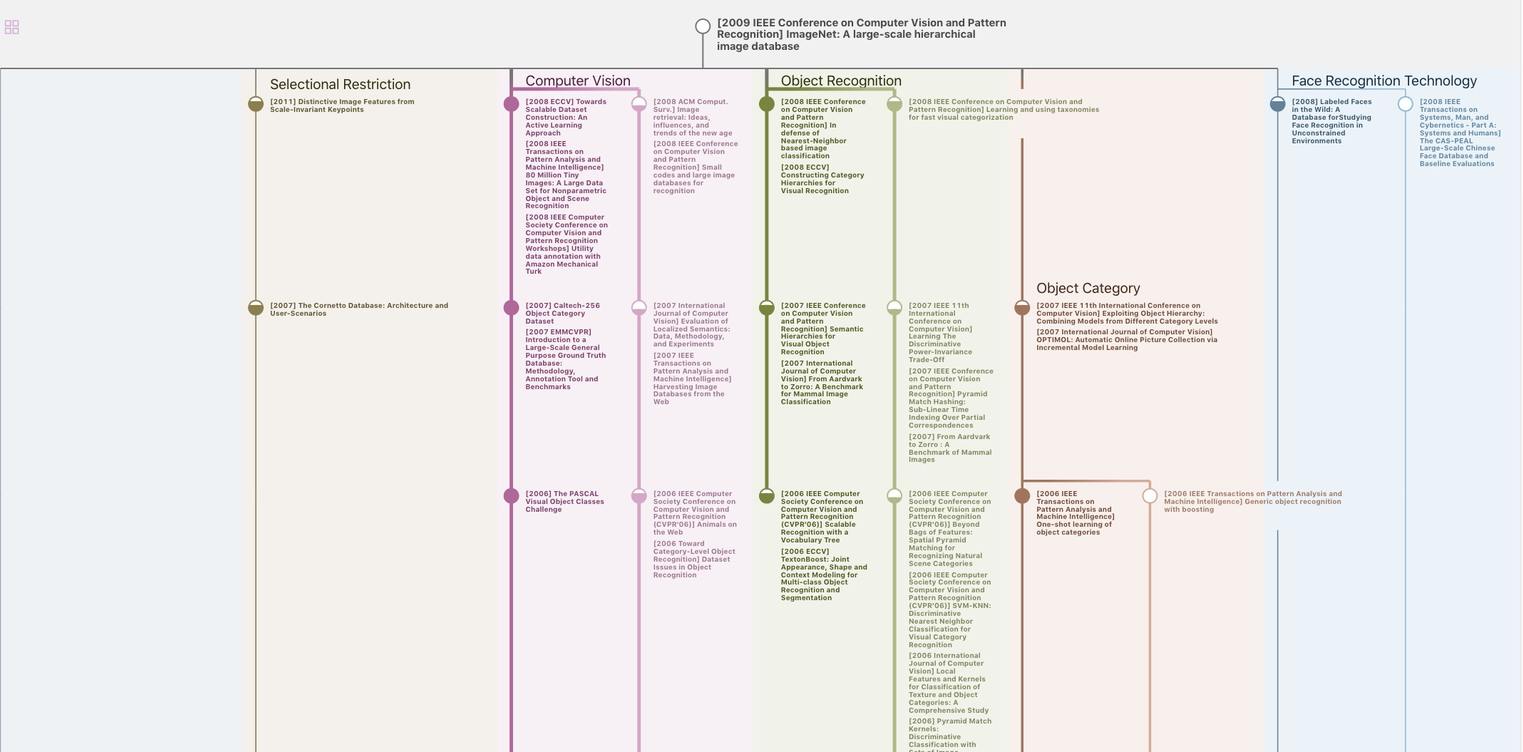
生成溯源树,研究论文发展脉络
Chat Paper
正在生成论文摘要