Spatial-temporal graph neural network traffic prediction based load balancing with reinforcement learning in cellular networks
INFORMATION FUSION(2024)
摘要
Balancing network traffic among base stations poses a primary challenge for mobile operators because of the escalating demand for enhanced data speeds in large-scale 5G radio applications. Within cellular networks, traffic flow prediction constitutes a pivotal issue in numerous applications, such as resource allocation, load balancing, and network slicing. In this paper, traffic prediction based load balancing framework with reinforcement learning is proposed to optimize neighbor cell relational parameters that can better balance traffic within a defined geographical cluster. Spatial-temporal-event cross attention graph convolution neural network (STECA-GCN) is put forward to predict the precise traffic flow. The model takes event dimension features into account, while also incorporating direct cross-fusion among diverse features. Concurrently, we have developed a strategy based on deep reinforcement learning to facilitate dynamic load balancing decisions. Simulation results show that our proposed load balancing framework can improve overall system performance. In particular, the combination of loading rate and energy efficiency can achieve a 12% improvement. The load balancing of the base station can better deal with social emergencies.
更多查看译文
关键词
Cellular networks,Load balancing,Traffic prediction,Graph neural network,Reinforcement learning
AI 理解论文
溯源树
样例
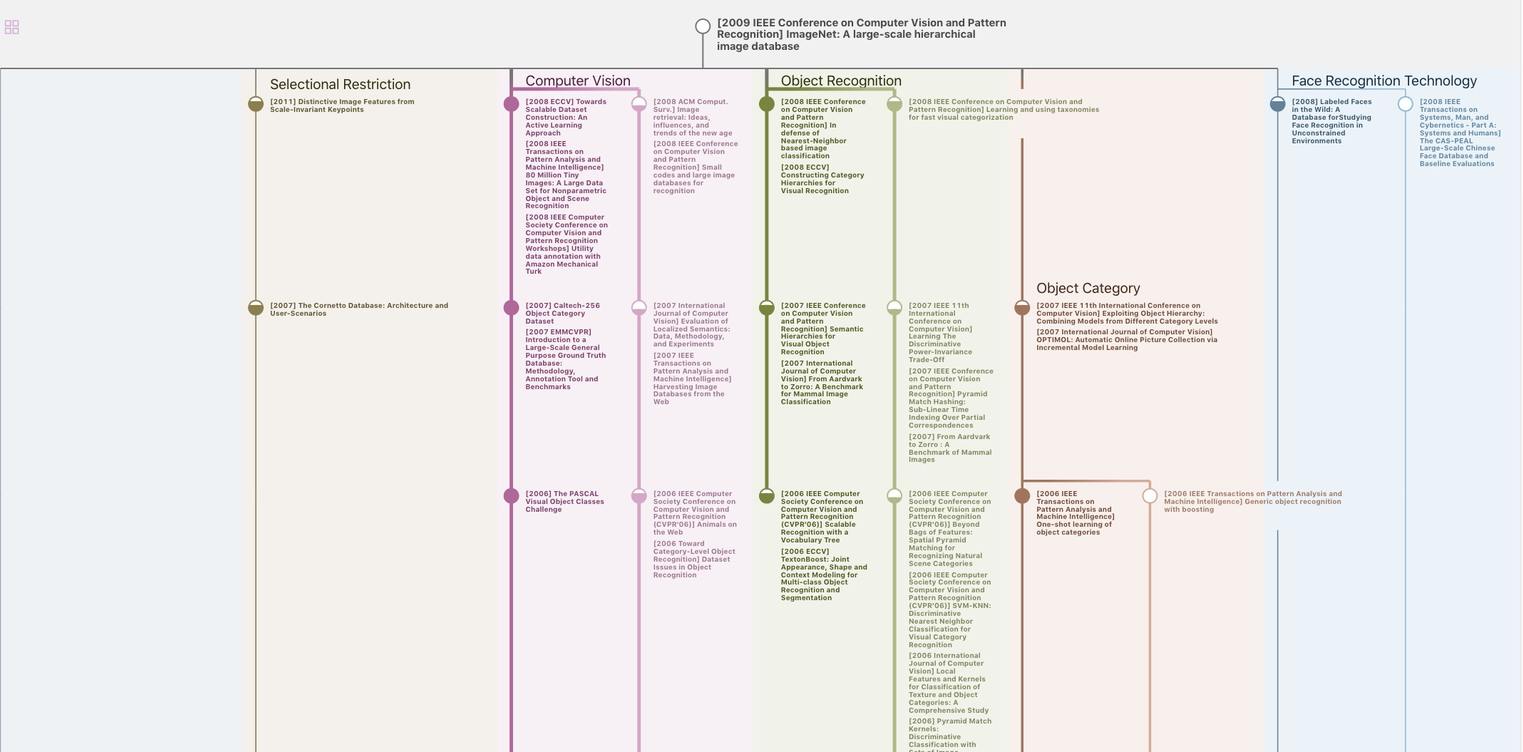
生成溯源树,研究论文发展脉络
Chat Paper
正在生成论文摘要