Online unsupervised optimization framework for machine performance assessment based on distance metric learning
MECHANICAL SYSTEMS AND SIGNAL PROCESSING(2024)
摘要
Machine performance assessment is of great significance to machine health management, whose major tasks are incipient fault detection in a normal stage and constructing a health index (HI) to track the degradation status. However, most previous assessment methods need the rich domain knowledge or labeled historical data and are not adaptive to time-varying working conditions. To address these challenges, a novel two-stage online unsupervised optimization framework for machine performance assessment is proposed without relying on any historical abnormal and faulty data. In the first stage, high-dimensional features are extracted from the raw monitoring signals and then projected into the low-dimensional space for feature selection. A distance metric learning-based clustering model considering separability is proposed to simultaneously detect the incipient fault initial time and search the most effective features, which can separate the normal and degradation stages more distinctly. In the second stage, once the incipient fault is identified, a composite HI is generated based on Mahalanobis distance between the normal features and current degradation features. An adaptive information-theoretic metric learning algorithm is developed to iteratively optimize and update the HI for online degradation assessment, whose objective function is formulated based on LogDet divergence, and the monotonicity and robustness properties. Finally, the effectiveness of the proposed method is illustrated through applications to two bearing datasets.
更多查看译文
关键词
Unsupervised,Degradation assessment,Incipient fault detection,Health index construction,Distance metric learning
AI 理解论文
溯源树
样例
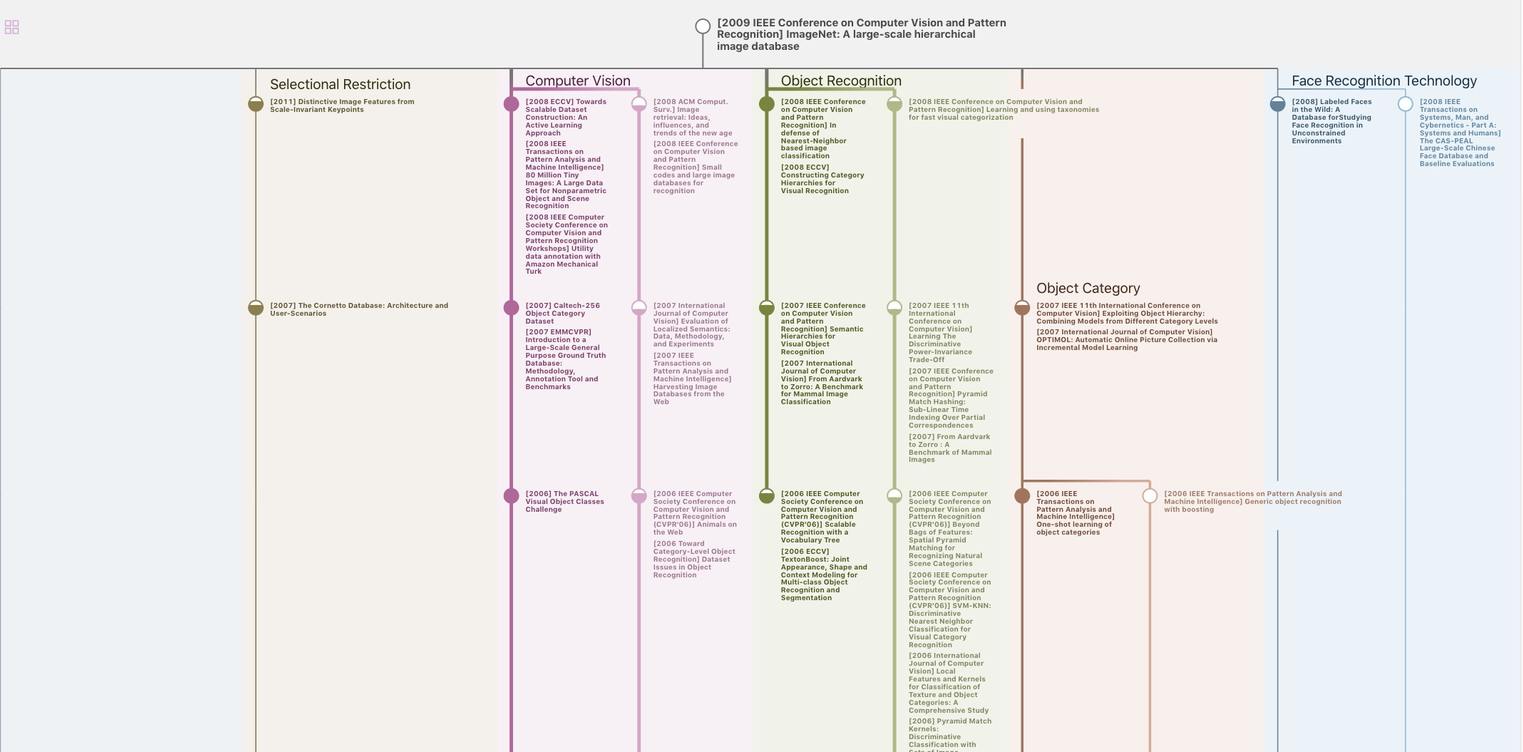
生成溯源树,研究论文发展脉络
Chat Paper
正在生成论文摘要