Bayesian inference of relative fitness on high-throughput pooled competition assays
PLOS COMPUTATIONAL BIOLOGY(2024)
摘要
The tracking of lineage frequencies via DNA barcode sequencing enables the quantification of microbial fitness. However, experimental noise coming from biotic and abiotic sources complicates the computation of a reliable inference. We present a Bayesian pipeline to infer relative microbial fitness from high-throughput lineage tracking assays. Our model accounts for multiple sources of noise and propagates uncertainties throughout all parameters in a systematic way. Furthermore, using modern variational inference methods based on automatic differentiation, we are able to scale the inference to a large number of unique barcodes. We extend this core model to analyze multi-environment assays, replicate experiments, and barcodes linked to genotypes. On simulations, our method recovers known parameters within posterior credible intervals. This work provides a generalizable Bayesian framework to analyze lineage tracking experiments. The accompanying open-source software library enables the adoption of principled statistical methods in experimental evolution. In this study, we present a novel Bayesian pipeline for analyzing DNA barcode tracking sequencing data, addressing the challenge of accurately quantifying competitive microbial fitness in the presence of experimental noise. Our method uniquely contributes to understanding microbial evolutionary dynamics by enabling reliable inference of the relative fitness of diverse microbial strains from high-throughput lineage tracking assays. Our approach is distinct in its ability to systematically account for and propagate uncertainties from various noise sources throughout all inferred parameters. Furthermore, the error-propagation quality of our Bayesian method allows us to extend the inference pipeline to common dataset structures, such as jointly analyzing multiple experimental replicates or accounting for multiple unique barcodes mapping the equivalent genotypes. This comprehensive treatment of uncertainties is crucial in experimental settings where noise can significantly influence the results. Furthermore, we have optimized our pipeline for scalability, allowing it to handle large numbers of unique barcodes effectively. This scalability is essential for analyzing complex datasets typical in microbial fitness studies.
更多查看译文
关键词
competition assays,relative fitness,bayesian inference,high-throughput
AI 理解论文
溯源树
样例
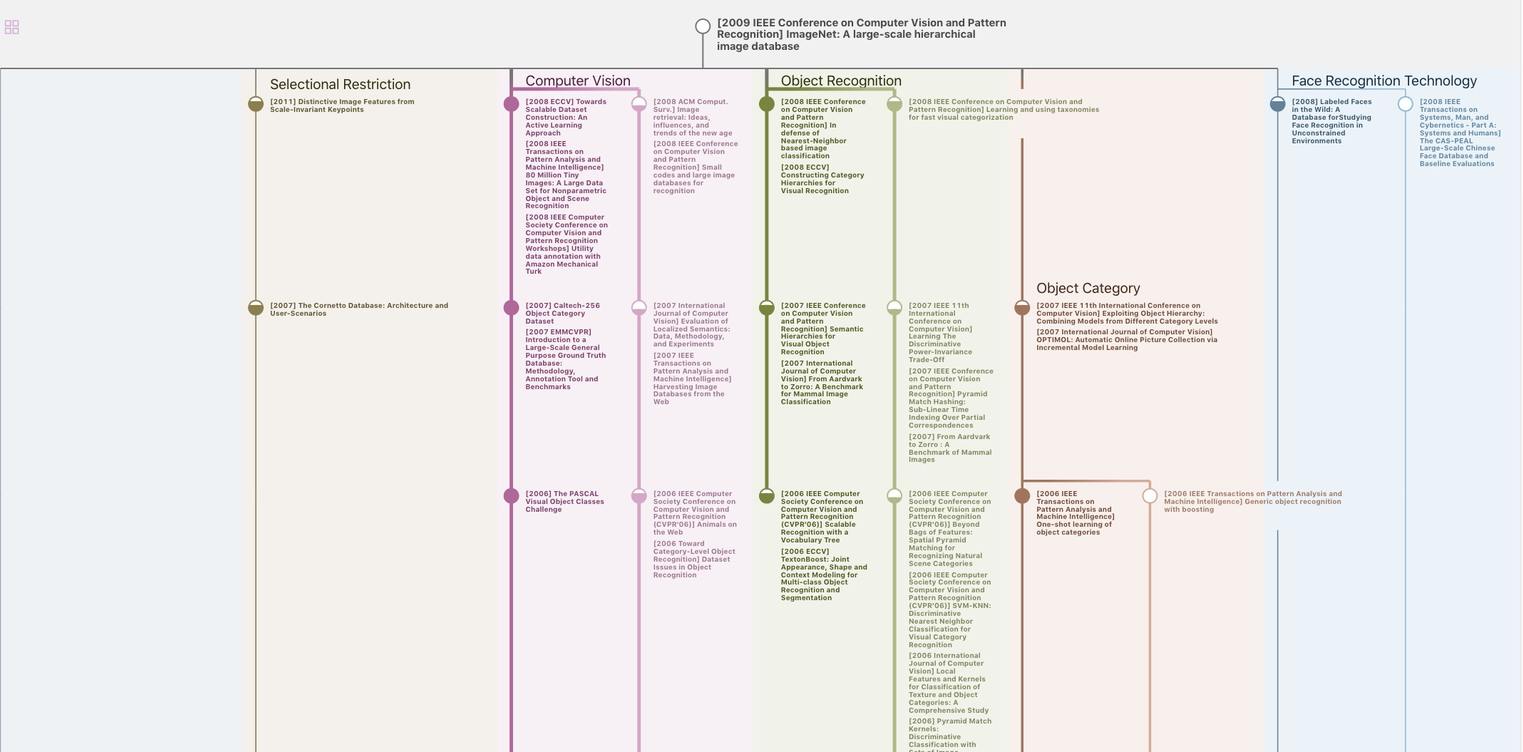
生成溯源树,研究论文发展脉络
Chat Paper
正在生成论文摘要