Predicted Improvement Aggregation Adjustment Search for Parallel Multi-Objective Bayesian Optimization*
2023 International Conference on Communications, Computing, Cybersecurity, and Informatics (CCCI)(2023)
摘要
Expensive multi-objective black-box optimization problems often only allow limited functional evaluations because of time and cost considerations. However, traditional evolutionary algorithms usually require many sample evaluations, making it challenging to solve the above expensive optimization problems. Multi-objective Bayesian optimization algorithms can efficiently solve expensive multi-objective black-box problems by using a sequential search to define infill criteria. Still, conventional serial computing ways cannot fully utilize abundant computer resources. To address the above issues. This paper proposes a predicted improvement aggregation adjustment criterion matched to parallel computing for expensive multi-objective optimization problems in the Bayesian optimization framework. The proposed method employs a predicted improvement aggregation adjustment-based acquisition function to efficiently select batch inputs for evaluation to quickly find high-quality Pareto-set solutions. The predicted improvement aggregation adjustment criterion achieves aggregation and adjustment by integrating the Tchebycheff aggregation function and distance influence function in the design space. Experiment results on several benchmark tests and the vehicle crashworthiness design problem show that the proposed method consistently outperforms compared the state-of-the-art algorithms.
更多查看译文
关键词
Kriging model,predicted improvement aggregation adjustment,Bayesian optimization,parallel computing
AI 理解论文
溯源树
样例
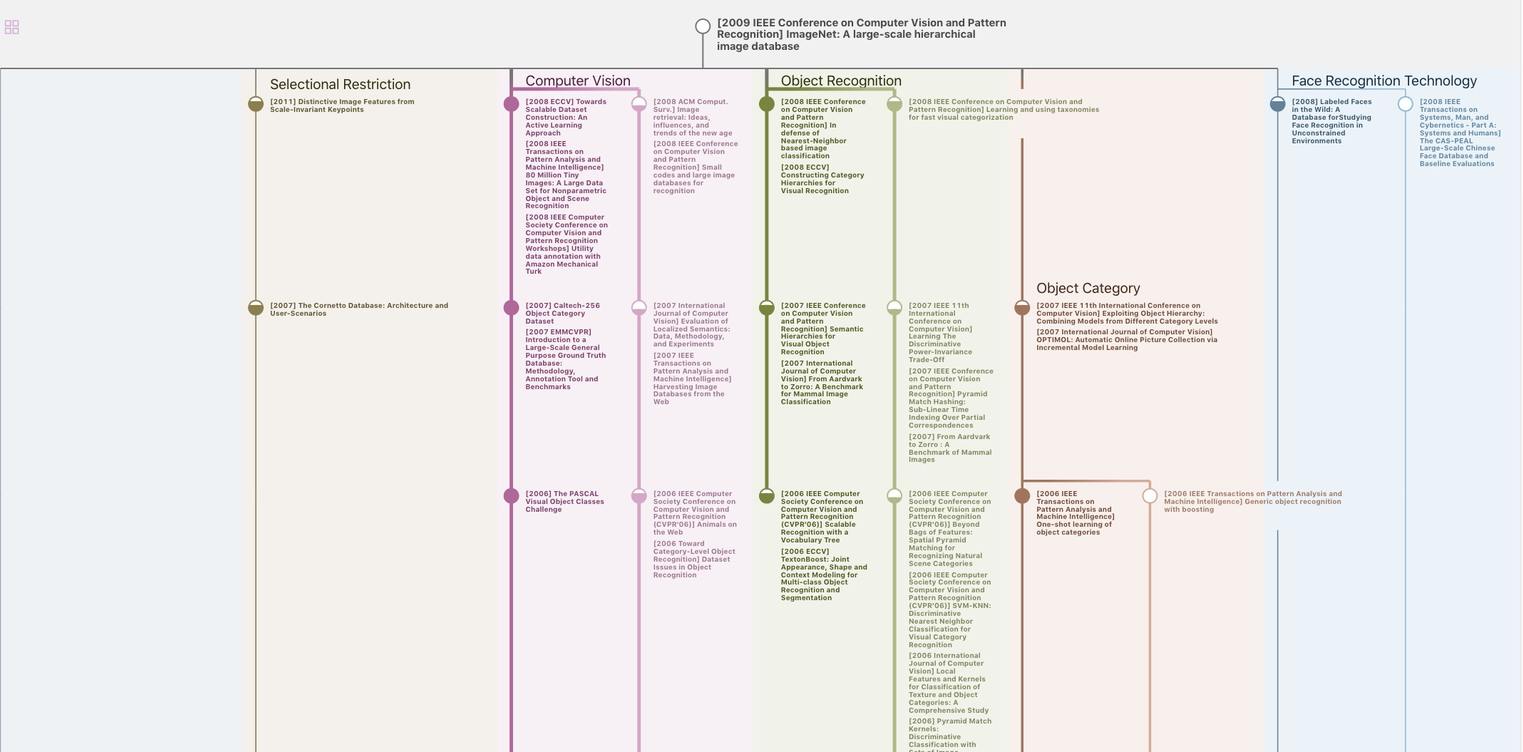
生成溯源树,研究论文发展脉络
Chat Paper
正在生成论文摘要