Using machine learning to improve anaphylaxis case identification in medical claims data
JAMIA open(2023)
摘要
Objective Anaphylaxis is a severe life-threatening allergic reaction, and its accurate identification in healthcare databases can harness the potential of "Big Data" for healthcare or public health purposes.Methods This study used claims data obtained between October 1, 2015 and February 28, 2019 from the CMS database to examine the utility of machine learning in identifying incident anaphylaxis cases. We created a feature selection pipeline to identify critical features between different datasets. Then a variety of unsupervised and supervised methods were used (eg, Sammon mapping and eXtreme Gradient Boosting) to train models on datasets of differing data quality, which reflects the varying availability and potential rarity of ground truth data in medical databases.Results Resulting machine learning model accuracies ranged between 47.7% and 94.4% when tested on ground truth data. Finally, we found new features to help experts enhance existing case-finding algorithms.Discussion Developing precise algorithms to detect medical outcomes in claims can be a laborious and expensive process, particularly for conditions presented and coded diversely. We found it beneficial to filter out highly potent codes used for data curation to identify underlying patterns and features. To improve rule-based algorithms where necessary, researchers could use model explainers to determine noteworthy features, which could then be shared with experts and included in the algorithm.Conclusion Our work suggests machine learning models can perform at similar levels as a previously published expert case-finding algorithm, while also having the potential to improve performance or streamline algorithm construction processes by identifying new relevant features for algorithm construction. Electronic health records and medical claims data are a potential treasure trove for identifying the new underlying content and confirming the existing knowledge base. However, whenever researchers introduce screening criteria in the data curation process, they will also introduce bias if they are not careful. Therefore, it is crucial to consider what information can go into machine learning models. In this work, we show how we used feature elimination and feature selection to replicate the success of human expert-defined anaphylaxis identification models. We then used common and essential features between minimally curated and expert-defined datasets to create a new machine-learning model that can beat the human expert-defined algorithms. This process can be repeated and automated to iteratively develop better models and features, which can help healthcare practitioners design more successful case-defining algorithms.
更多查看译文
关键词
anaphylaxis case identification,medical claims data,machine learning
AI 理解论文
溯源树
样例
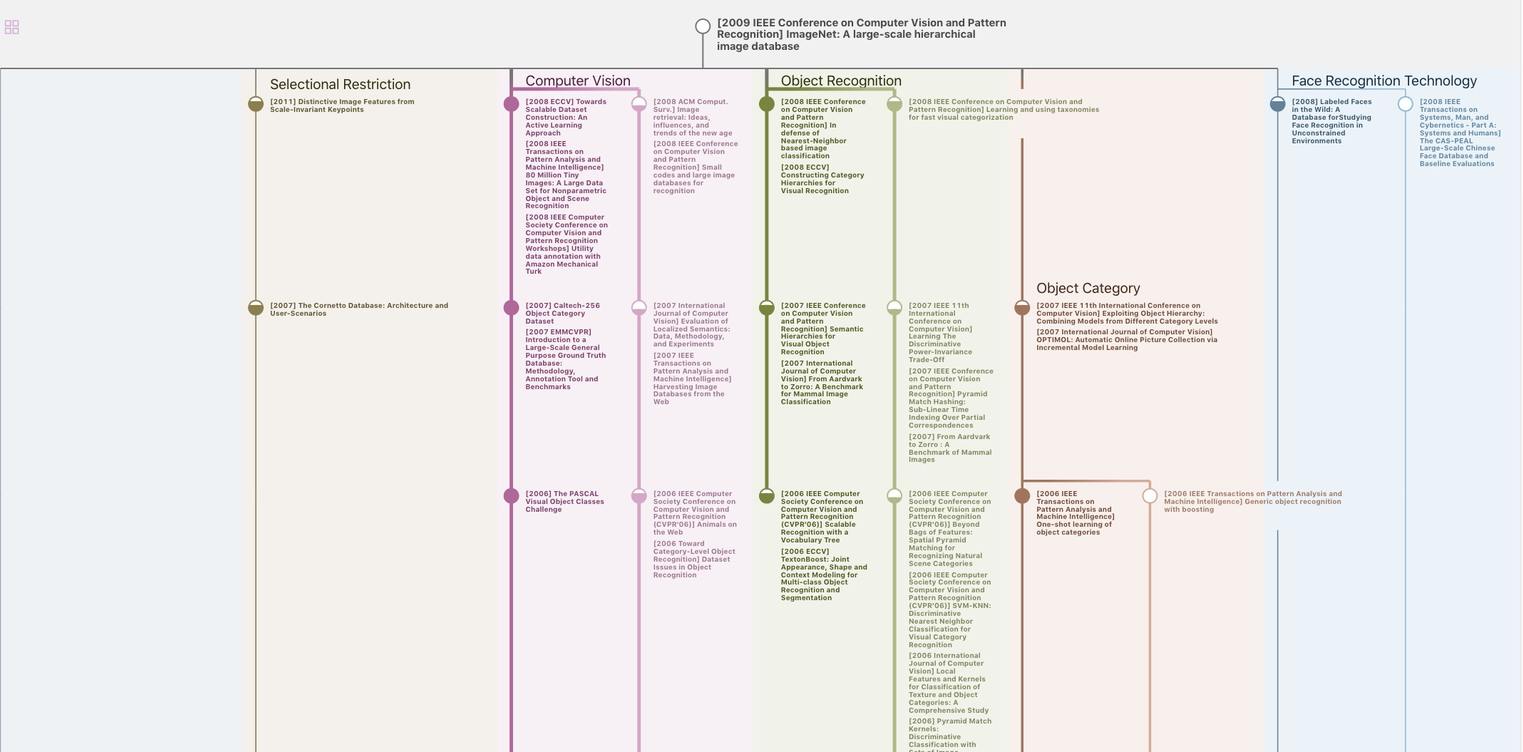
生成溯源树,研究论文发展脉络
Chat Paper
正在生成论文摘要