Bayesian Optimization with Hidden Constraints via Latent Decision Models
CoRR(2023)
摘要
Bayesian optimization (BO) has emerged as a potent tool for addressing intricate decision-making challenges, especially in public policy domains such as police districting. However, its broader application in public policymaking is hindered by the complexity of defining feasible regions and the high-dimensionality of decisions. This paper introduces the Hidden-Constrained Latent Space Bayesian Optimization (HC-LSBO), a novel BO method integrated with a latent decision model. This approach leverages a variational autoencoder to learn the distribution of feasible decisions, enabling a two-way mapping between the original decision space and a lower-dimensional latent space. By doing so, HC-LSBO captures the nuances of hidden constraints inherent in public policymaking, allowing for optimization in the latent space while evaluating objectives in the original space. We validate our method through numerical experiments on both synthetic and real data sets, with a specific focus on large-scale police districting problems in Atlanta, Georgia. Our results reveal that HC-LSBO offers notable improvements in performance and efficiency compared to the baselines.
更多查看译文
关键词
bayesian optimization,hidden constraints,models
AI 理解论文
溯源树
样例
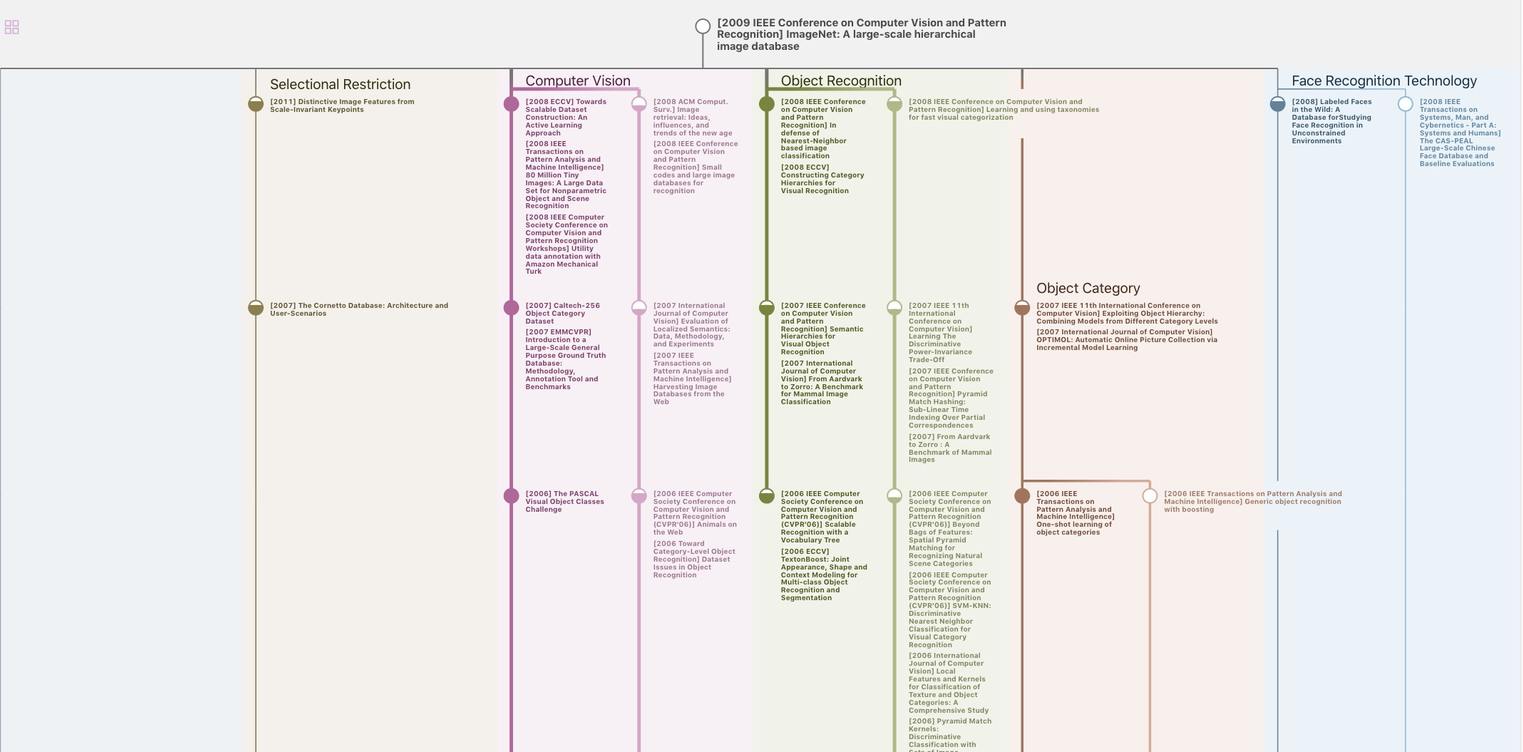
生成溯源树,研究论文发展脉络
Chat Paper
正在生成论文摘要