Patch-to-Sample Reasoning for Cervical Cancer Screening of Whole Slide Image
IEEE transactions on artificial intelligence(2023)
摘要
Deep learning has been instrumental in improving the accuracy of cervical cancer screening using whole-slide images (WSIs) in recent years. Due to the complexity of the computer-aided screening task, the pipeline typically involves detecting “abnormal” cervical cells, and runs classification at the patch and sample levels, respectively. While the patch-level classification for normal or abnormal cells cannot be perfect, the errors may accumulate across individual patches and make the subsequent sample-level classification even more difficult. To address these issues, we propose a Patch-to-Sample (P2S) reasoning method to screen the cervical abnormality in this paper. We first improve the patch-level classifier by the
hard patch mining
strategy, such that the classifier is not only more accurate but also more powerful to represent suspicious cells in local patches. Then, we propose
score embedding
and
token pooling
to a transformer network, which aggregates multiple patches and derives the diagnosis result at the sample level. Experiments show that our P2S method can more effectively utilize the key patches in individual samples, and thus outperforms existing methods.
更多查看译文
关键词
Computer-Aided diagnosis,hard patch mining,score embedding,token pooling
AI 理解论文
溯源树
样例
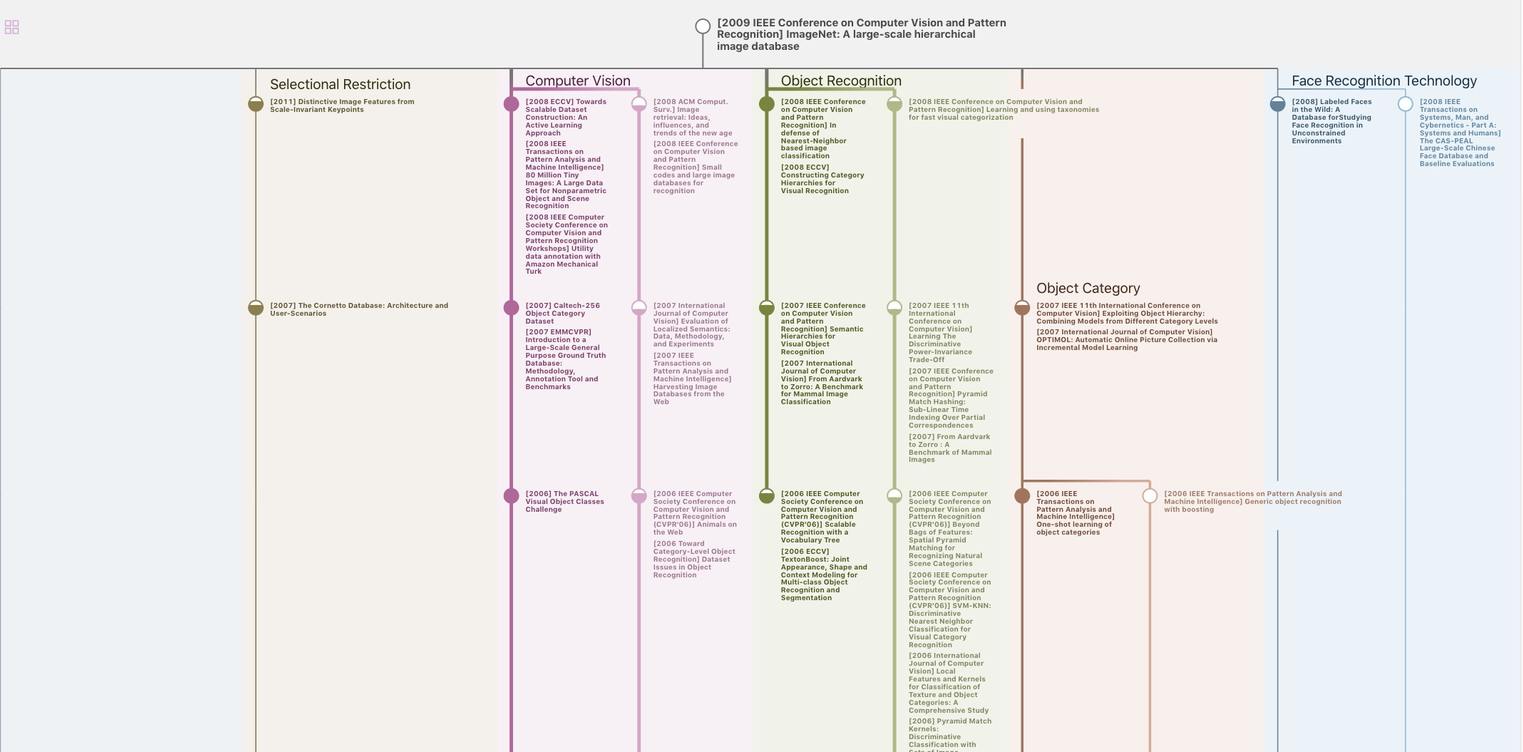
生成溯源树,研究论文发展脉络
Chat Paper
正在生成论文摘要