Collaborative knowledge distillation via filter knowledge transfer
EXPERT SYSTEMS WITH APPLICATIONS(2024)
摘要
Knowledge distillation is a promising model compression technique that generally distills the knowledge from a complex teacher model to a lightweight student model. However, the performance gain of a student model is usually limited by the capacity gap between the large teacher model and the small student model. In this paper, we propose a new collaborative knowledge distillation method that makes use of a new strategy, named Filter Knowledge Transfer (FKT) to detect and learn the valuable filter information following from the teacher to the student. To be specific, the useful knowledge of filters measured by using information entropy is transferred between different peer networks and unimportant filters are reactivated according to the ratio based on the characterized filter information entropy during the online distillation process. Experimental results derived on four popular datasets, CIFAR-10/100, Market-1501, and Tiny-ImageNet, demonstrate the superiority of our proposed method over the others we considered.
更多查看译文
关键词
Model compression,Knowledge distillation,Filter pruning,Filter knowledge transfer
AI 理解论文
溯源树
样例
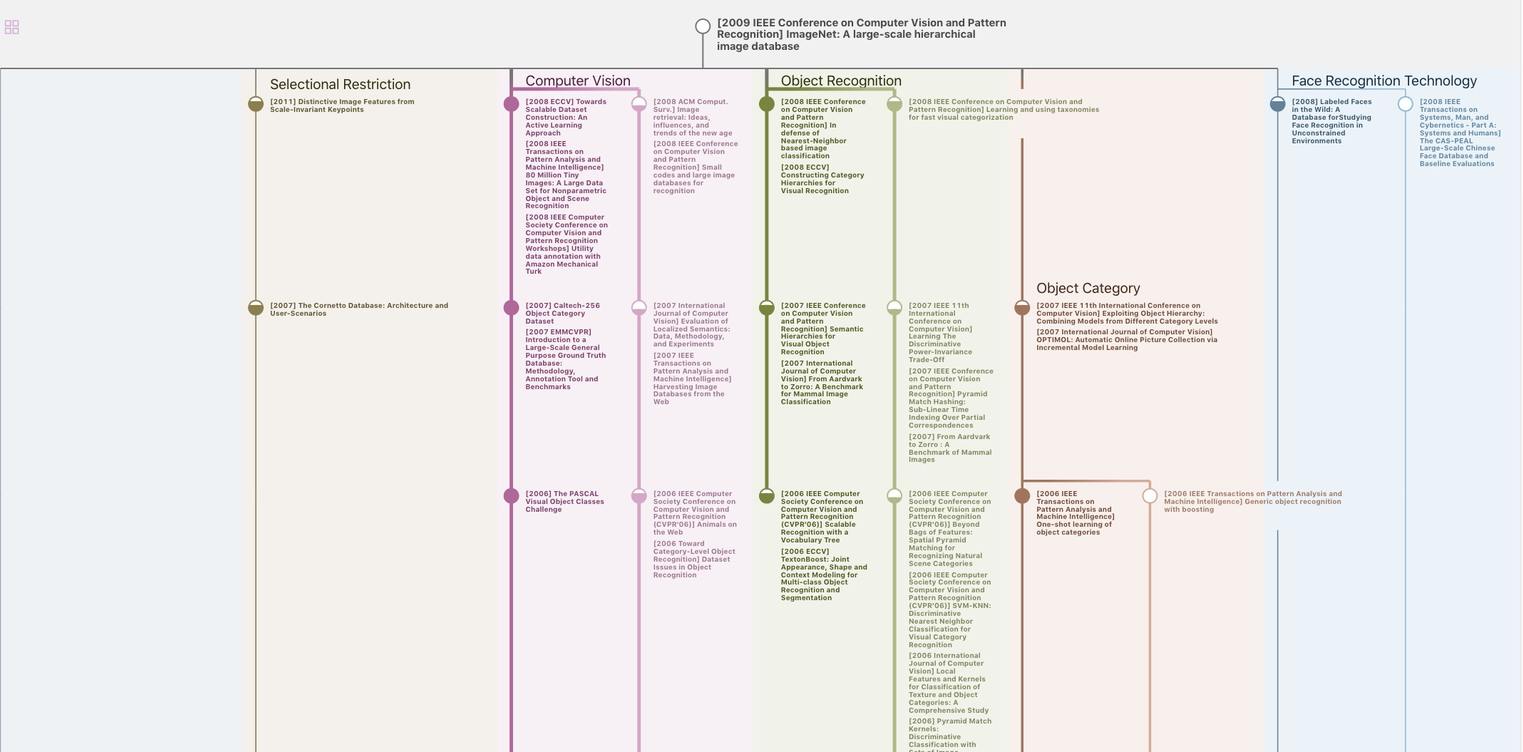
生成溯源树,研究论文发展脉络
Chat Paper
正在生成论文摘要