Different Methods of EEG Signal Analysis Using Power Spectral Density, ChronoNet and ResNest
European Journal of Electrical Engineering and Computer Science(2023)
摘要
Brain diseases like epilepsy can be identified using electroencephalograms (EEGs). Automated EEG data processing has the potential to improve patient care because manual interpretation requires a lot of time, resources, and money. The paper emphasizes that EEG data can be used to detect intellectual ability and human brain-related diseases like epilepsy. We propose one method ResNest for estimating Intelligence Quotient and two methods (Power spectral density (PSD) and ChronoNet) for detecting epilepsy. The first approach involves utilizing the datasets found at zenodo organization with 5-fold cross validation using the Welch PSD for feature extraction and various classifiers (Kernel SVM, Naive Bayes, Random Forest, Decision Tree). It was found that 99.1% accuracy could be achieved using Kernel SVM. We also propose ChronoNet, a cutting-edge architecture for recurrent neural networks that has been constructed using the Keras framework. It achieves an accuracy of roughly 98.89% by utilizing the data found at Temple University Hospital EEG corpus. The required datasets from Kaggle have been utilized in conjunction with the ResNest method. Using ResNest50d (Epochs=10), we achieved a maximum accuracy of 91%.
更多查看译文
关键词
eeg signal analysis,power spectral density
AI 理解论文
溯源树
样例
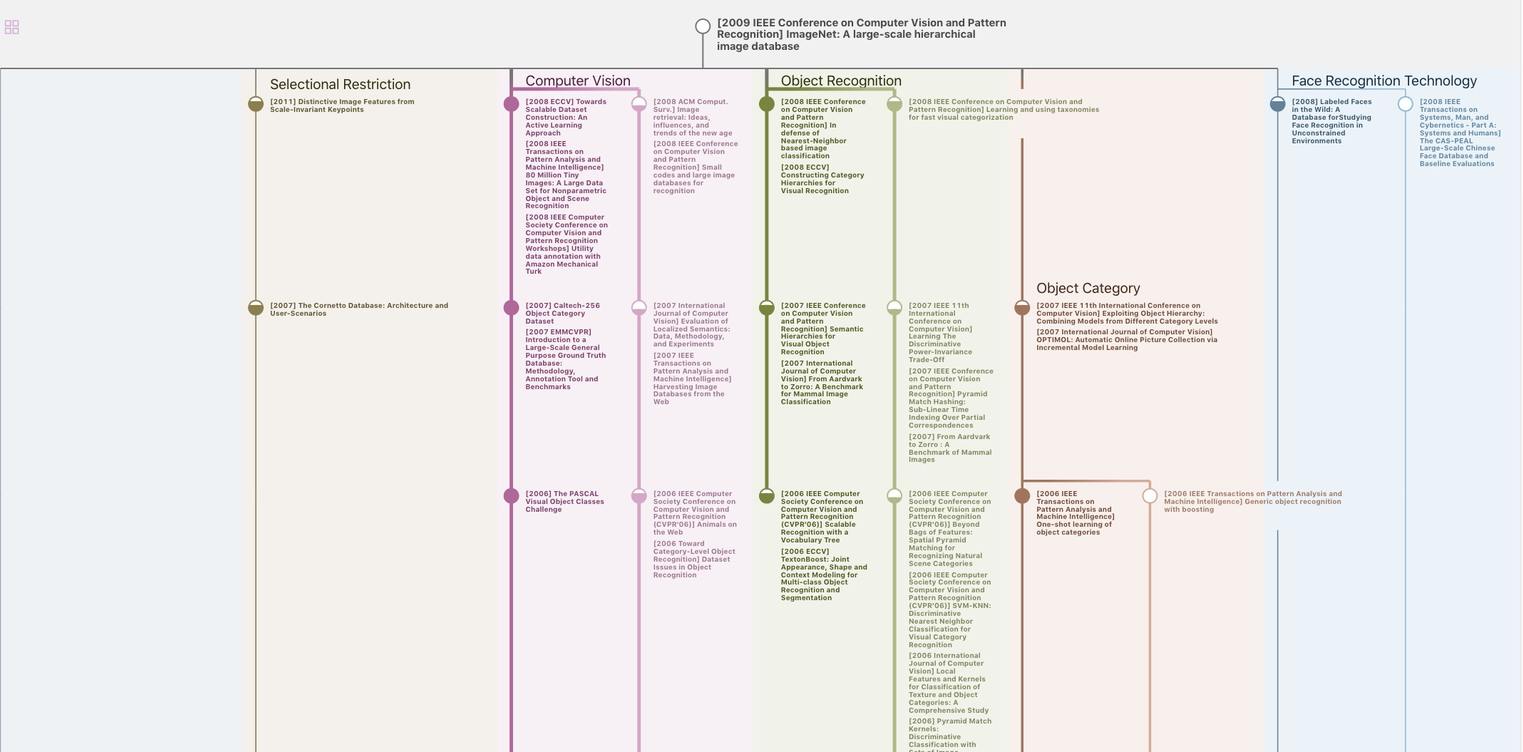
生成溯源树,研究论文发展脉络
Chat Paper
正在生成论文摘要