RingMo-Sense: Remote Sensing Foundation Model for Spatiotemporal Prediction via Spatiotemporal Evolution Disentangling
IEEE TRANSACTIONS ON GEOSCIENCE AND REMOTE SENSING(2023)
摘要
Remote sensing (RS) spatiotemporal prediction aims to infer future trends from historical spatiotemporal data, e.g., videos and time-series images, which has a broad application prospect in many fields. The foundation model is a promising research direction for spatiotemporal information mining because of its robust feature extraction capability and has made rapid progress in natural scenes. Nevertheless, due to the spatially multiscale and temporally multiscale properties in RS data, these methods still encounter bottlenecks when applied to RS. Therefore, we propose a remote sensing foundation model for spatiotemporal prediction via spatiotemporal evolution disentangling, abbreviated as RingMo-Sense. Considering spatial affinity, temporal continuity, and spatiotemporal interaction, we construct spatial, temporal, and spatiotemporal triple-branch prediction networks. Specifically, we use parameter-sharing and progressive joint training strategies to achieve stable long-range prediction and parameter reduction simultaneously. In addition, we build an RS spatiotemporal dataset by collecting various RS videos and time-series images. The experimental results on six downstream spatiotemporal tasks demonstrate that the proposed model yields competitive performance.
更多查看译文
关键词
Spatiotemporal evolution disentangling,spatiotemporal predictive foundation model
AI 理解论文
溯源树
样例
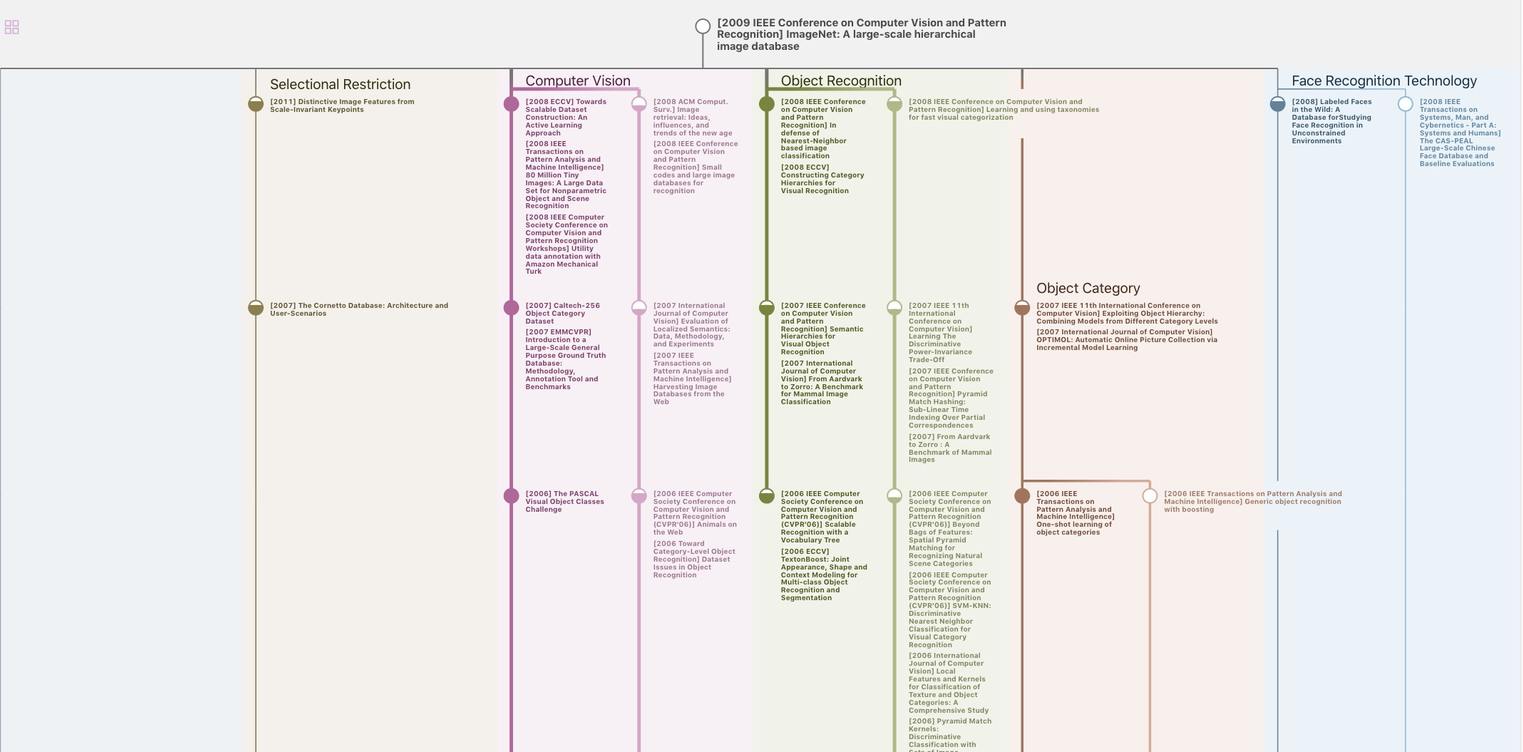
生成溯源树,研究论文发展脉络
Chat Paper
正在生成论文摘要