NetGO 3.0: A Protein Language Model Improves Large-scale Functional Annotations
Genomics, Proteomics & Bioinformatics(2023)
摘要
As one of the state-of-the-art automated function prediction (AFP) methods, NetGO 2.0 integrates multi-source information to improve the performance. However, it mainly utilizes the proteins with experimentally supported functional annotations without leveraging valuable information from a vast number of unannotated proteins. Recently, protein language models have been proposed to learn informative representations [e.g., Evolutionary Scale Modeling (ESM)-1b embedding] from protein sequences based on self-supervision. Here, we represented each protein by ESM-1b and used logistic regression (LR) to train a new model, LR-ESM, for AFP. The experimental results showed that LR-ESM achieved comparable performance with the best-performing component of NetGO 2.0. Therefore, by incorporating LR-ESM into NetGO 2.0, we developed NetGO 3.0 to improve the performance of AFP extensively. NetGO 3.0 is freely accessible at https://dmiip.sjtu.edu.cn/ng3.0.
更多查看译文
关键词
protein language model,large-scale
AI 理解论文
溯源树
样例
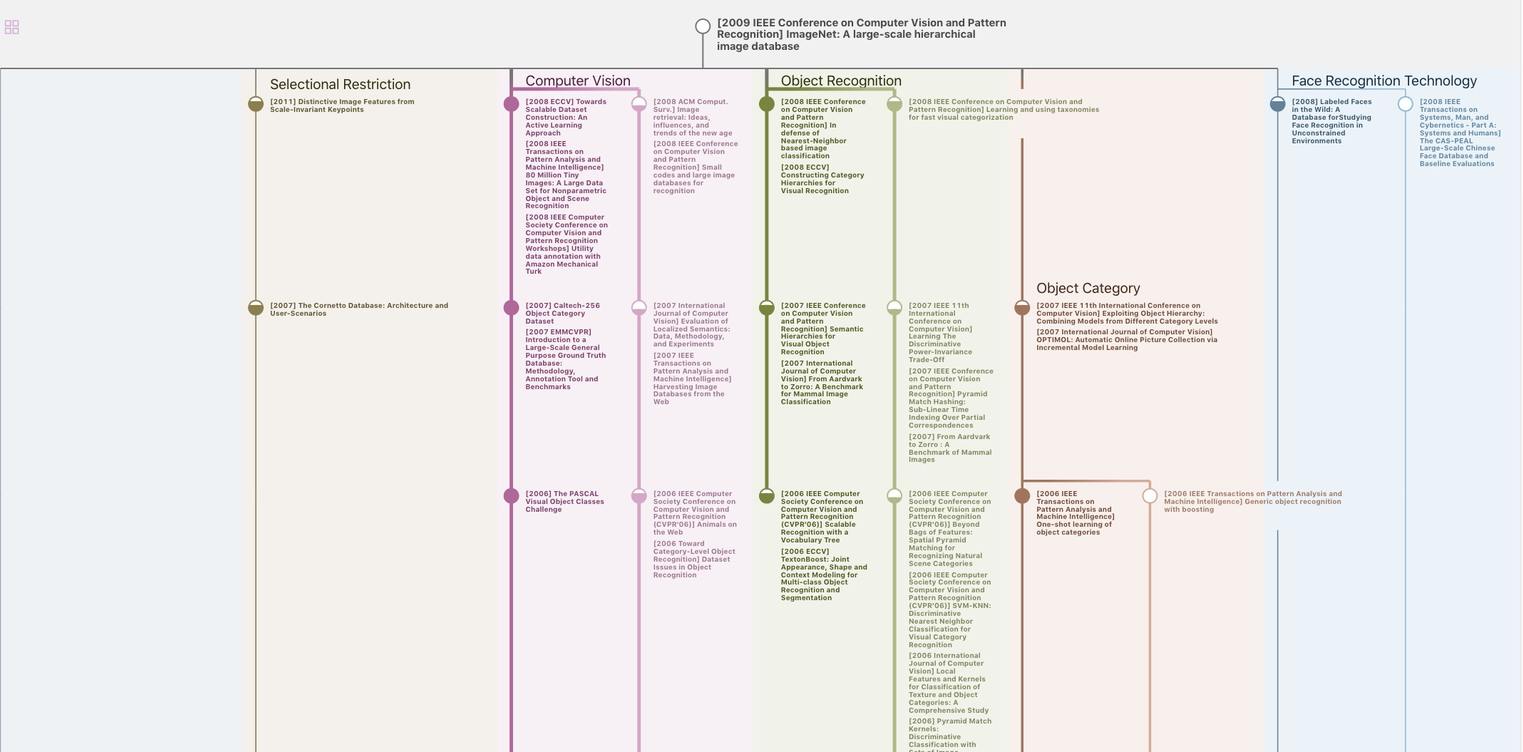
生成溯源树,研究论文发展脉络
Chat Paper
正在生成论文摘要