Machine learning reaction barriers in low data regimes: a horizontal and diagonal transfer learning approach
Digital discovery(2023)
摘要
Machine learning (ML) models can, once trained, make reaction barrier predictions in seconds, which is orders of magnitude faster than quantum mechanical (QM) methods such as density functional theory (DFT). However, these ML models need to be trained on large datasets of typically thousands of expensive, high accuracy barriers and do not generalise well beyond the specific reaction for which they are trained. In this work, we demonstrate that transfer learning (TL) can be used to adapt pre-trained Diels-Alder barrier prediction neural networks (NNs) to make predictions for other pericyclic reactions using horizontal TL (hTL) and additionally, at higher levels of theory with diagonal TL (dTL). TL-derived predictions are possible with mean absolute errors (MAEs) below the accepted chemical accuracy threshold of 1 kcal mol(-1), a significant improvement on pre-TL prediction MAEs of >5 kcal mol(-1), and in extremely low data regimes, with as few as 33 and 39 new datapoints needed for hTL and dTL, respectively. Thus, hTL and dTL are powerful options for providing insight into reaction feasibility without the need for extensive high-throughput experimental or computational screening or large dataset generation for training bespoke ML models.
更多查看译文
关键词
transfer learning,reaction barriers,transfer learning approach,low data regimes,machine learning
AI 理解论文
溯源树
样例
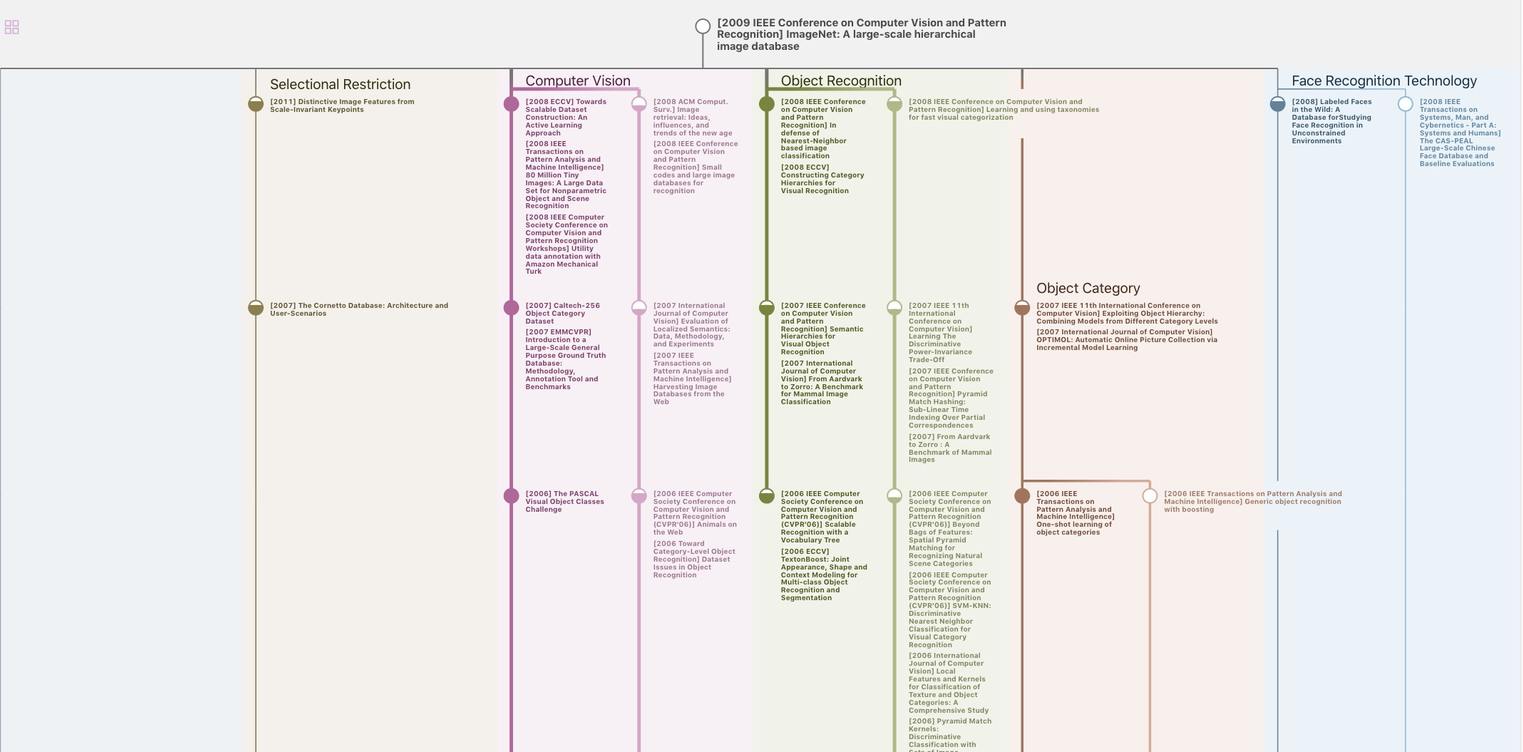
生成溯源树,研究论文发展脉络
Chat Paper
正在生成论文摘要