Ask more, know better: Reinforce-Learned Prompt Questions for Decision Making with Large Language Models
CoRR(2023)
摘要
Large language models (LLMs) demonstrate their promise in tackling
complicated practical challenges by combining action-based policies with chain
of thought (CoT) reasoning. Having high-quality prompts on hand, however, is
vital to the framework's effectiveness. Currently, these prompts are
handcrafted utilising extensive human labor, resulting in CoT policies that
frequently fail to generalise. Human intervention is also required to develop
grounding functions that ensure low-level controllers appropriately process CoT
reasoning. In this paper, we propose a comprehensive training framework for
complex task-solving, incorporating human prior knowledge into the learning of
action policies. To that purpose, we offer a new leader-follower bilevel
framework that is capable of learning to ask relevant questions (prompts) and
subsequently undertaking reasoning to guide the learning of actions. The prompt
policy is employed to make introspective revisions based on historical
findings, leading the CoT process to consider the anticipated goals and
generate outputs that lead to decisive, high-performing actions. The action
policy subsequently learns to comprehend and integrate the CoT outputs to take
actions. Our empirical data reveal that our framework outperforms leading
methods in 5 decision-making tasks such as Overcooked and FourRoom.
更多查看译文
AI 理解论文
溯源树
样例
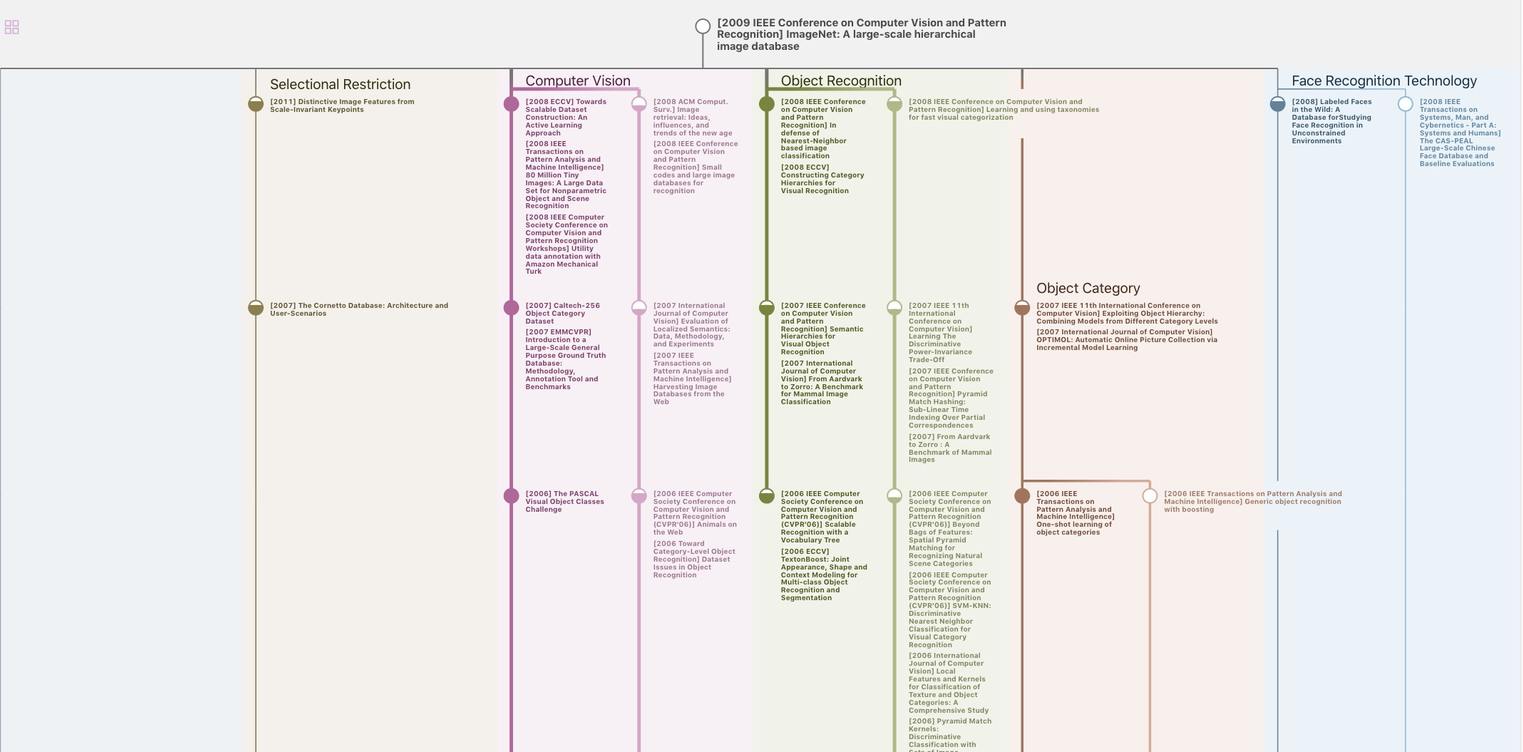
生成溯源树,研究论文发展脉络
Chat Paper
正在生成论文摘要