A Spectral Condition for Feature Learning
CoRR(2023)
摘要
The push to train ever larger neural networks has motivated the study of initialization and training at large network width. A key challenge is to scale training so that a network's internal representations evolve nontrivially at all widths, a process known as feature learning. Here, we show that feature learning is achieved by scaling the spectral norm of weight matrices and their updates like $\sqrt{\texttt{fan-out}/\texttt{fan-in}}$, in contrast to widely used but heuristic scalings based on Frobenius norm and entry size. Our spectral scaling analysis also leads to an elementary derivation of \emph{maximal update parametrization}. All in all, we aim to provide the reader with a solid conceptual understanding of feature learning in neural networks.
更多查看译文
关键词
feature learning,spectral condition
AI 理解论文
溯源树
样例
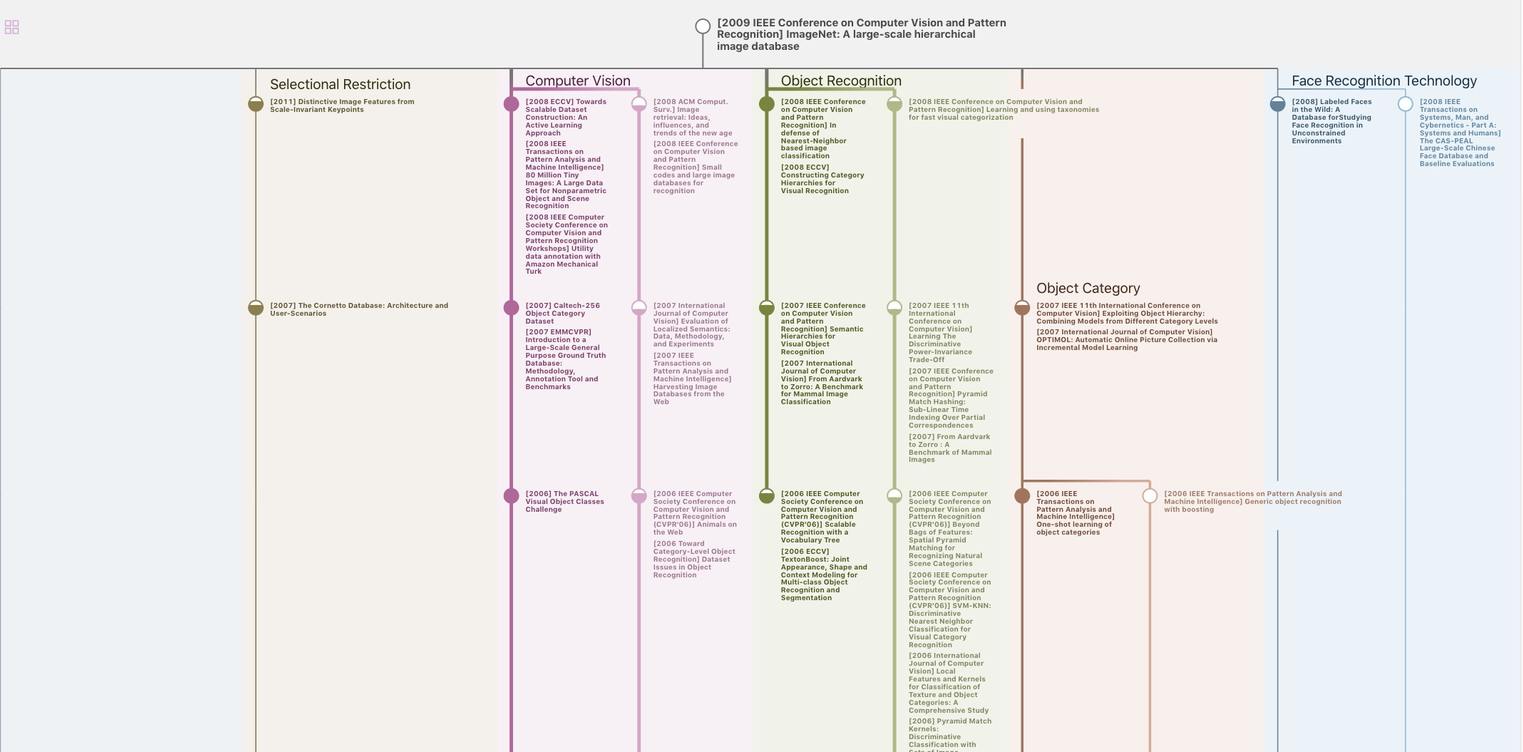
生成溯源树,研究论文发展脉络
Chat Paper
正在生成论文摘要