Fine-Tuning Pre-Trained Language Model for Urgency Classification on Food Safety Feedback
2023 10th International Conference on ICT for Smart Society (ICISS)(2023)
摘要
Singapore Food Agency (SFA) receives hundreds of food safety feedback reports regarding Singapore's food safety every week, which can be time-consuming and costly to manage them. Prompt response to urgent food safety feedback is crucial in cases of food poisoning outbreaks. Automating the task of feedback urgency classification can help SFA officers to prioritise feedback efficiently and effectively, so that they can respond quickly to urgent cases. In this paper, we propose an approach to fine-tune a pre-trained language model based on BERT, which is a sequence classification task, for feedback urgency classification. In addition, to speed up the labeling of task-specific feedback data, we also propose a process that utilizes the zero-shot text classification and decision tree methods for data labeling with minimal human supervision. We have conducted experiments to evaluate the proposed fine-tuned BERT model and compared with the DistilBERT and XLNet models for the feedback urgency classification task. The performance results show that the proposed fine-tuned BERT model has achieved promising performance and outperformed the fine-tuned DistilBERT and XLNet models by 7% and 5%, respectively in macro-averaged F1-score.
更多查看译文
关键词
feedback urgency classification,deep learning,natural language processing
AI 理解论文
溯源树
样例
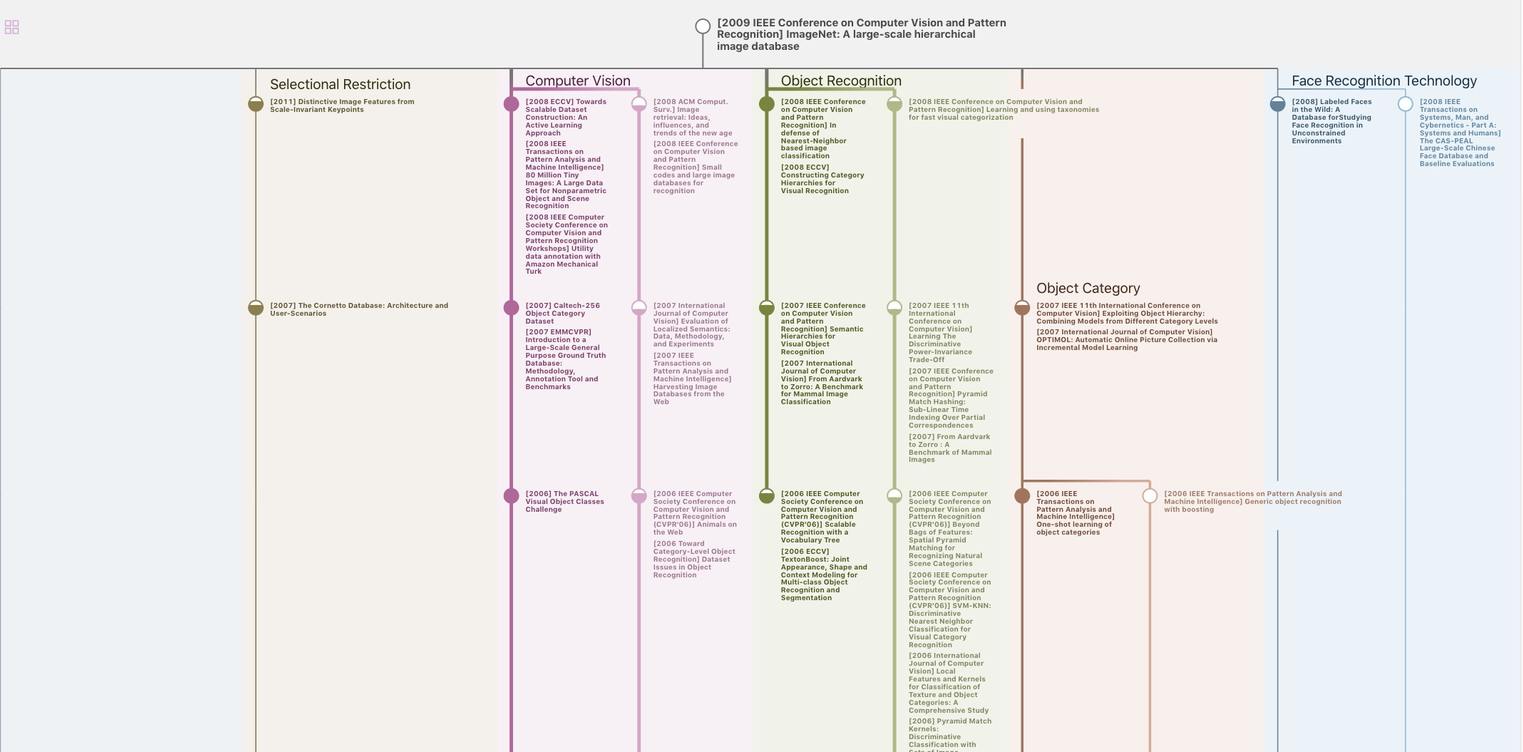
生成溯源树,研究论文发展脉络
Chat Paper
正在生成论文摘要