Online Adaptive Integral Reinforcement Learning for Nonlinear Multi-Input System
IEEE Transactions on Circuits and Systems II: Express Briefs(2023)
摘要
In this brief article, a novel adaptive integral reinforcement learning (AIRL) scheme is proposed for the continuous-time (CT) system. Moreover, it is used to learn the optimal controls of the partially unknown multi-input nonlinear system. Firstly, the Nash equilibrium of multi-input is defined. Two neural networks (NN) are used to approximate the cost functions with the integral reinforcement signal, which can avoid directly solving the Hamilton–Jacobi–Bellman (HJB) equation such that dynamic information and derivatives of NN activations are not needed. Then, a novel learning algorithm is used to update the unknown NN weights. The studied weights are used to obtain the optimum multi-policies. The learned weight convergence is proved. Finally, two examples are presented to verify the system performance with the proposed AIRL scheme.
更多查看译文
关键词
reinforcement,learning
AI 理解论文
溯源树
样例
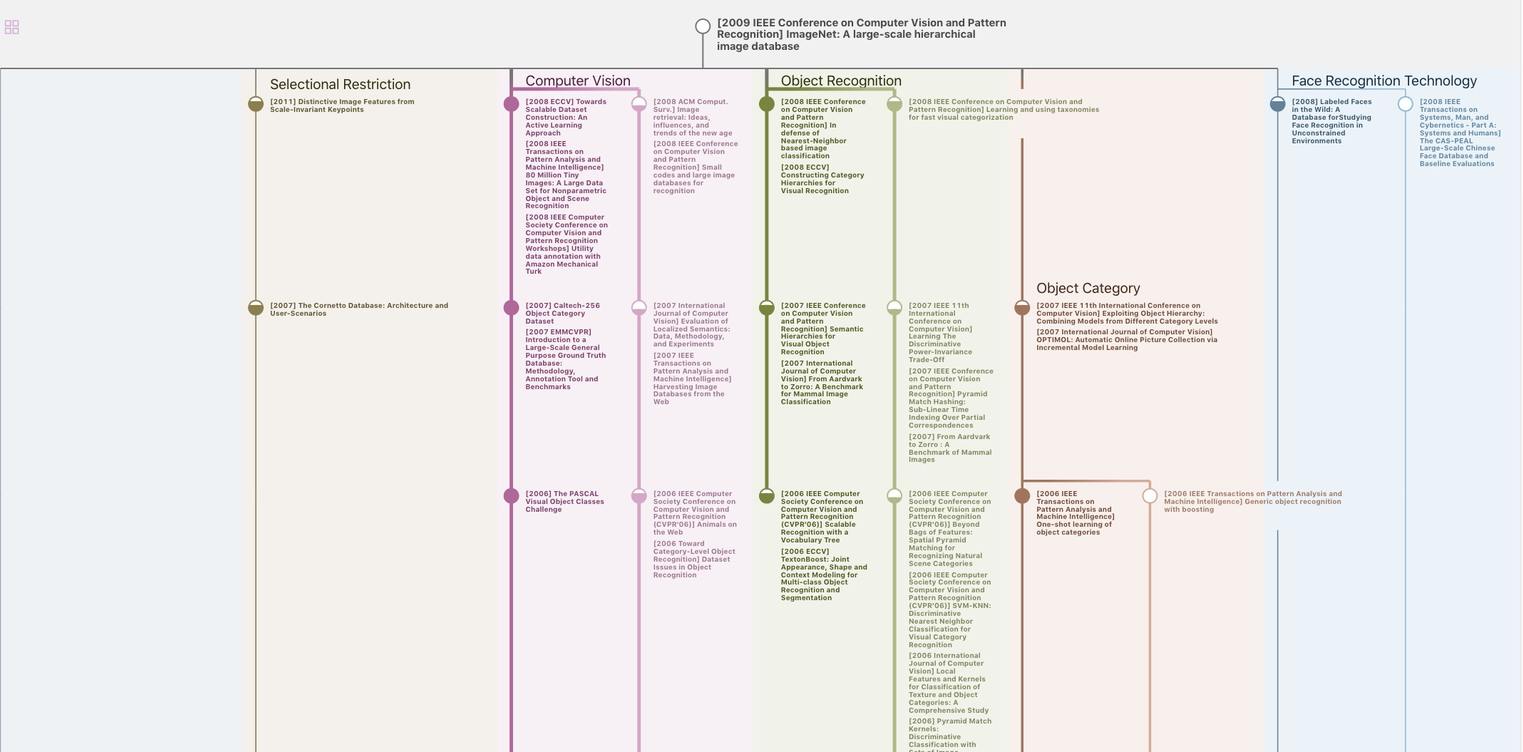
生成溯源树,研究论文发展脉络
Chat Paper
正在生成论文摘要