Explainable Approaches for Forecasting Building Electricity Consumption
ENERGIES(2023)
摘要
Building electric energy is characterized by a significant increase in its uses (e.g., vehicle charging), a rapidly declining cost of all related data collection, and a proliferation of smart grid concepts, including diverse and flexible electricity pricing schemes. Not surprisingly, an increased number of approaches have been proposed for its modeling and forecasting. In this work, we place our emphasis on three forecasting-related issues. First, we look at the forecasting explainability, that is, the ability to understand and explain to the user what shapes the forecast. To this extent, we rely on concepts and approaches that are inherently explainable, such as the evolutionary approach of genetic programming (GP) and its associated symbolic expressions, as well as the so-called SHAP (SHapley Additive eXplanations) values, which is a well-established model agnostic approach for explainability, especially in terms of feature importance. Second, we investigate the impact of the training timeframe on the forecasting accuracy; this is driven by the realization that fast training would allow for faster deployment of forecasting in real-life solutions. And third, we explore the concept of counterfactual analysis on actionable features, that is, features that the user can really act upon and which therefore present an inherent advantage when it comes to decision support. We have found that SHAP values can provide important insights into the model explainability. In our analysis, GP models demonstrated superior performance compared to neural network-based models (with a 20-30% reduction in Root Mean Square Error (RMSE)) and time series models (with a 20-40% lower RMSE), but a rather questionable potential to produce crisp and insightful symbolic expressions, allowing a better insight into the model performance. We have also found and reported here on an important potential, especially for practical, decision support, of counterfactuals built on actionable features, and short training timeframes.
更多查看译文
关键词
electricity demand forecasting,model explainability,SHAP values,neural networks,structured time series,genetic programming (GP),symbolic expressions,training timeframe,counterfactuals,actionable features
AI 理解论文
溯源树
样例
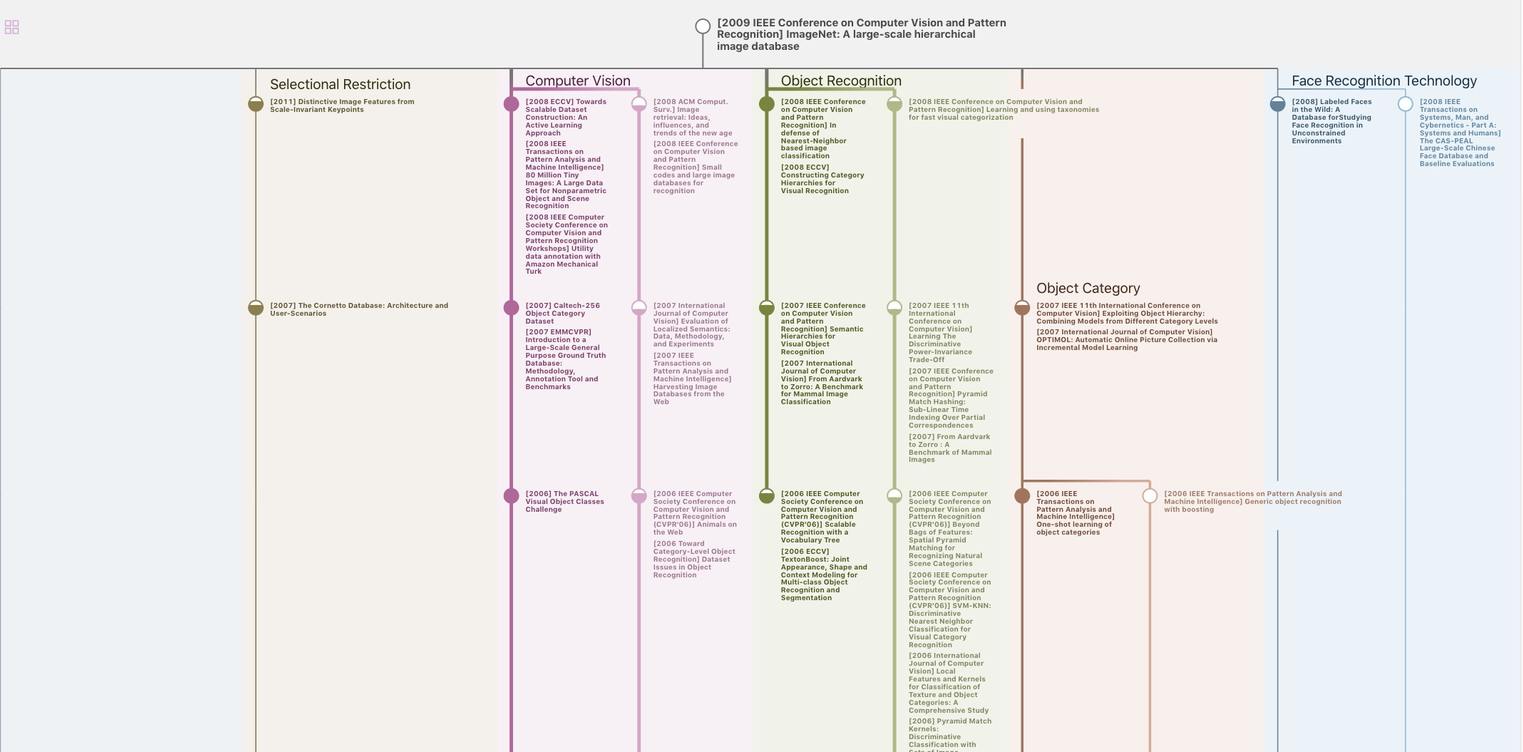
生成溯源树,研究论文发展脉络
Chat Paper
正在生成论文摘要