Optimizing Task Completion Time in Disaster-Affected Regions with the WMDDPG-GSA Algorithm for UAV-Assisted MEC Systems
Processes(2023)
摘要
In this paper, we investigate a UAV-assisted Mobile Edge Computing (MEC) system that is deployed with multiple UAVs to provide timely data processing services to disaster-stricken areas. In our model, since base stations are unavailable in disaster-affected areas, we solely employ UAVs as MEC servers, as well as enable real-time data transmission during UAV flights by estimating and compensating for the Doppler Frequency Shift (DFS). Subsequently, an optimization problem is formulated to jointly optimize the trajectories and offloading strategies of multiple UAVs to minimize the task completion time. We enhance the performance of the Multi-Agent Deep Deterministic Policy Gradient (MADDPG) algorithm by using a weighted strategy algorithm, and we thus propose the Weighted-Strategy-Based Multi-Agent Deep Deterministic Policy Gradient (WMDDPG) algorithm for optimizing UAV trajectories. We employ the Greedy-Based Simulated Annealing (GSA) algorithm to overcome the limitations of the greedy algorithm and to obtain the best offloading strategy. The results demonstrate the effectiveness of the proposed WMDDPG-GSA algorithm, as it outperforms benchmark algorithms.
更多查看译文
关键词
UAV,Mobile Edge Computing (MEC),Multi-Agent Deep Reinforcement Learning,Doppler Frequency Shift (DFS)
AI 理解论文
溯源树
样例
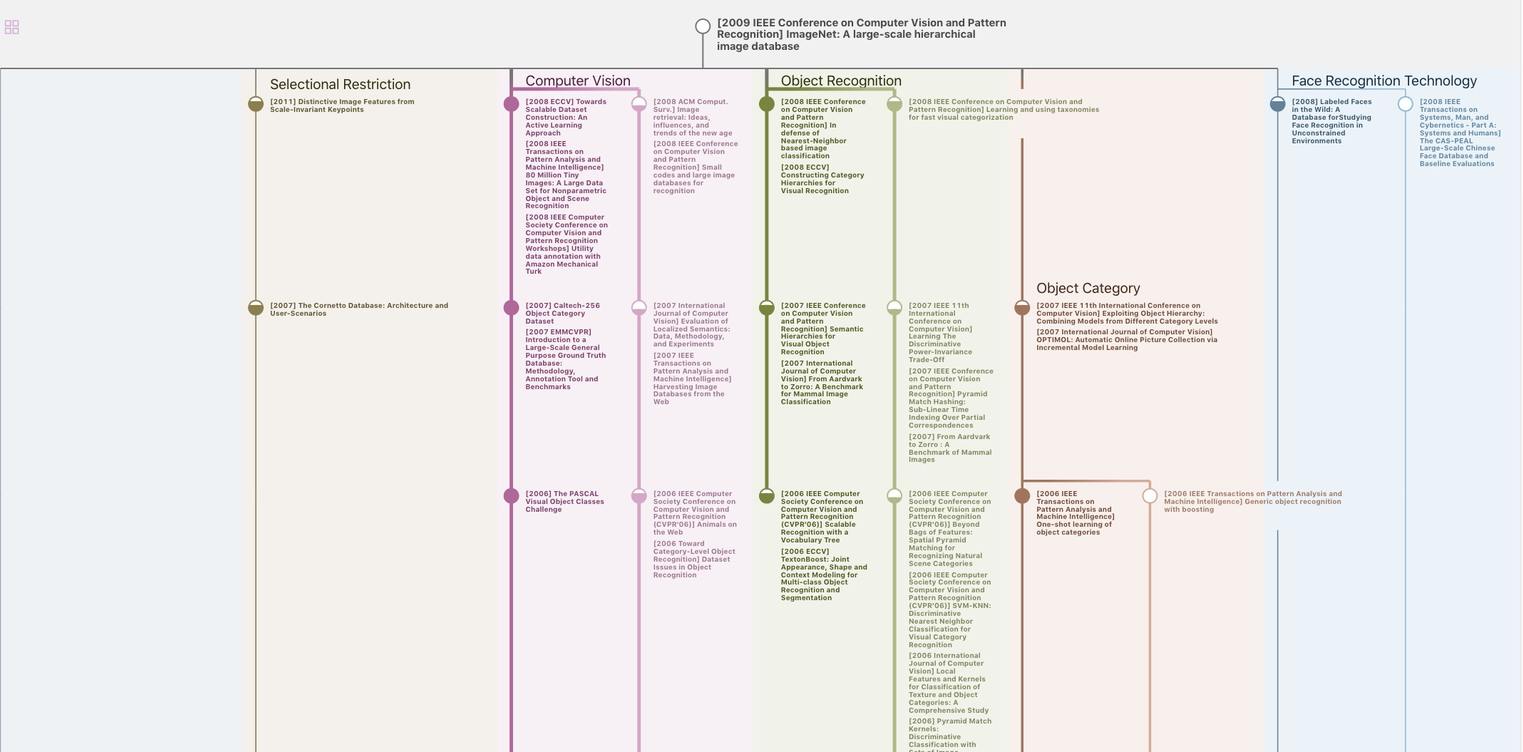
生成溯源树,研究论文发展脉络
Chat Paper
正在生成论文摘要