Decepticon: Attacking Secrets of Transformers
2023 IEEE INTERNATIONAL SYMPOSIUM ON WORKLOAD CHARACTERIZATION, IISWC(2023)
摘要
With the growing burden of training deep learning models with huge datasets, transfer learning has been widely adopted (e.g., Transformers like BERT, GPT). Transfer learning significantly reduces the time and effort of model training. However, the security impact of using shared pre-trained models has not been evaluated. In this paper, we provide in-depth characterizations of the fine-tuning process and reveal the security vulnerabilities of transfer-learned models. Then, we show a novel two-level model extraction attack; 1) identifying the pre-trained model of a victim transfer-learned model through model fingerprint collected from off-the-shelf GPUs and 2) extracting the entire weights of the victim blackbox model based on the hints in the pre-trained model. The extracted model shows almost alike prediction accuracy with over 94% matching prediction outputs with the victim model. The two-level model extraction enables large model weight extraction that is considered as challenging if not impossible through significantly reduced extraction effort.
更多查看译文
AI 理解论文
溯源树
样例
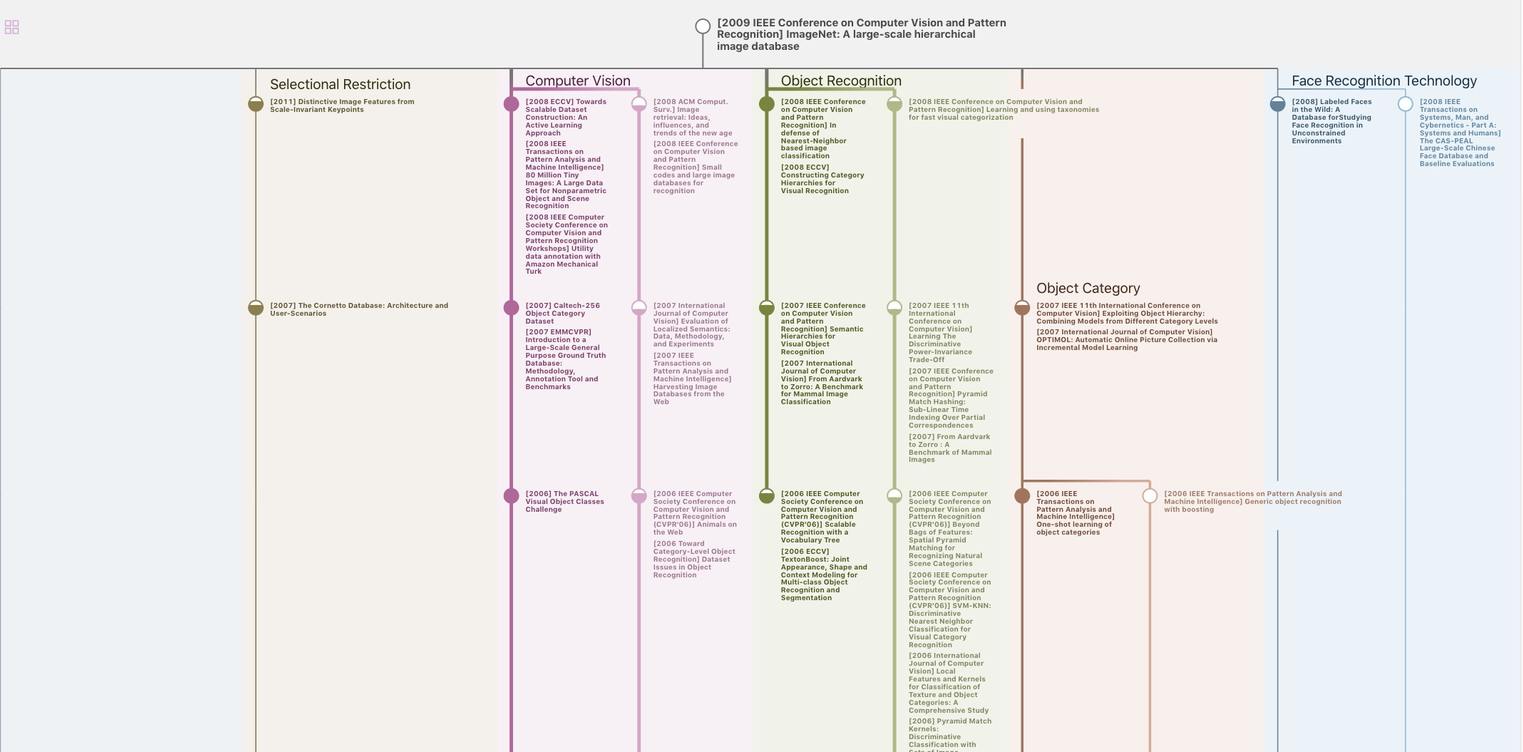
生成溯源树,研究论文发展脉络
Chat Paper
正在生成论文摘要