Topological feature selection for time series data
arXiv (Cornell University)(2023)
摘要
We use tools from applied topology for feature selection on vector-valued time series data. We employ persistent homology and sliding window embeddings to quantify the coordinated dynamics of time series. We describe an algorithm for gradient descent to assigns scores, or weights, to the variables of the time series based on their contribution to the dynamics as quantified by persistent homology; the result is a convex combination of a subset of the variables. In this setting, we prove persistence vineyards are piecewise linear and we give a simple formula for the derivatives of the vines. We demonstrate our method of topological feature selection with synthetic data and C. elegans neuronal data.
更多查看译文
关键词
topological feature selection,feature selection,time series
AI 理解论文
溯源树
样例
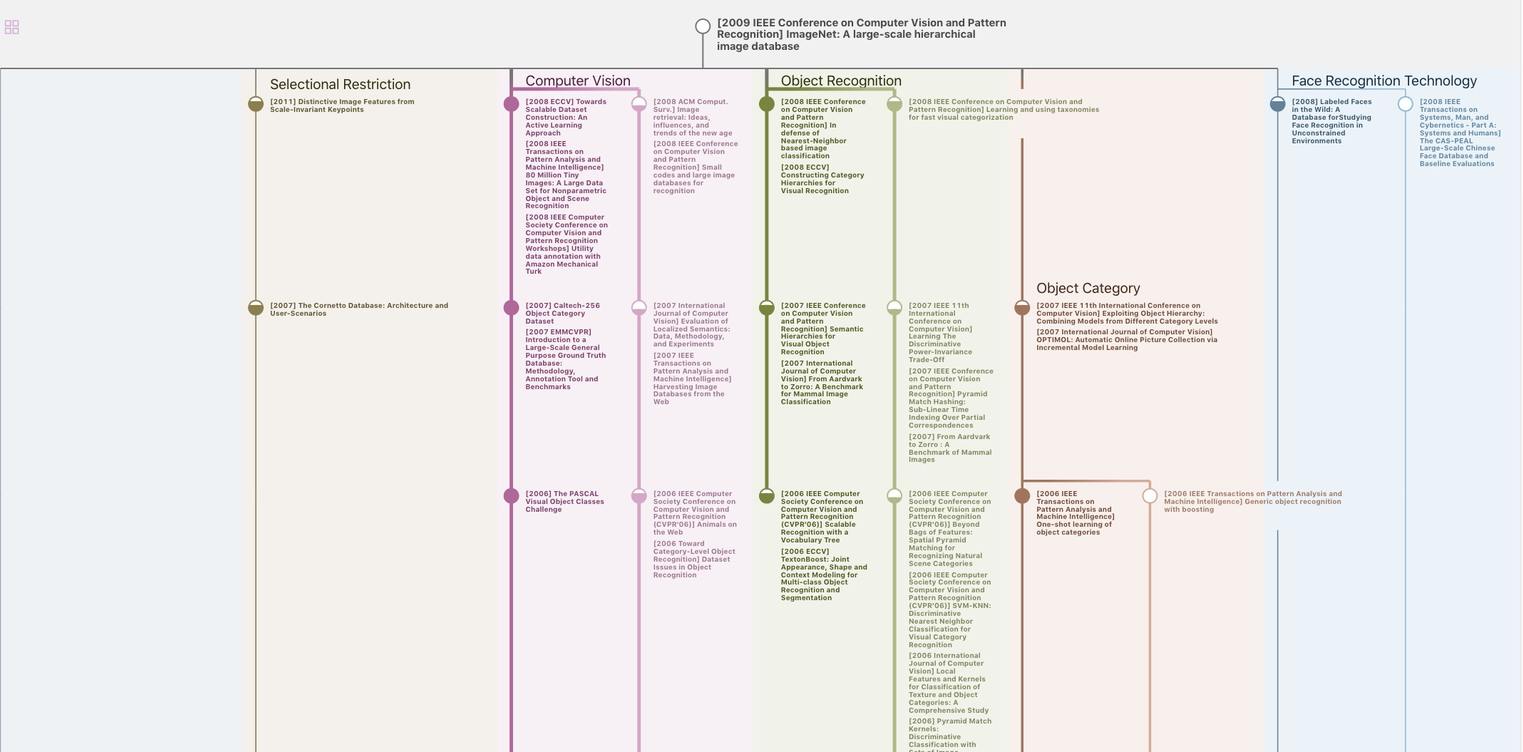
生成溯源树,研究论文发展脉络
Chat Paper
正在生成论文摘要