BEVContrast: Self-Supervision in BEV Space for Automotive Lidar Point Clouds
CoRR(2023)
摘要
We present a surprisingly simple and efficient method for self-supervision of 3D backbone on automotive Lidar point clouds. We design a contrastive loss between features of Lidar scans captured in the same scene. Several such approaches have been proposed in the literature from PointConstrast, which uses a contrast at the level of points, to the state-of-the-art TARL, which uses a contrast at the level of segments, roughly corresponding to objects. While the former enjoys a great simplicity of implementation, it is surpassed by the latter, which however requires a costly pre-processing. In BEVContrast, we define our contrast at the level of 2D cells in the Bird's Eye View plane. Resulting cell-level representations offer a good trade-off between the point-level representations exploited in PointContrast and segment-level representations exploited in TARL: we retain the simplicity of PointContrast (cell representations are cheap to compute) while surpassing the performance of TARL in downstream semantic segmentation.
更多查看译文
关键词
automotive lidar point
AI 理解论文
溯源树
样例
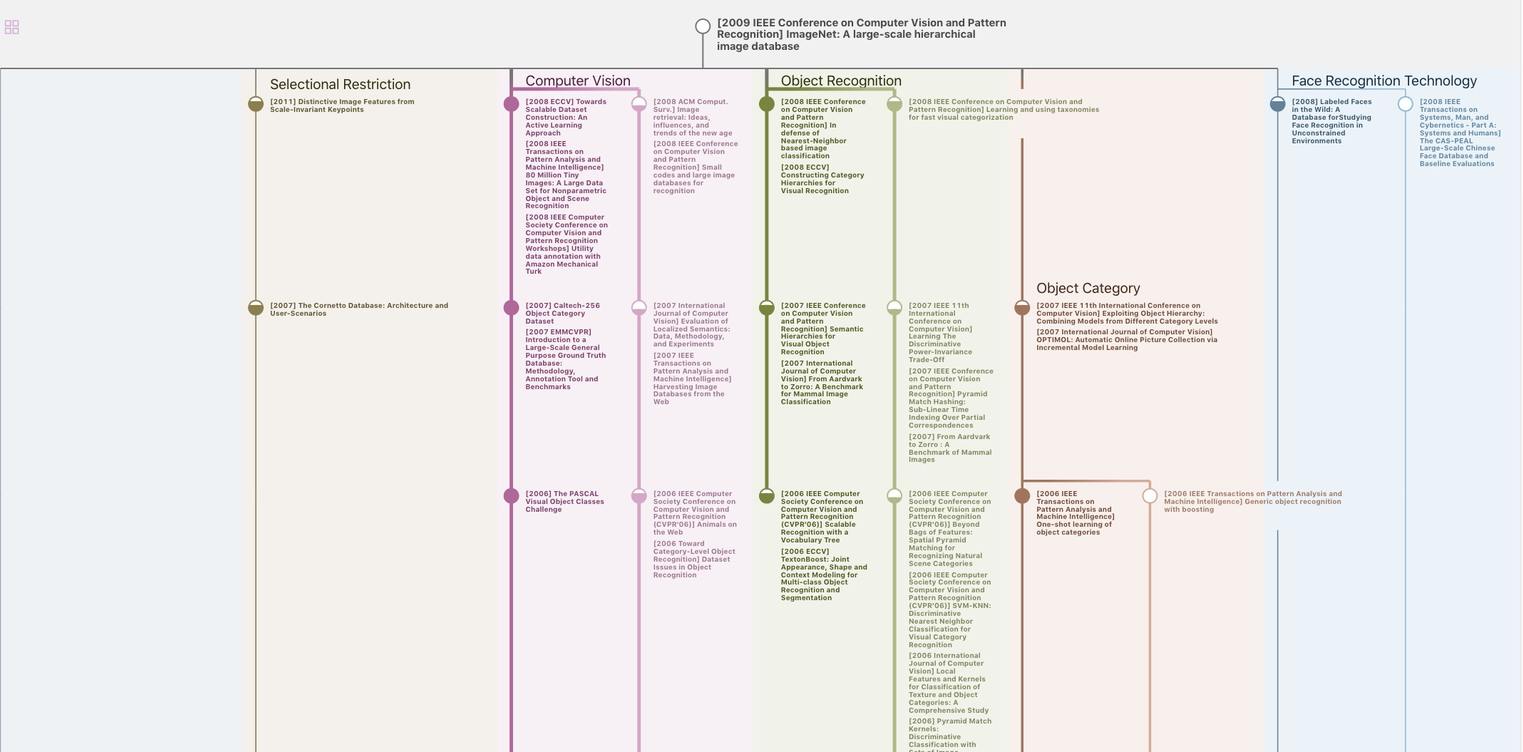
生成溯源树,研究论文发展脉络
Chat Paper
正在生成论文摘要