Faster Recalibration of an Online Predictor via Approachability
CoRR(2023)
摘要
Predictive models in ML need to be trustworthy and reliable, which often at the very least means outputting calibrated probabilities. This can be particularly difficult to guarantee in the online prediction setting when the outcome sequence can be generated adversarially. In this paper we introduce a technique using Blackwell's approachability theorem for taking an online predictive model which might not be calibrated and transforming its predictions to calibrated predictions without much increase to the loss of the original model. Our proposed algorithm achieves calibration and accuracy at a faster rate than existing techniques arXiv:1607.03594 and is the first algorithm to offer a flexible tradeoff between calibration error and accuracy in the online setting. We demonstrate this by characterizing the space of jointly achievable calibration and regret using our technique.
更多查看译文
关键词
online predictor,faster recalibration
AI 理解论文
溯源树
样例
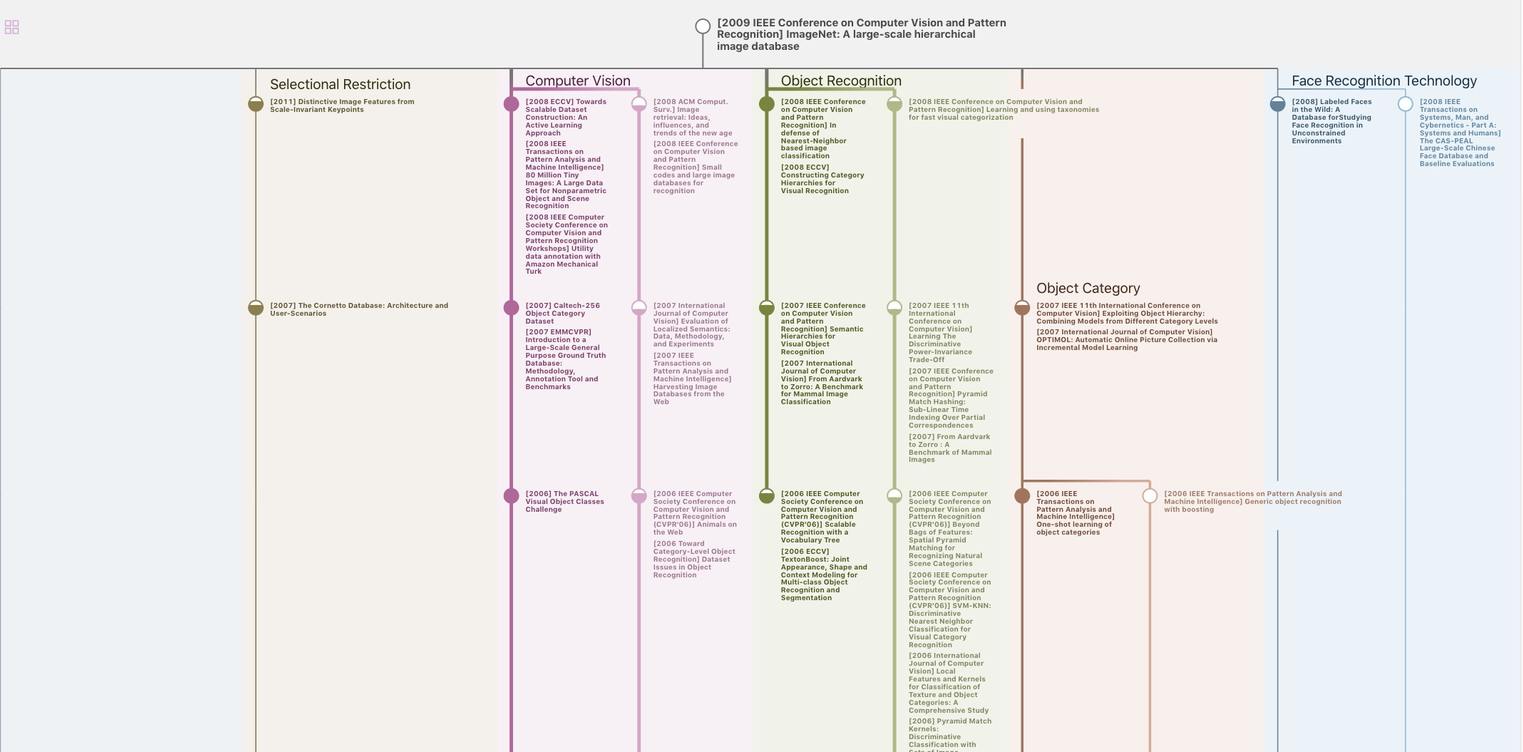
生成溯源树,研究论文发展脉络
Chat Paper
正在生成论文摘要