Efficient Neural Network Approaches for Conditional Optimal Transport with Applications in Bayesian Inference
CoRR(2023)
摘要
We present two neural network approaches that approximate the solutions of static and dynamic conditional optimal transport (COT) problems, respectively. Both approaches enable sampling and density estimation of conditional probability distributions, which are core tasks in Bayesian inference. Our methods represent the target conditional distributions as transformations of a tractable reference distribution and, therefore, fall into the framework of measure transport. COT maps are a canonical choice within this framework, with desirable properties such as uniqueness and monotonicity. However, the associated COT problems are computationally challenging, even in moderate dimensions. To improve the scalability, our numerical algorithms leverage neural networks to parameterize COT maps. Our methods exploit the structure of the static and dynamic formulations of the COT problem. PCP-Map models conditional transport maps as the gradient of a partially input convex neural network (PICNN) and uses a novel numerical implementation to increase computational efficiency compared to state-of-the-art alternatives. COT-Flow models conditional transports via the flow of a regularized neural ODE; it is slower to train but offers faster sampling. We demonstrate their effectiveness and efficiency by comparing them with state-of-the-art approaches using benchmark datasets and Bayesian inverse problems.
更多查看译文
关键词
conditional optimal transport
AI 理解论文
溯源树
样例
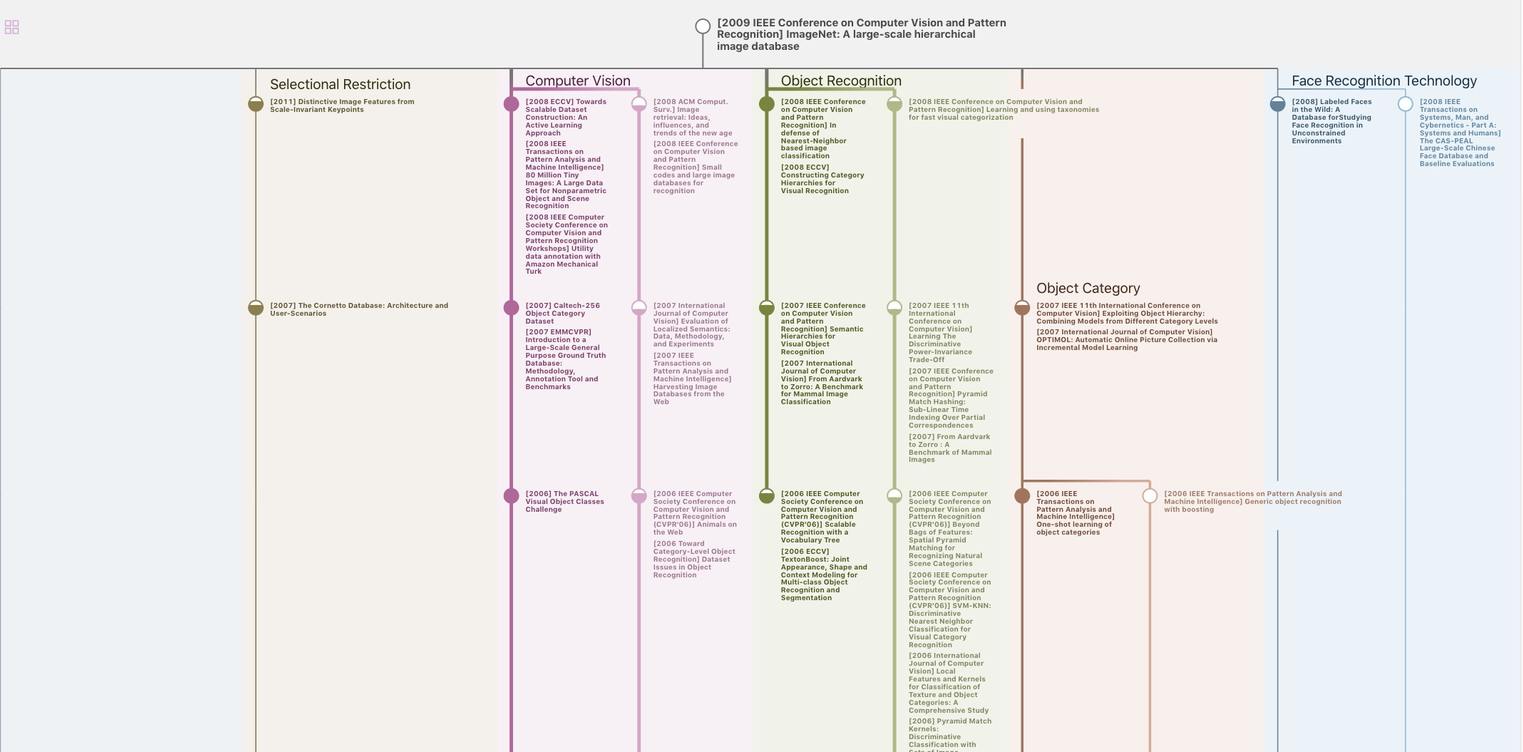
生成溯源树,研究论文发展脉络
Chat Paper
正在生成论文摘要