Comparative analysis of multiple YOLO-based target detectors and trackers for ADAS in edge devices
ROBOTICS AND AUTONOMOUS SYSTEMS(2024)
摘要
Accurate detection and tracking of vulnerable road users and traffic objects represent vital tasks for autonomous driving and driving assistance systems. The recent developments in the field rely heavily on deep learning techniques, which require large computational resources. Thus, optimizing software components alongside appropriate hardware choices is imperative for the development of a system that can perform real-time inference. In this paper, we propose a solution for object detection and tracking in an autonomous driving scenario. Specifically, we compare and explore the applicability of various state-of-the-art object detectors trained on the BDD100K dataset, including YOLOv8, YOLOv7, YOLOv5, Scaled-YOLOv4, and YOLOR. Moreover, we investigate the deployment of these algorithms on edge devices, such as the NVIDIA Jetson AGX Xavier. Additionally, we assess the effectiveness of DeepStream technology for real-time inference, comparing different object trackers, such as NvDCF and DeepSORT, using the KITTI tracking dataset. The suggested approach entails utilizing a YOLOR-CSP architecture in conjunction with a DeepSORT tracker, to achieve a real-time inference rate of 33.3 frames per second (FPS) with a detection interval of one, and a 17 FPS rate with an interval of zero.
更多查看译文
关键词
YOLOv8,Object detection,Object tracking,Edge devices autonomous vehicles
AI 理解论文
溯源树
样例
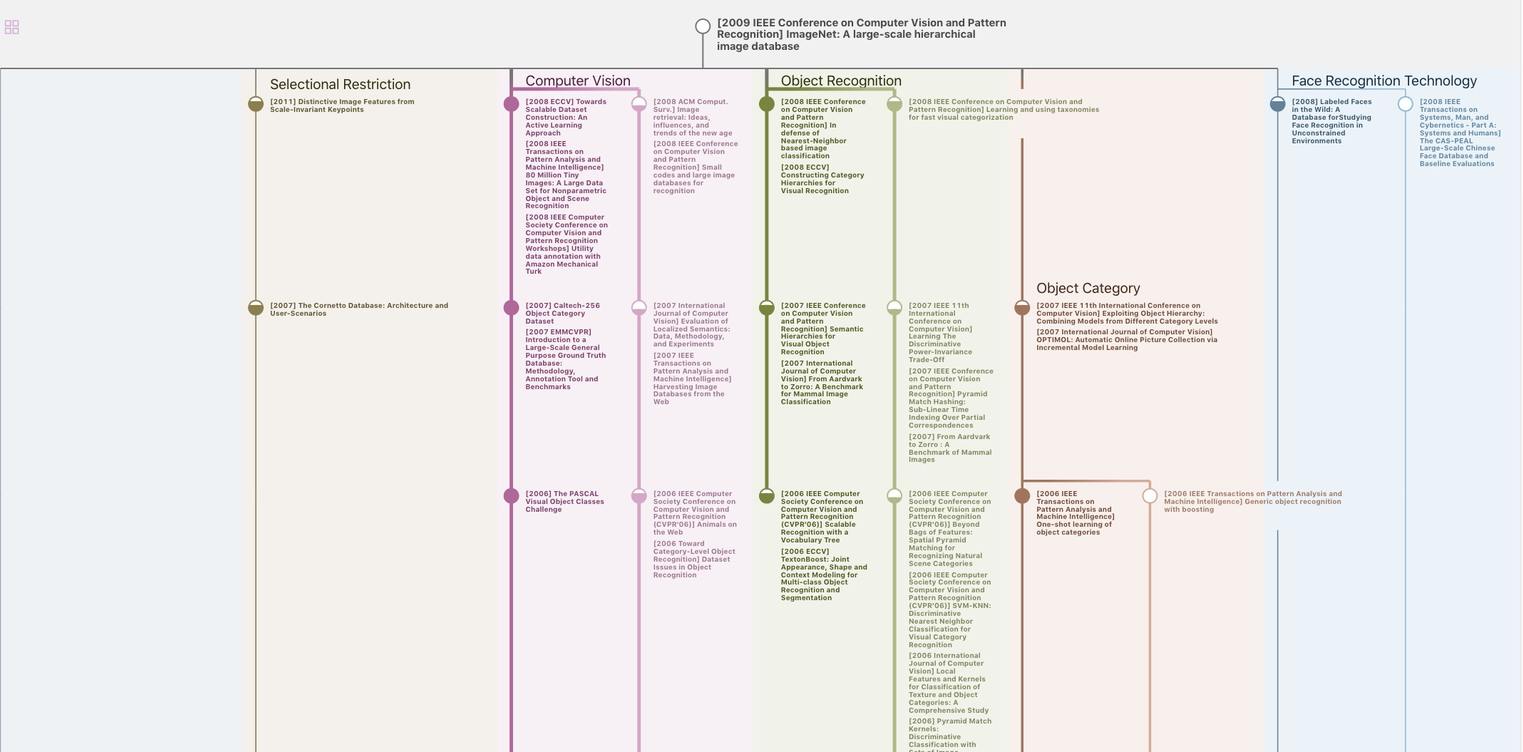
生成溯源树,研究论文发展脉络
Chat Paper
正在生成论文摘要