MISL: Multi-grained image-text semantic learning for text-guided image inpainting
PATTERN RECOGNITION(2024)
摘要
Text-guided image inpainting aims to generate corrupted image patches and obtain a plausible image based on textual descriptions, considering the relationship between textual and visual semantics. Existing works focus on predicting missing patches from the residual pixels of corrupted images, ignoring the visual semantics of the objects of interest in the images corresponding to the textual descriptions. In this paper, we propose a text-guided image inpainting method with multi-grained image-text semantic learning (MISL), consisting of global-local generators and discriminators. More specifically, we devise hierarchical learning (HL) with global -coarse-grained, object-fine-grained, and global-fine-grained learning stages in the global-local generators to refine the corrupted images from the global to local. In particular, the object-fine-grained learning stage focuses on the visual semantics of objects of interest in corrupted images by using an encoder-decoder network with self-attention blocks. Not only that, we design a mask reconstruction (MR) module to further act on the restoration of the objects of interest corresponding to the given textual descriptions. To inject the textual semantics into the global-local generators, we implement a multi-attention (MA) module that incorporates the word-level and sentence-level textual features to generate three different-grained images. For training, we exploit a global discriminator and a flexible discriminator to penalize the whole image and the corrupted region, respectively. Extensive experiments conducted on four datasets show the outperformance of the proposed MISL.
更多查看译文
关键词
Text-guided image inpainting,Textual and visual semantics,Hierarchical learning
AI 理解论文
溯源树
样例
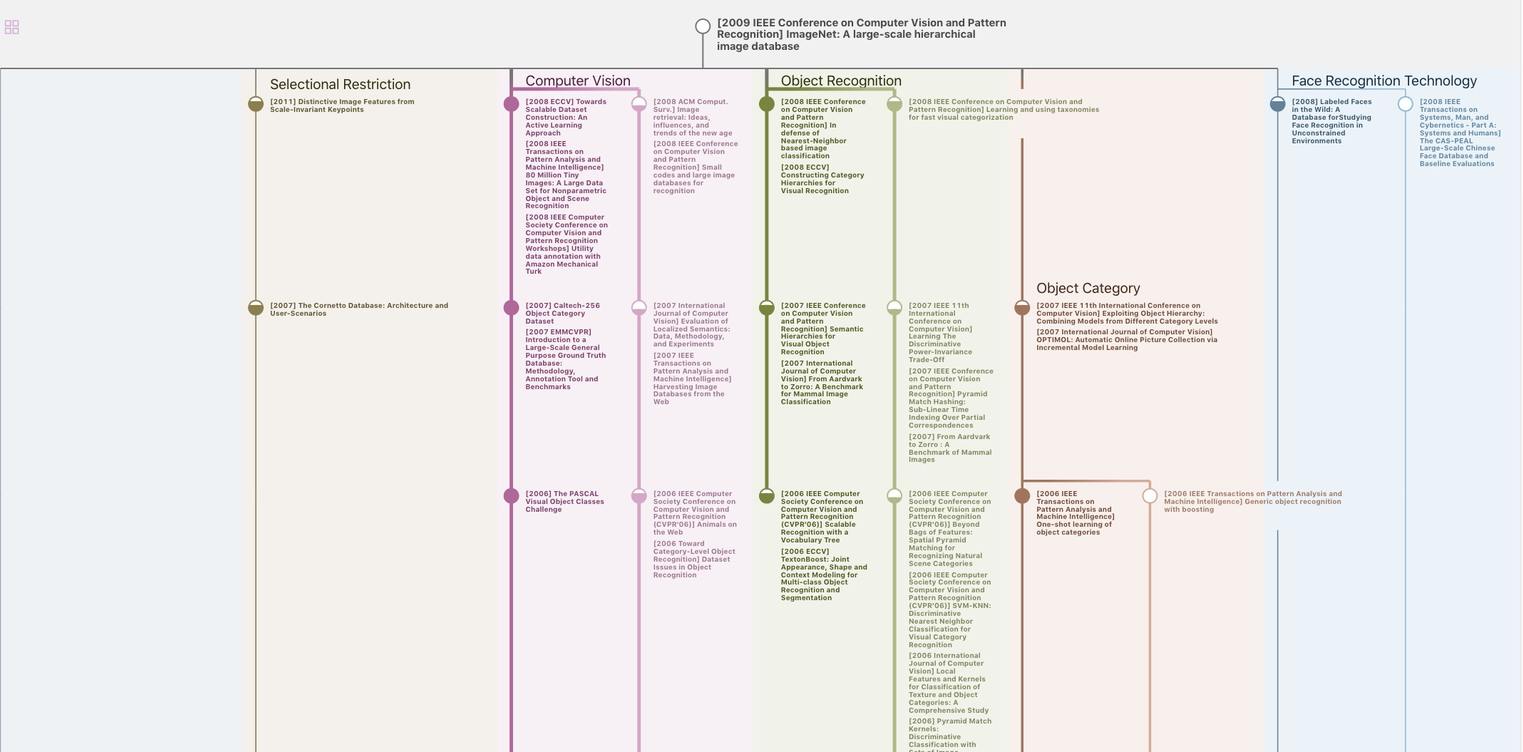
生成溯源树,研究论文发展脉络
Chat Paper
正在生成论文摘要