Multi-label feature selection by strongly relevant label gain and label mutual aid
Pattern Recognition(2024)
摘要
Multi-label feature selection, which addresses the challenge of high dimensionality in multi-label learning, has wide applicability in pattern recognition, machine learning, and related domains. Most existing studies on multi-label feature selection assume that all labels have the same importance with respect to features, however, they overlook the differences between labels and candidate features relative to selected features and the internal influence of the label space. To address this issue, we propose a novel method for multi-label feature selection that accounts for both the strongly relevant label gain and the label mutual aid. Firstly, we advance two new potential relationships between labels and candidate features relative to selected features, and the label discriminant function is introduced. Secondly, the mutual aid information between labels is presented to describe the internal correlation of the label space. Thirdly, the concept of strongly relevant label gain is defined based on the label discriminant function, which allows better exploration of positive correlation between features. Finally, the experimental results on sixteen multi-label benchmark datasets indicate that the proposed method outperforms other compared representative multi-label feature selection methods.
更多查看译文
关键词
Fuzzy rough set,Fuzzy conditional mutual information,Multi-label feature selection,Strongly relevant label gain,Label mutual aid
AI 理解论文
溯源树
样例
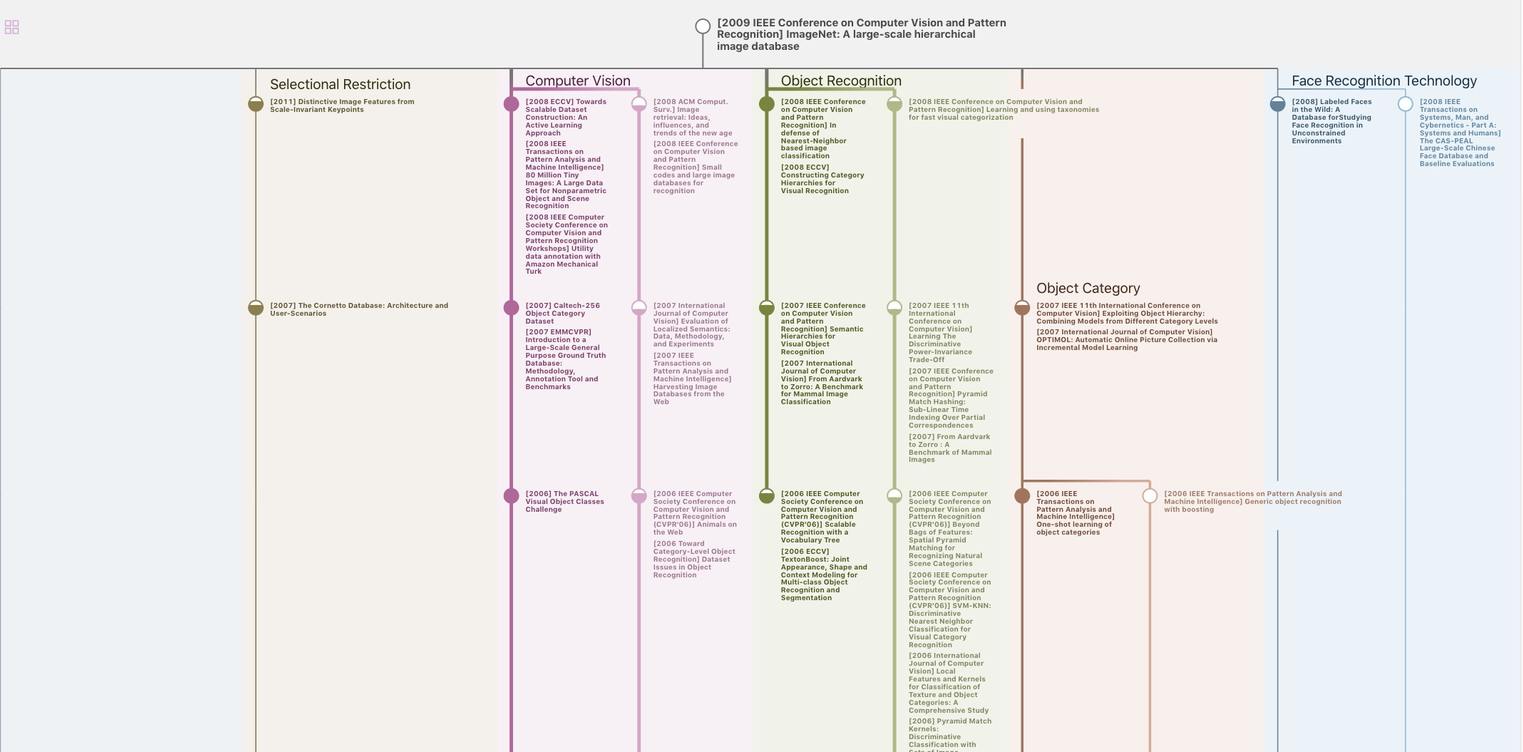
生成溯源树,研究论文发展脉络
Chat Paper
正在生成论文摘要