Combining physical-based model and machine learning to forecast chlorophyll-a concentration in freshwater lakes
SCIENCE OF THE TOTAL ENVIRONMENT(2024)
摘要
Increasing algal blooms in freshwater lakes have become a serious challenge facing the world. Short-term forecast of chlorophyll-a concentration (Chla) is essential for providing early warnings and taking action to mitigate the risks of algal blooms in freshwater lakes. At present, a variety of data-driven models and physicalbased models have been developed for Chla forecast, yet how to effectively combine multiple models for improving the forecast accuracy remains largely unknown. Here we developed an effective model by combining a physical-based model and machine learning algorithms (long short-term memory, LSTM; random forest, RF; support vector machine, SVM) to forecast the Chla in a freshwater lake, and a Bayesian model averaging (BMA) ensemble forecasting method was further proposed to improve the accuracy and reliability of the forecast results. We found that, with the increase of time steps of advance forecast from 1-day to 7-day, the forecast accuracy as measured by R2 of the machine learning algorithms is decreased from 0.95 to 0.68. The combination of physicalbased modeling with LSTM had great capability in short-term forecast of Chla, owing to the fact that the physicalbased model can provide high-frequency Chla data and LSTM is skilled at forecasting in the sequence. This is also evidenced by the weights in the BMA method. The proposed BMA short-term ensemble forecasting results had the robust performance when compared to each individual machine learning forecast model for the 7-day advance forecast, with the largest R2 (0.834) and the smallest RMSE (0.267 mu g/L). In particular, the uncertainty of a single machine learning model can be effectively reduced by the BMA method.
更多查看译文
关键词
Chlorophyll-a concentration,Algal blooms,Freshwater lake,Short-term ensemble forecast,Machine learning,Bayesian model averaging
AI 理解论文
溯源树
样例
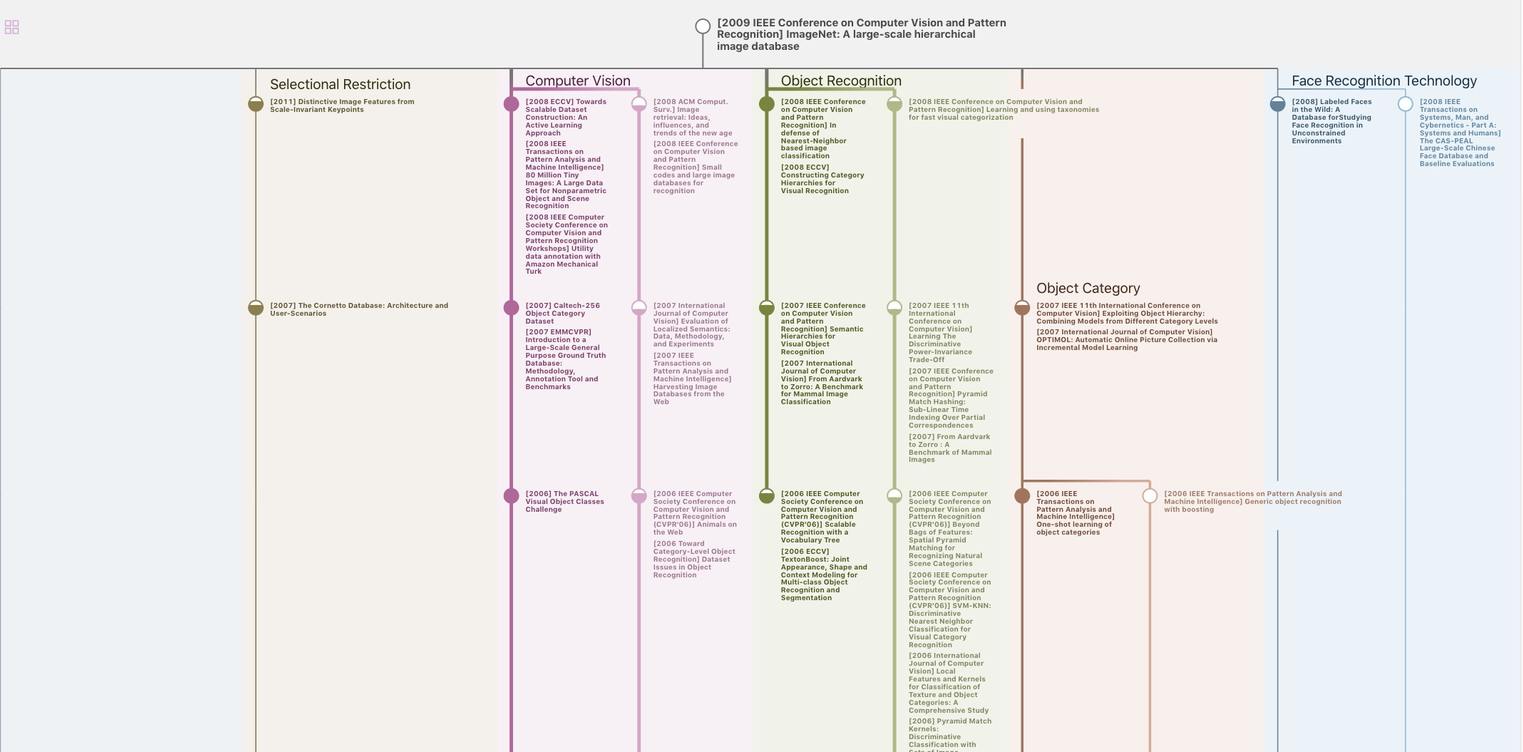
生成溯源树,研究论文发展脉络
Chat Paper
正在生成论文摘要