A data-driven methodology with a nonparametric reliability method for optimal condition-based maintenance strategies
RELIABILITY ENGINEERING & SYSTEM SAFETY(2024)
摘要
Optimizing condition-based maintenance (CBM) strategies based on machine learning (ML) methods as reinforcement learning (RL) have been receiving increasing attention due to their competencies. However, most existing research depends on simplifying assumptions about the deterioration process and aims to obtain only a threshold for preventive maintenance using the maintenance cost as RL's reward function. To tackle these limitations, this paper proposes a data-driven CBM optimization methodology that combines ML prediction model and RL method with a reliability-based method for remaining useful life (RUL) estimation. The prediction model learns the system's actual deterioration from historical deterioration data. The RL method integrates the prediction model with a customized reward function that minimizes the average maintenance cost. This reward function incorporates the maintenance cost and the system's RUL. The RUL estimation method utilizes the Nonparametric reliability method's Kaplan-Meier (KM) product limit. The CBM decision-making problem enables preventive and corrective replacements besides multi-level preventive repairs that restore the system's condition to a specific previous deterioration level. Contrary to the widely proposed threshold CBM strategies, the proposed methodology provides a strategy that maps each system state to the optimal maintenance action. A real case study is proposed to validate the methodology.
更多查看译文
关键词
Condition-based maintenance,Optimal strategy,Reinforcement learning,Deteriorating system,Remaining useful life
AI 理解论文
溯源树
样例
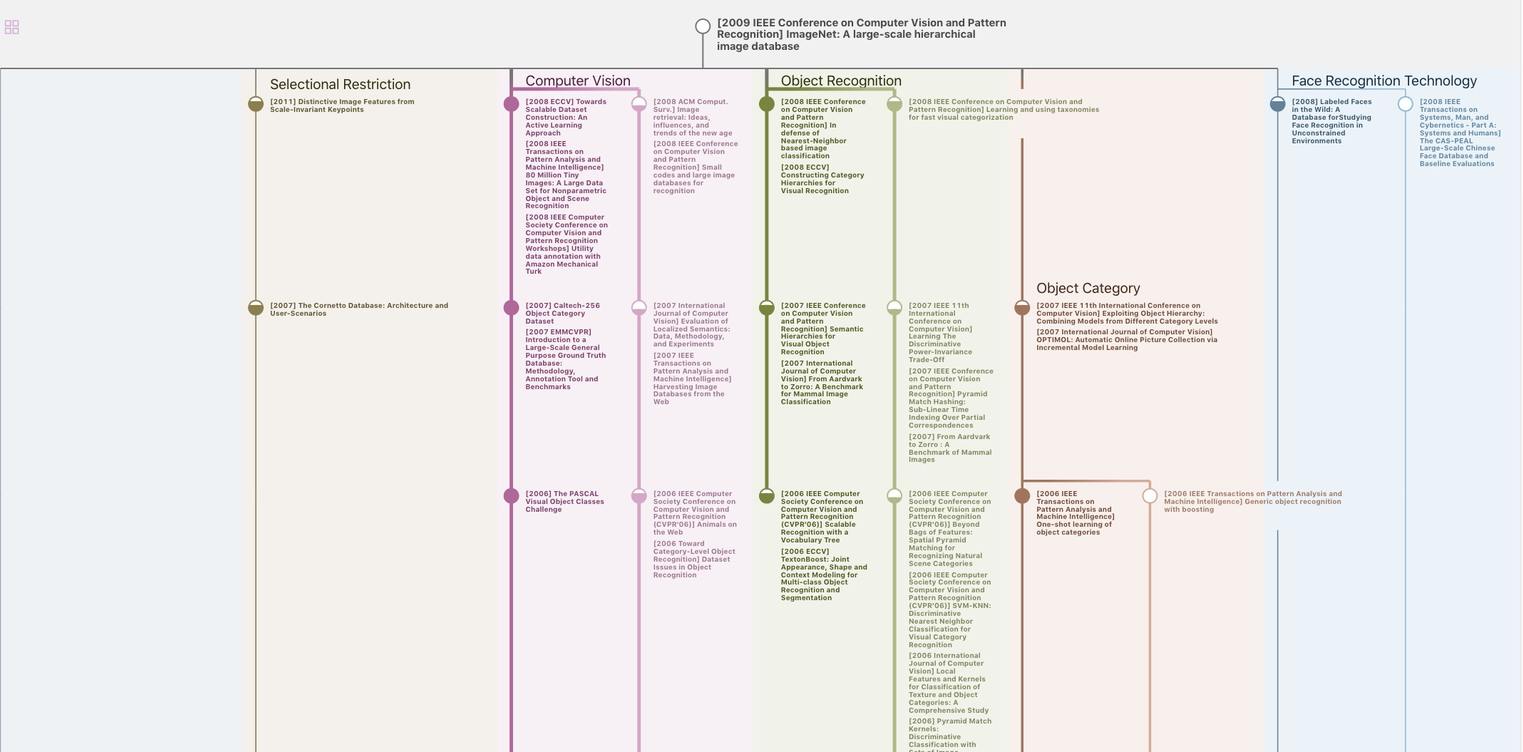
生成溯源树,研究论文发展脉络
Chat Paper
正在生成论文摘要