The Effect of Synthetic Training Data on the Performance of a Deep Learning Based Markerless Biomechanics System
bioRxiv (Cold Spring Harbor Laboratory)(2023)
摘要
As markerless motion capture technologies develop and mature, there is an increasing demand from the biomechanics community to provide kinematic data with the same level of accuracy as current gold standard methods. The purpose of this study was to evaluate how adding synthetic data to the training dataset of a deep learning based markerless biomechanics system impacts the accuracy of kinematic measurements during two functional movements. Synchronized video from multiple camera views was captured along with marker-based data from 9 subjects who performed 3 repetitions of countermovement jumps and squats. Including synthetic data to the training reduced lower limb error on average by 65.1% and 70.1% for the countermovement jump and squat movements, respectively. These results demonstrate the promising utility of supplementing the training of a deep learning markerless motion capture system with synthetic data.
### Competing Interest Statement
The authors have declared no competing interest.
更多查看译文
关键词
synthetic training data,deep learning
AI 理解论文
溯源树
样例
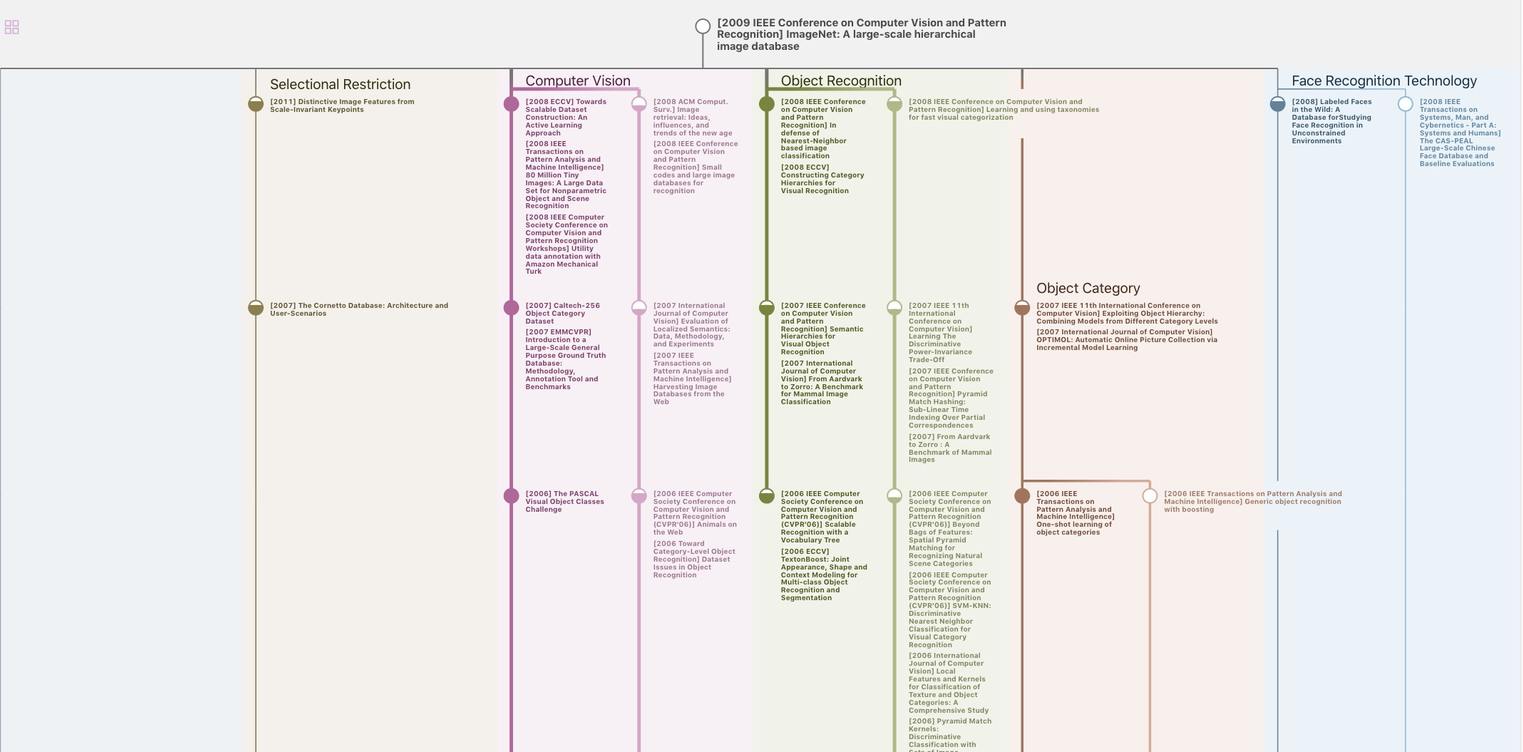
生成溯源树,研究论文发展脉络
Chat Paper
正在生成论文摘要