MIL-ViT: A multiple instance vision transformer for fundus image classification
JOURNAL OF VISUAL COMMUNICATION AND IMAGE REPRESENTATION(2023)
摘要
Despite the great success of deep learning approaches, retinal disease classification is still challenging as the early-stage pathological regions of retinal diseases may be extremely tiny and subtle, which are difficult for networks to detect. The feature representations learnt by deep learning models focusing more on the local view may lead to indiscriminative semantic-level representation. On the contrary, if they focus more on the global semantic-level, they may ignore the discerning subtle local pathological regions. To address this issue, in this paper, we propose a hybrid framework, combining the strong global semantic representation learning capability of the vision Transformer (ViT) and the excellent capacity of local representation extraction from the conventional multiple instance learning (MIL). Particularly, a multiple instance vision Transformer (MIL-ViT) is implemented, where the vanilla ViT branch and the MIL branch generate semantic probability distributions separately, and a bag consistency loss is proposed to minimize the difference between them. Moreover, a calibrated attention mechanism is developed to embed the instance representation into the bag representation in our MIL-ViT. To further improve the feature representation capability for fundus images, we pre-train the vanilla ViT on a large-scale fundus image database. The experimental results validate that the generalization capability of the model using our pre-trained weights for fundus disease diagnosis is better than the one using ImageNet pre-trained weights. Extensive experiments on four publicly available benchmarks demonstrate that our proposed MIL-ViT outperforms latest fundus image classification methods, including various deep learning models and deep MIL methods. All our source code and pre-trained models are publicly available at https://github.com/greentreeys/MIL-VT.
更多查看译文
关键词
Vision transformer,Multiple instance learning,Fundus image,Attention aggregation,Calibrated attention mechanism
AI 理解论文
溯源树
样例
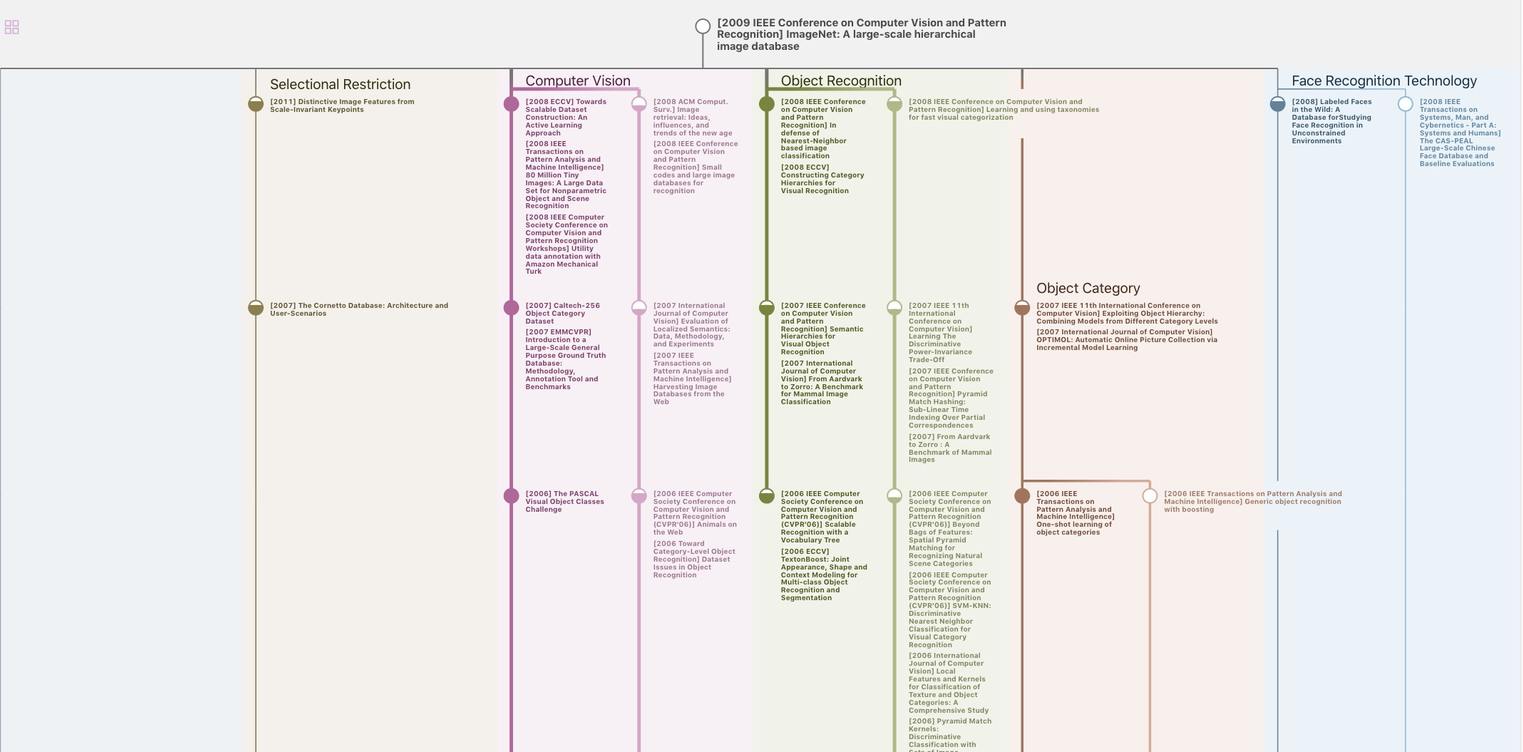
生成溯源树,研究论文发展脉络
Chat Paper
正在生成论文摘要