BiOM: A framework for multimodal multiobjective optimization
INFORMATION SCIENCES(2024)
摘要
Multimodal multiobjective evolutionary algorithms are expected to search for not only Pareto optimal front but also the corresponding multiple equivalent Pareto optimal sets. To this end, this paper designs a framework to ameliorate the performance of the existing multiobjective evolutionary algorithms (MOEAs) for solving multimodal multiobjective optimization problems (MMOPs). In this framework, an MMOP is transformed into a bi-objective optimization problem. The first objective is built by either decomposition-based method or indicator-based method in MOEAs to ensure the population convergence. A diversity indicator is another objective used to preserve the population diversity. Based on these two objectives, two diversity selection strategies are developed, which are responsible for balancing the diversity and the convergence in the decision and objective spaces, respectively, at the same time. According to the feedback information in the evolution, our framework adaptively selects one of them to pick out the promising individuals. Four variants based on our framework are built and are evaluated on 22 MMOPs benchmark functions. Six feature selection problems are also employed to test the performance of our framework. The experimental results confirm that our proposed framework performs well in all criteria.
更多查看译文
关键词
Multimodal multiobjective optimization,Multiobjective optimization,Feature selection,Pareto optimal set,Pareto front
AI 理解论文
溯源树
样例
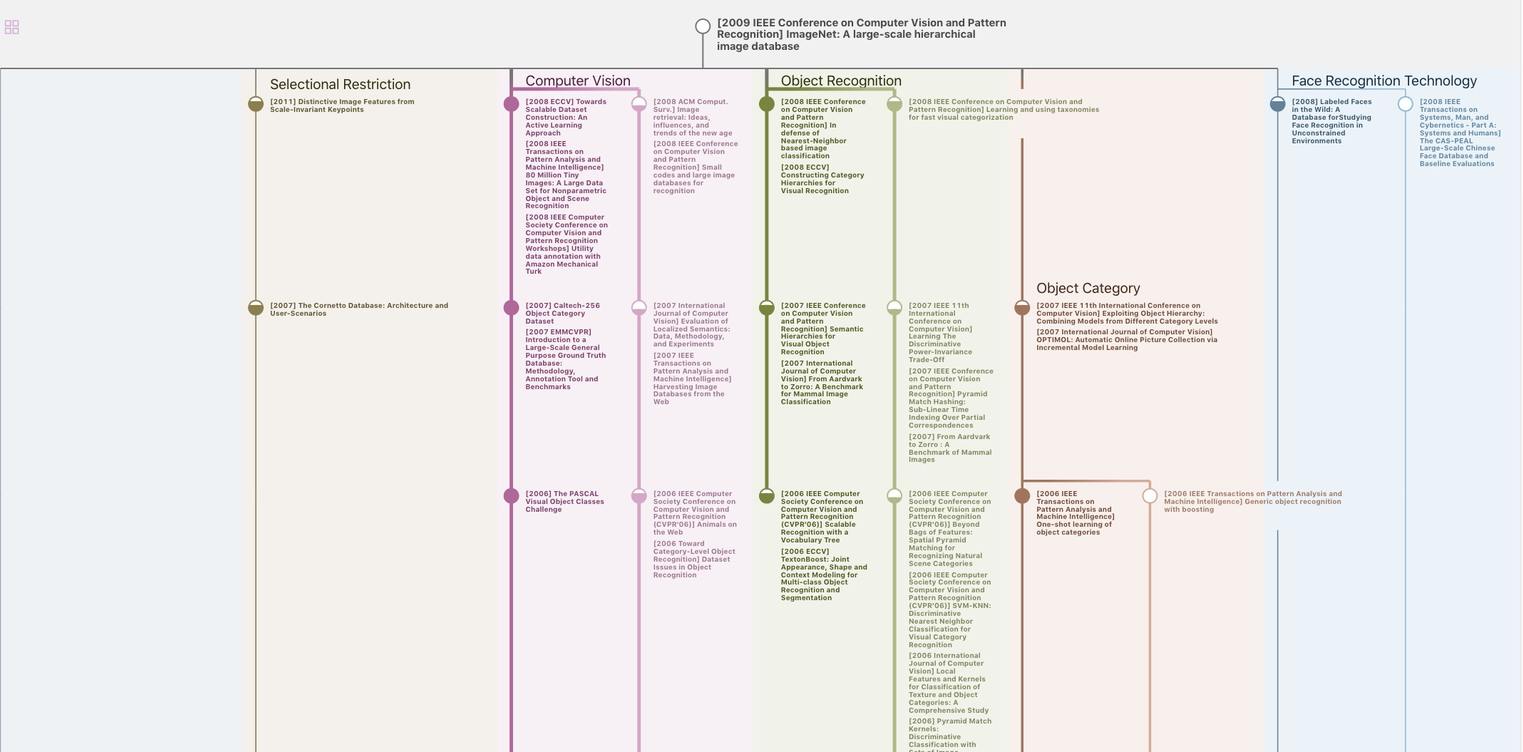
生成溯源树,研究论文发展脉络
Chat Paper
正在生成论文摘要