A hybrid approach combining data envelopment analysis and recurrent neural network for predicting the efficiency of research institutions
EXPERT SYSTEMS WITH APPLICATIONS(2024)
摘要
The traditional data envelopment analysis (DEA) method relies solely on current and historical performance and is unable to predict future efficiency. To overcome this limitation, we propose a novel prediction model called the input-output memory network (IOMN), which extends the Recurrent Neural Network (e.g., Long Short-Term Memory (LSTM) and Gate Recurrent Unit (GRU) to forecast the input and output variables in future periods. We then combined the IOMN with the DEA-Malmquist index to evaluate the efficiency of Decision-Making Units (DMUs) from the past to the future. To demonstrate the effectiveness of our proposed model, we conducted a case study of 15 research institutions in the Chinese Academy of Sciences (CAS) and compared its performance with those of linear regression (LR) and support vector regression (SVR) methods. The comparative results show that the IOMN significantly outperformed both the LR and SVR methods. Finally, we fed the past and predicted future data into the DEA-Malmquist index to examine the productivity efficiency trends in research institutions over time. Our results indicate that the annual mean of total factor productivity (TFP) continued to fluctuate at approximately 1 during the observation period. In the future, the geometric mean of TFP will decline slightly by 2.3%, the geometric mean of efficiency change (EC) will decline by 5%, and the geometric mean of technical change (TC) will increase by 2.9%.
更多查看译文
关键词
Recurrent Neural Network,LSTM,GRU,DEA,Malmquist index,Research institution
AI 理解论文
溯源树
样例
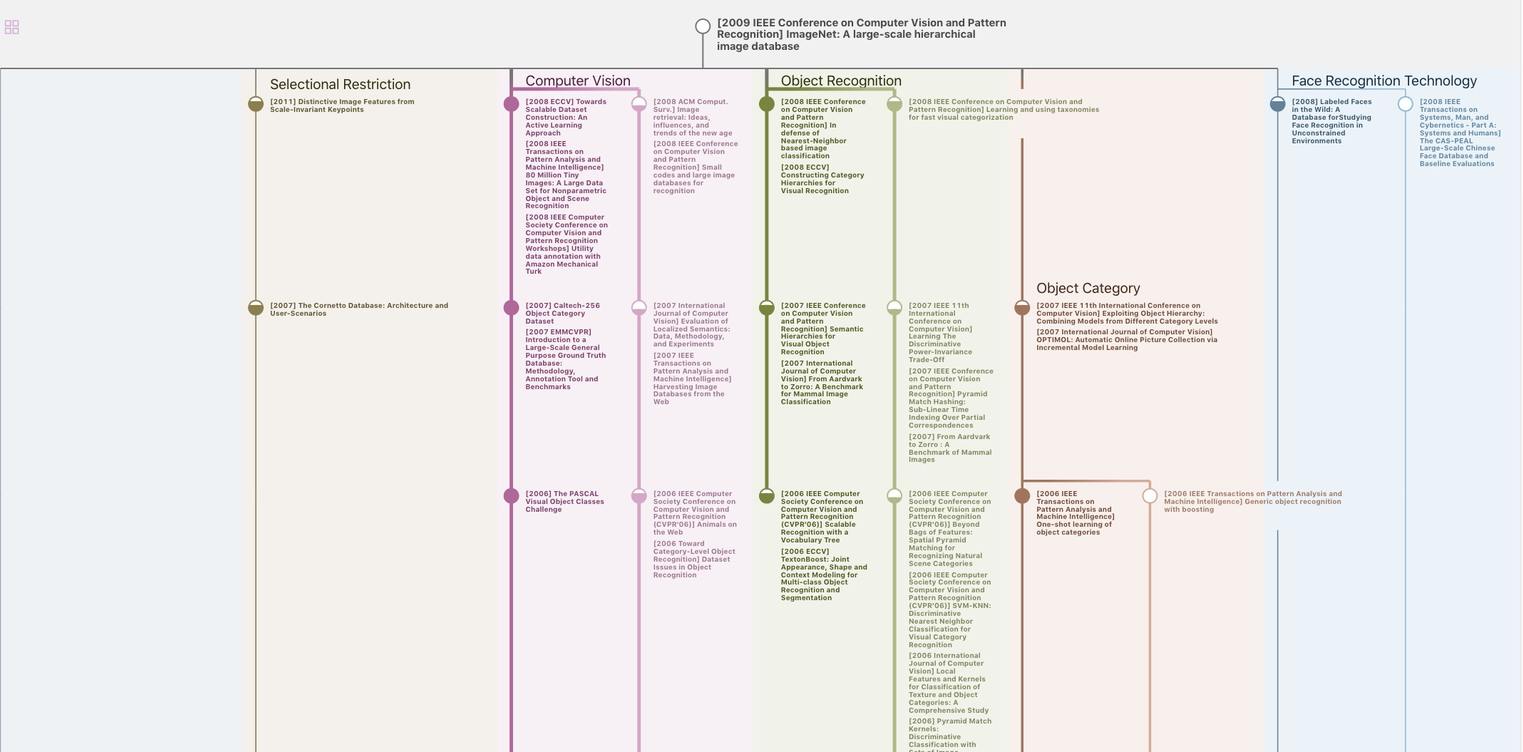
生成溯源树,研究论文发展脉络
Chat Paper
正在生成论文摘要