LiDAR attribute based point cloud labeling using CNNs with 3D convolution layers
Computers & Geosciences(2023)
摘要
The goal of this work is to study the adaptation of a semantic segmentation technique to use LiDAR point clouds in their original format as input. Until now, almost all the methods that have been proposed to apply deep learning models on point clouds keep their focus on generic point clouds, making use only of geometric or color information. The deep learning architecture proposed in this work acts as an alternative to classical convolution models. We use the EdgeConv operator, applied to 3D data, which uses the nearest neighbors to extract local features. We show the results of an experimentation that reveals the influence of additional LiDAR information channels on the performance of the neural network, proving that the usage of channels such as intensity can improve the average accuracy up to 20% when compared to the exclusive usage of geometry channels. Unlike other related works, this one is based in the study of semantic segmentation applied to outdoor environments.
更多查看译文
关键词
CNN,Edgeconv,Point cloud,LiDAR
AI 理解论文
溯源树
样例
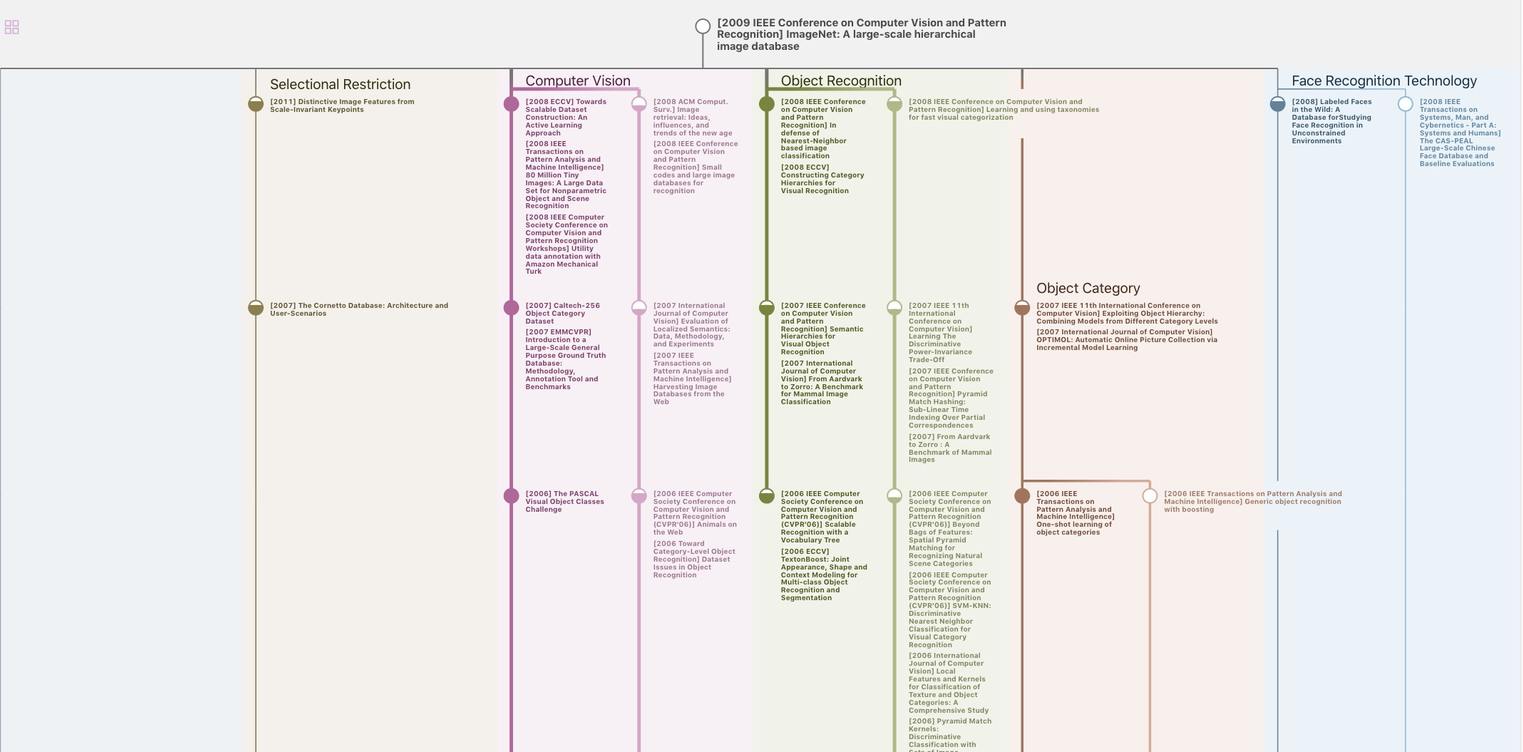
生成溯源树,研究论文发展脉络
Chat Paper
正在生成论文摘要