A deep local-temporal architecture with attention for lightweight human activity recognition
Applied Soft Computing(2023)
摘要
Human Activity Recognition (HAR) is an essential area of pervasive computing deployed in numerous fields. In order to seamlessly capture human activities, various inertial sensors embedded in wearable devices have been used to generate enormous amounts of signals, which are multidimensional time series of state changes. Therefore, the signals must be divided into windows for feature extraction. Deep learning (DL) methods have recently been used to automatically extract local and temporal features from signals obtained using wearable sensors. Likewise, multiple input deep learning architectures have been proposed to improve the quality of learned features in wearable sensor HAR. However, these architectures are often designed to extract local and temporal features on a single pipeline, which affects feature representation quality. Also, such models are always parameter-heavy due to the number of weights involved in the architecture. Since resources (CPU, battery, and memory) of end devices are limited, it is crucial to propose lightweight deep architectures for easy deployment of activity recognition models on end devices. To contribute, this paper presents a new deep parallel architecture named DLT, based on pipeline concatenation. Each pipeline consists of two sub-pipelines, where the first subpipeline learns local features in the current window using 1D-CNN, and the second sub-pipeline learns temporal features using Bi-LSTM and LSTMs before concatenating the feature maps and integrating channel attention. By doing this, the proposed DLT model fully harnessed the capabilities of CNN and RNN equally in capturing more discriminative features from wearable sensor signals while increasing responsiveness to essential features. Also, the size of the model is reduced by adding a lightweight module to the top of the architecture, thereby ensuring the proposed DLT architecture is lightweight. Experiments on two publicly available datasets showed that the proposed architecture achieved an accuracy of 98.52% on PAMAP2 and 97.90% on WISDM datasets, outperforming existing models with few model parameters.
更多查看译文
关键词
Wearable sensors,Local features,Temporal features,Lightweight,Deep learning
AI 理解论文
溯源树
样例
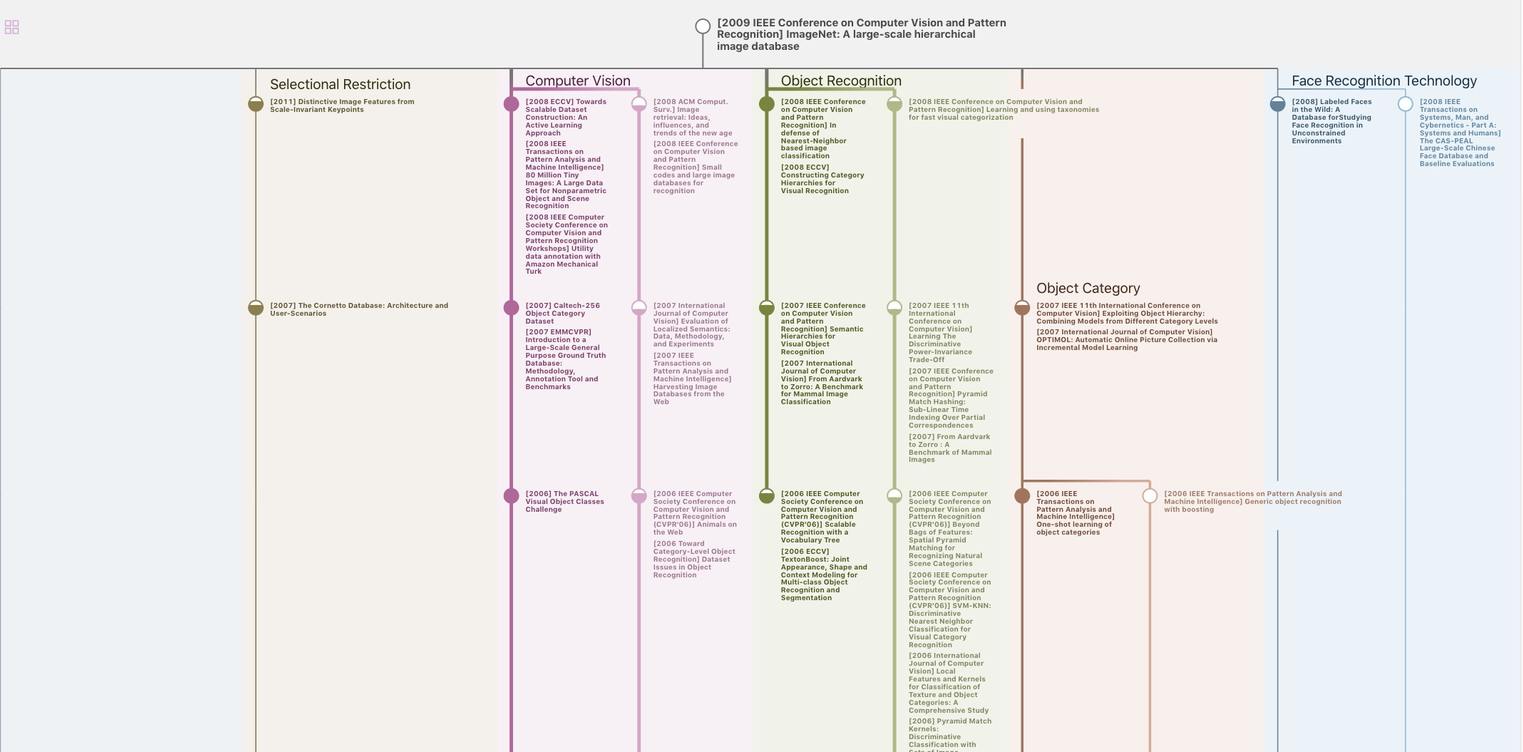
生成溯源树,研究论文发展脉络
Chat Paper
正在生成论文摘要