EDVAE: Disentangled latent factors models in counterfactual reasoning for individual treatment effects estimation
INFORMATION SCIENCES(2024)
摘要
Estimating individual treatment effect (ITE) from observational data is a crucial but challenging task. Disentangled representations have been used to separate proxy variables into confounding, instrumental, and adjustment variables. However, accurately performing counterfactual reasoning based on observational data to identify ITE remains an open problem. In this paper, we revisit the problem of ITE estimation from both data and model perspectives, shedding light on previously underexplored aspects. Specifically, we investigate the impact of imbalanced data on ITE estimation, highlighting the significance of Assumption-Compliant and Method -Simplicity in handling imbalanced data. From a model perspective, we revisit disentangled representation learning from an information-theoretic standpoint and provide theoretical evidence supporting the effectiveness of the Variational Auto-Encoder (VAE) framework for achieving disentanglement. Leveraging these insights, we propose the EDVAE, a data -driven model for disentangled latent factors. EDVAE comprises three scalable components: an oversampling layer for imbalanced data, a representation layer for disentangled latent factors, and an outcome prediction layer. Experimental results on synthetic and real-world datasets underscore the effectiveness of our proposed method, showcasing its potential for addressing the intricate problem of ITE estimation from observational data. The source code is available at https://github .com /MoranCoder95 /EDVAE.
更多查看译文
关键词
Individual treatment effect (ITE),Counterfactual reasoning,Disentangled representations,Imbalanced data,Variational auto-encoder (VAE)
AI 理解论文
溯源树
样例
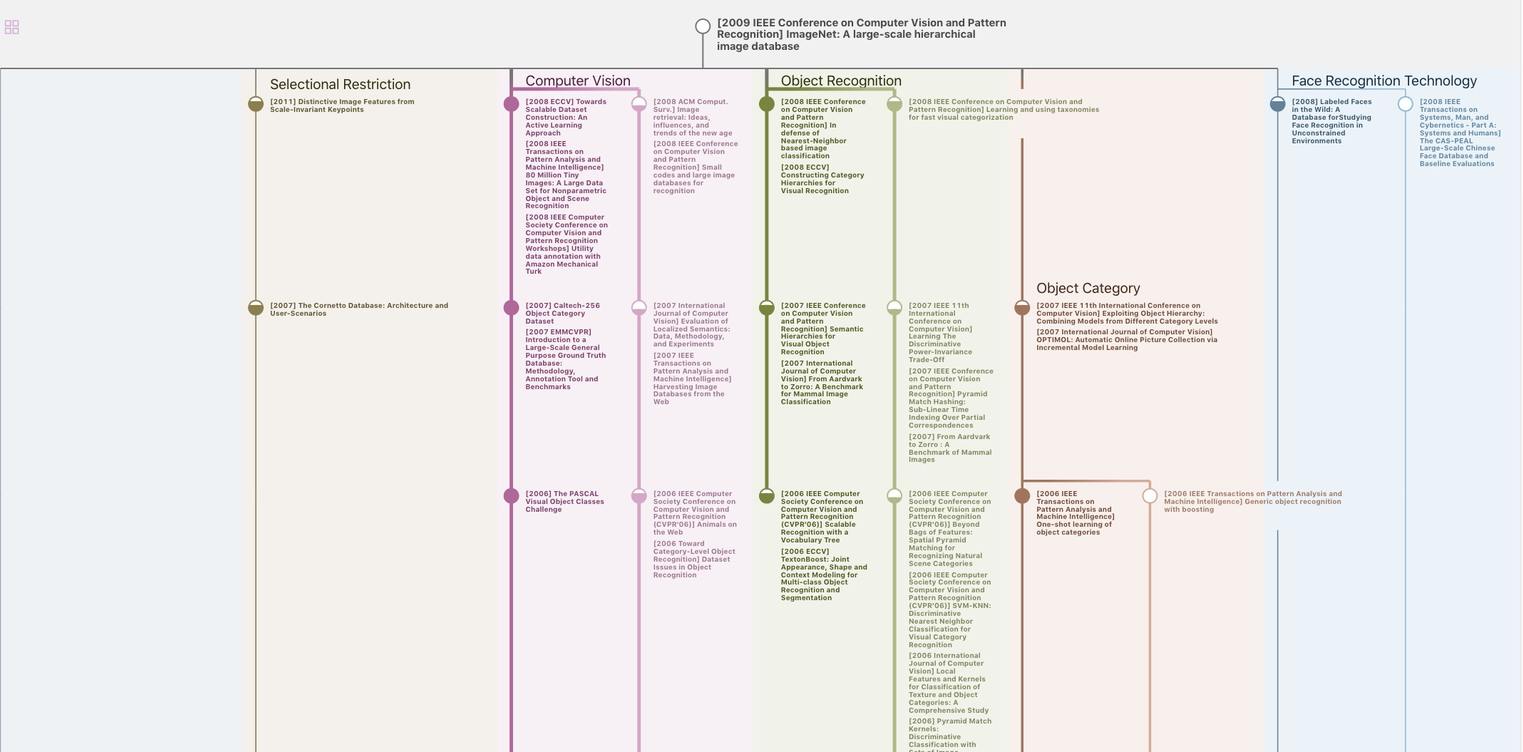
生成溯源树,研究论文发展脉络
Chat Paper
正在生成论文摘要